Energy-saving design of underground metro vertical alignment with deep reinforcement learning
TUNNELLING AND UNDERGROUND SPACE TECHNOLOGY(2024)
摘要
The design of metro vertical alignment greatly affects train traction energy consumption. This study develops a deep reinforcement learning (DRL) algorithm for an energy-saving design of underground metro vertical alignment and analyzes the influence of train traction energy consumption calculation factors (CFs) on energysaving design. First, a metro train operation simulation model is adopted using the approximate integral method, and the value range of CFs as input parameters is determined. Second, the 'agent', 'state', 'action', and 'reward' of the metro vertical alignment environment and the corresponding alignment constraints are determined. Third, a Dueling Double Deep Q Network (D3QN) is constructed to design the energy-saving metro vertical alignment. The proposed model is applied to a real-world metro case study, and the influence of CFs (design speed, limiting gradient in reverse slopes, and passenger capacity) on energy-saving design is analyzed. Finally, compared to manual design, the D3QN-based design outperforms GA-based design and DQN-based design, and demonstrates a remarkable energy savings of 6.17%.
更多查看译文
关键词
Vertical alignment design,Train traction energy consumption,Deep reinforcement learning,Energy-saving metro
AI 理解论文
溯源树
样例
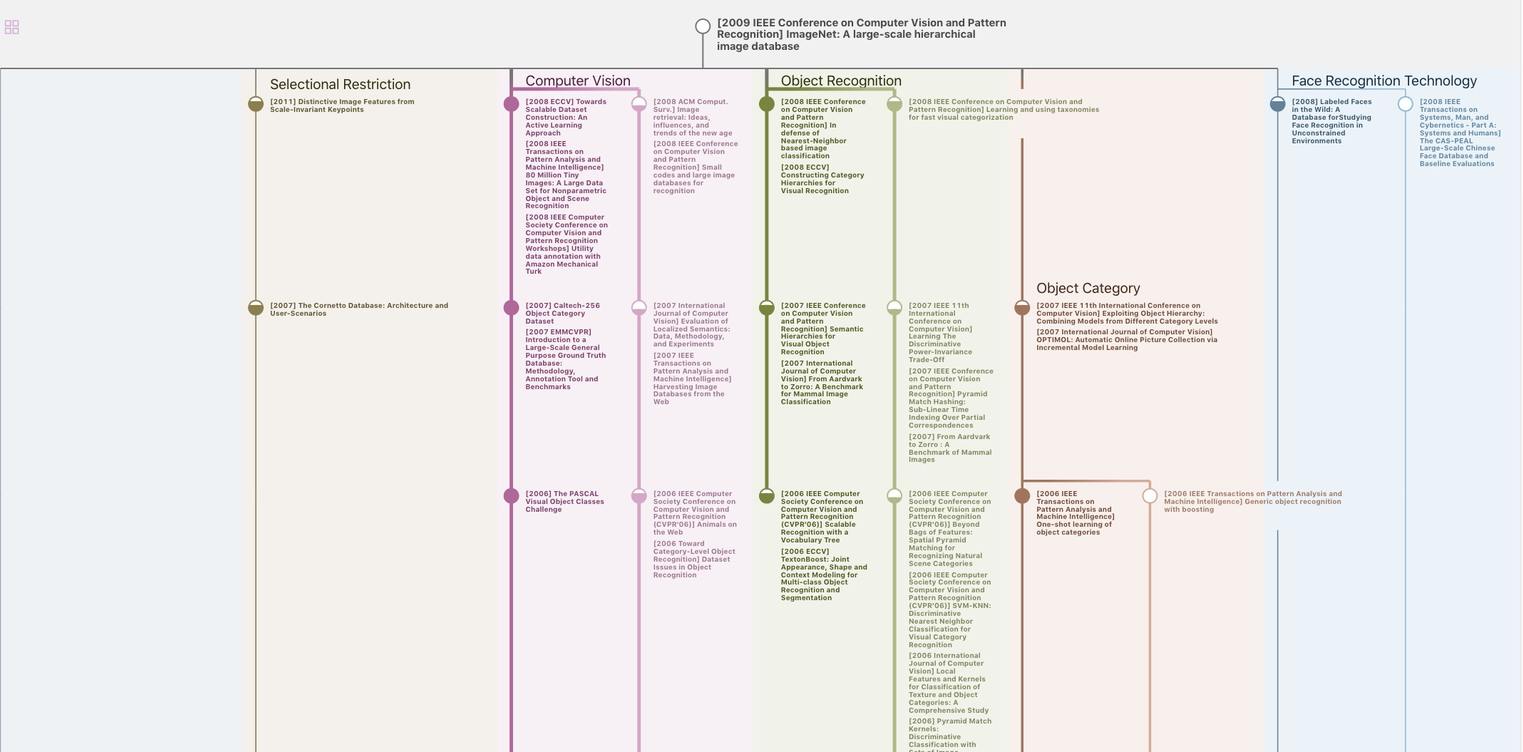
生成溯源树,研究论文发展脉络
Chat Paper
正在生成论文摘要