Modeling Crowd Data and Spatial Connectivity as Graphs for Crowd Flow Forecasting in Public Urban Space
COMPUTING IN CIVIL ENGINEERING 2023-RESILIENCE, SAFETY, AND SUSTAINABILITY(2024)
摘要
Predicting crowd flow patterns in a physical space can be useful for infrastructure management and safety planning. A simple representation of individuals in Euclidean space is insufficient for representing people's spatial distribution and movements over time. This paper describes a spatiotemporal graph formulation, namely crowd mobility graphs (CMGraphs), to represent the spatiotemporal data. The CMGraphs model employs dynamic node features that store temporal crowd flow information, while the time-invariant edges represent spatial connectivity of locations of interests in the surrounding space. The spatiotemporal formulation using the CMGraphs allows for crowd flow prediction. Specifically, graph neural network is used to aggregate neighborhood nodal information on CMGraphs to capture spatial connectivity. Subsequently, recurrent neural network is employed to generate future sequences of crowd flow. An experiment is conducted using a publicly available video dataset at a train station to demonstrate the effectiveness of the proposed CMGraph formulation for crowd flow forecasting.
更多查看译文
AI 理解论文
溯源树
样例
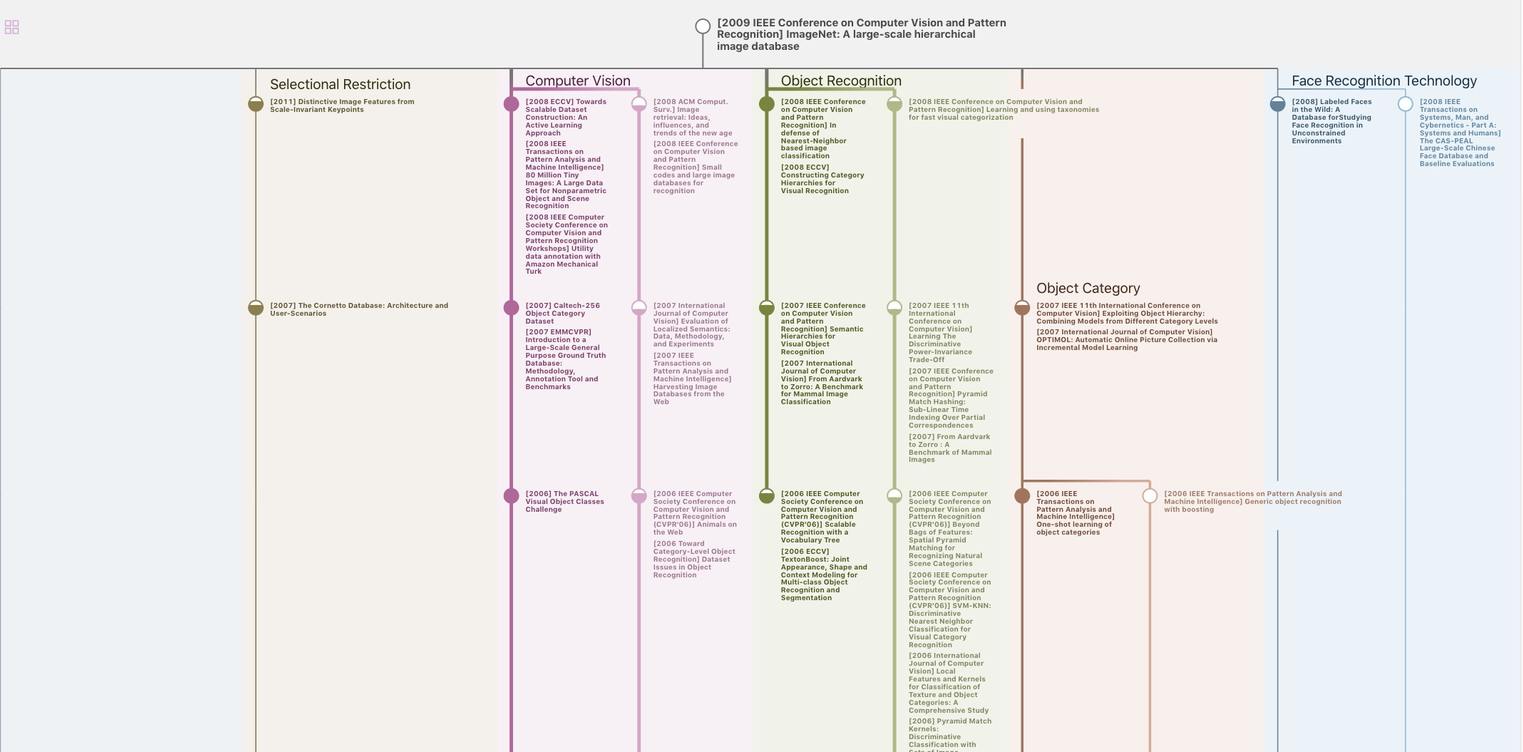
生成溯源树,研究论文发展脉络
Chat Paper
正在生成论文摘要