Reducing Oscillations for Obstacle Avoidance in a Dense Environment Using Deep Reinforcement Learning and Time-Derivative of an Artificial Potential Field
DRONES(2024)
摘要
Obstacle avoidance plays a crucial role in ensuring the safe path planning of quadrotor unmanned aerial vehicles (QUAVs). In this study, we propose a hierarchical framework for obstacle avoidance, which combines the use of artificial potential field (APF) and deep reinforcement learning (DRL) for training low-level motion controllers. Unlike traditional potential field methods, our approach modifies the state information received by the motion controllers using the outputs of the APF path planner. Specifically, the assumed target position is pushed away from obstacles, resulting in adjustments to the perceived position errors. Additionally, we address path oscillations by incorporating the target's velocity information, which is calculated based on the time-derivative of the repulsive force. Experimental results have validated the effectiveness of our proposed framework in avoiding collisions with obstacles and reducing oscillations.
更多查看译文
关键词
quadrotor unmanned aerial vehicle (QUAV),obstacle avoidance,artificial potential field (APF),deep reinforcement learning (DRL),oscillations
AI 理解论文
溯源树
样例
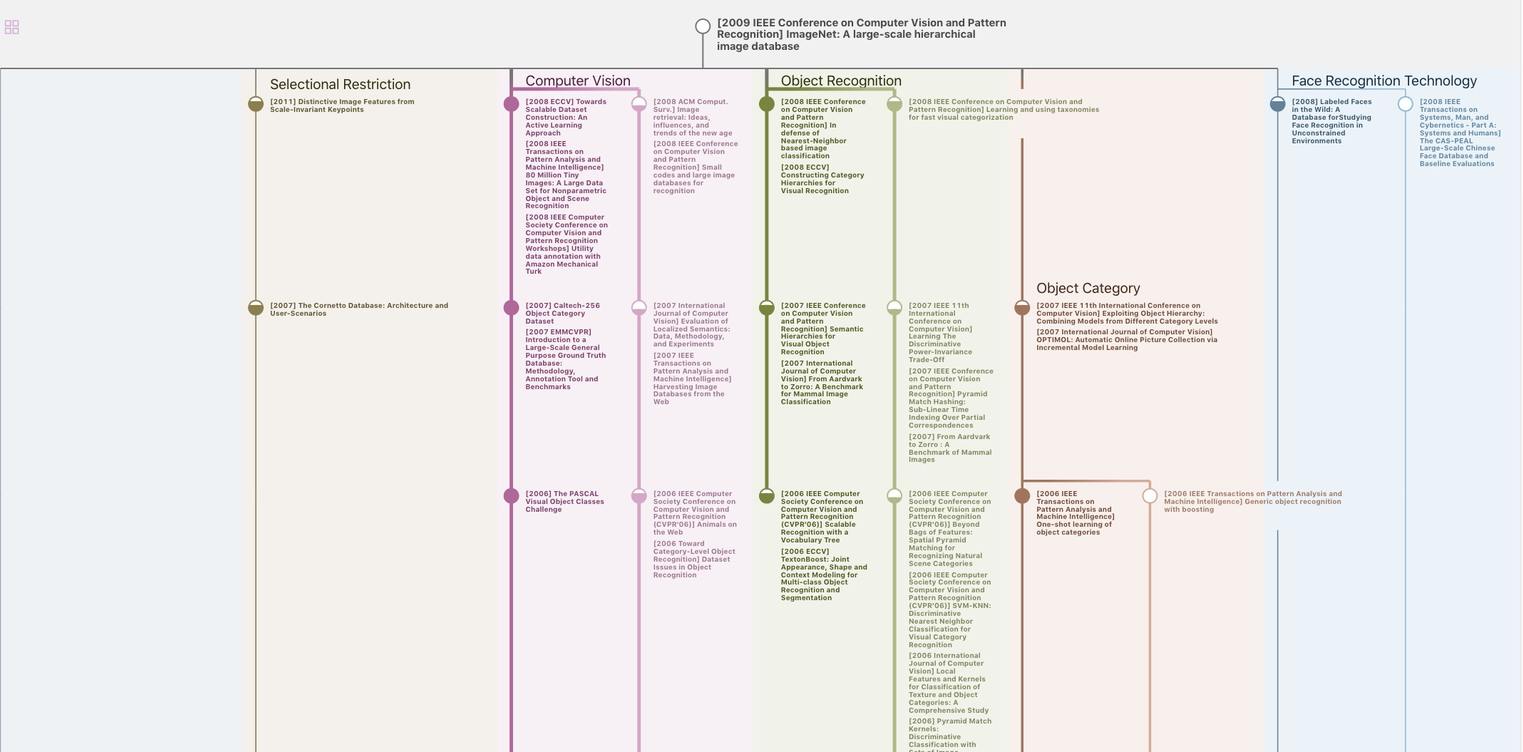
生成溯源树,研究论文发展脉络
Chat Paper
正在生成论文摘要