Energy Management of a Plug-in Hybrid Electric Vehicle Using Bayesian Optimization and Soft Actor-Critic Algorithm
IEEE Transactions on Transportation Electrification(2024)
摘要
The emerging deep reinforcement learning (DRL) algorithms are promising for energy management of plug-in hybrid electric vehicles (PHEVs), but most existing DRL methods cannot meet the requirement for learning robustness and data independence, which are essential to mission-critical systems like PHEVs. By incorporating Bayesian Optimization (BO) with the Soft Actor-Critic (SAC) algorithm, this paper proposes a new energy management strategy (EMS) for HEVs, namely, BO-SAC EMS. The present work contributes solutions to address the above-mentioned two challenges in DRL-based EMS: 1) through BO-based hyper-parameter tuning, the brittle convergence characteristics and robustness of the SAC algorithm have been significantly improved; and 2) by introducing a state-action-reward (SAR) co-design scheme for the SAC algorithm, the dependence on real-world data has been considerably reduced, thus improving training efficiency. Using the original SAC (Origin-SAC) method and dynamic programming (DP) results as the baseline, comparison studies are conducted under ten driving cycles. By estimating the maximum energy consumption per 100 kilometres based on the Six Sigma theory, BO-SAC is shown more robust by saving more than 3% energy in the worst case.
更多查看译文
关键词
Energy management,Hybrid electric vehicles,Soft actor-critic,Bayesian optimization,Robust optimization
AI 理解论文
溯源树
样例
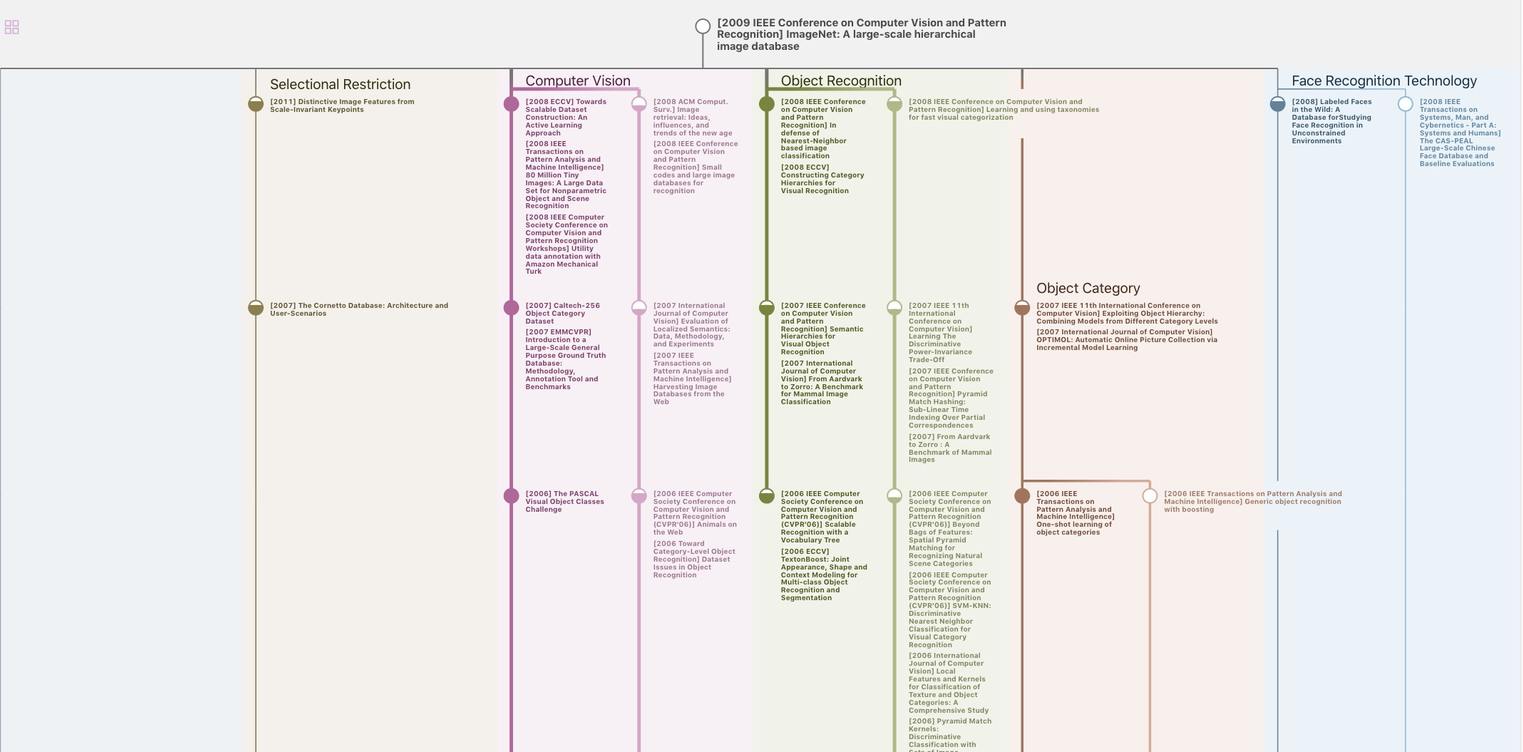
生成溯源树,研究论文发展脉络
Chat Paper
正在生成论文摘要