C2ANet: Cross-Scale and Cross-Modality Aggregation Network for Scene Depth Super-Resolution
IEEE TRANSACTIONS ON MULTIMEDIA(2024)
摘要
Existing depth super-resolution (DSR) methods typically utilize an additional high-resolution (HR) color image of the same scene as assistance to recover the low-resolution (LR) depth map. Although these color-guided methods have achieved impressive progress, they easily face with color image under-utilization and misutilization issues. In this article, we deeply investigate the above problems and further propose a novel DSR framework to alleviate them. Specifically, we propose a Cross-scale and Cross-modality Aggregation Network (C(2)ANet) to learn abundant and accurate complementarity from color images to help recover the degraded depth map. Our C-2 ANet can simultaneously extract multi-scale representations from color images with parallel network hierarchies, and effectively aggregate cross-scale and cross-modality contexts to boost HR representations in each hierarchy. Then, to appropriately use the guided color image, we further design a Feature Aggregation Module ( FAM ) to adaptively select and fuse task-relevant features, which consists of (1) a feature alignment block to learn transformation offsets and align upsampled features with targeted HR features, and (2) a feature fusion block based on cross-attention mechanism to maintain strong structural context and suppress texture distraction. Experimental results on synthetic and real-world benchmark datasets demonstrate the superiority of our proposed method in comparison with other state-of-the-art DSR methods.
更多查看译文
关键词
Color,Image color analysis,Task analysis,Feature extraction,Image reconstruction,Spatial resolution,Aggregates,Alignment,cross-attention,cross-modality,cross-scale,depth super-resolution
AI 理解论文
溯源树
样例
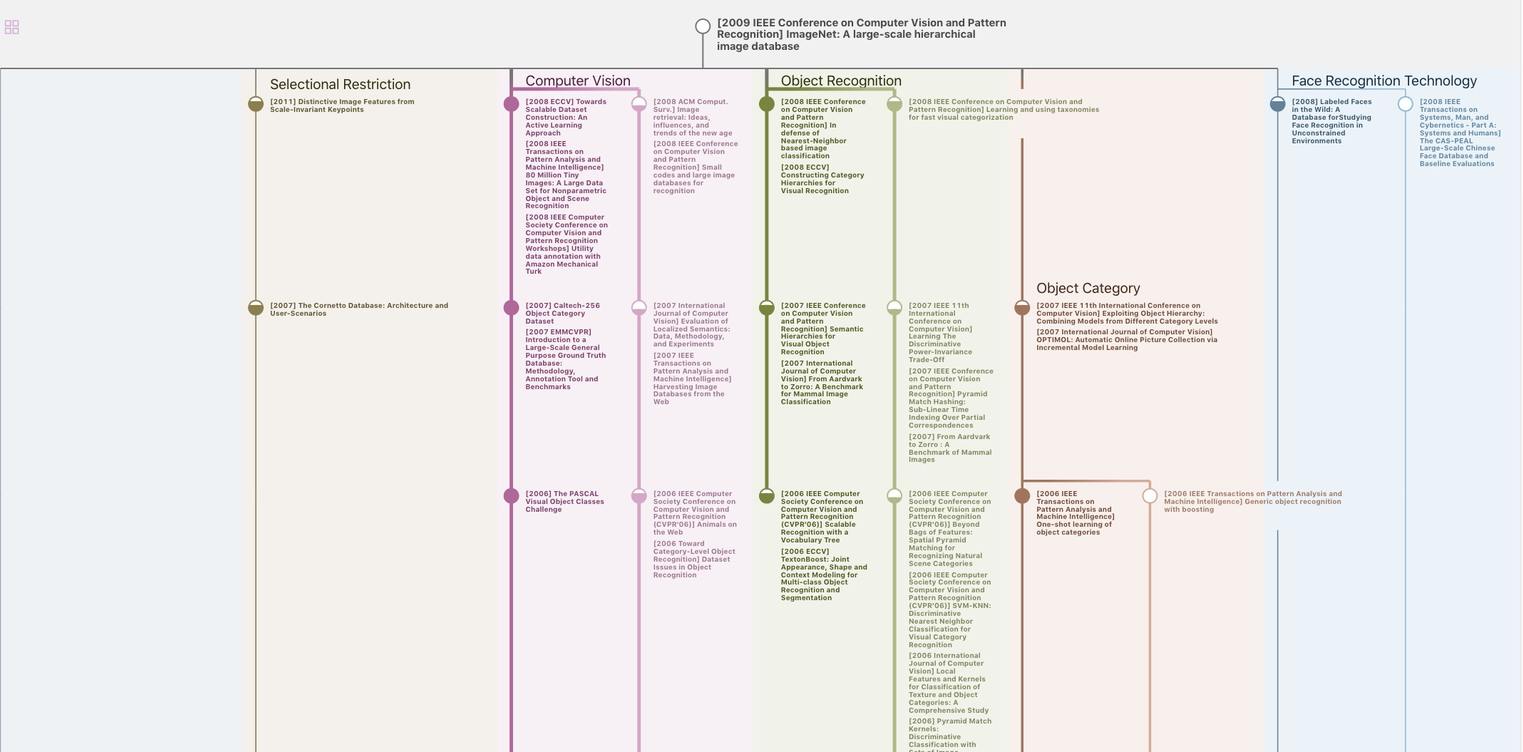
生成溯源树,研究论文发展脉络
Chat Paper
正在生成论文摘要