Towards ultrafast quantitative phase imaging via differentiable microscopy [Invited]
BIOMEDICAL OPTICS EXPRESS(2024)
摘要
With applications ranging from metabolomics to histopathology, quantitative phase microscopy (QPM) is a powerful label -free imaging modality. Despite significant advances in fast multiplexed imaging sensors and deep -learning -based inverse solvers, the throughput of QPM is currently limited by the pixel -rate of the image sensors. Complementarily, to improve throughput further, here we propose to acquire images in a compressed form so that more information can be transferred beyond the existing hardware bottleneck of the image sensor. To this end, we present a numerical simulation of a learnable optical compression -decompression framework that learns content -specific features. The proposed differentiable quantitative phase microscopy ( partial differential -QPM) first uses learnable optical processors as image compressors. The intensity representations produced by these optical processors are then captured by the imaging sensor. Finally, a reconstruction network running on a computer decompresses the QPM images post aquisition. In numerical experiments, the proposed system achieves compression of x 64 while maintaining the SSIM of -0.90 and PSNR of -30 dB on cells. The results demonstrated by our experiments open up a new pathway to QPM systems that may provide unprecedented throughput improvements.
更多查看译文
AI 理解论文
溯源树
样例
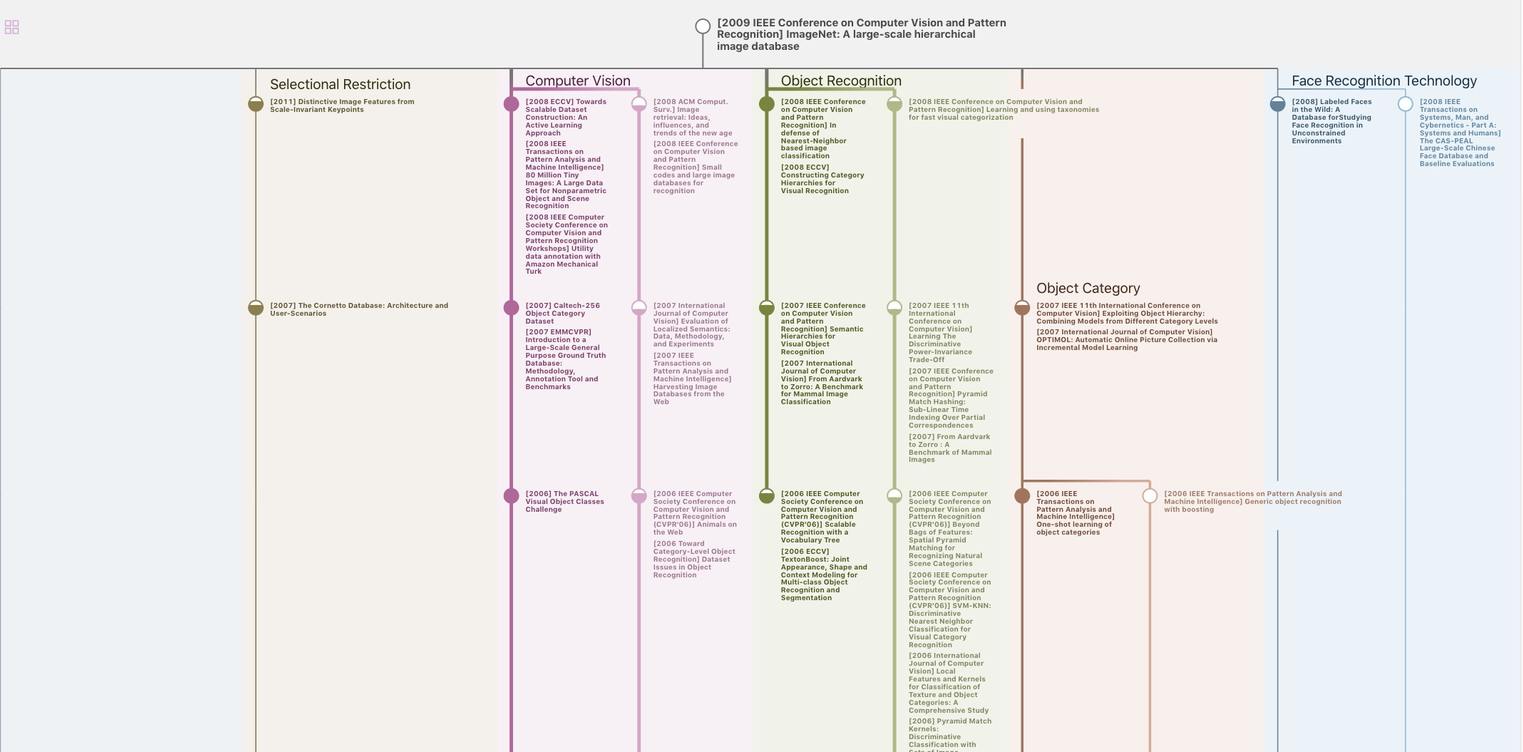
生成溯源树,研究论文发展脉络
Chat Paper
正在生成论文摘要