Stable Variable Selection Method with Shrinkage Regression Applied to the Selection of Genetic Variants Associated with Alzheimer's Disease
APPLIED SCIENCES-BASEL(2024)
摘要
In this work, we aimed to establish a stable and accurate procedure with which to perform feature selection in datasets with a much higher number of predictors than individuals, as in genome-wide association studies. Due to the instability of feature selection where many potential predictors are measured, a variable selection procedure is proposed that combines several replications of shrinkage regression models. A weighted formulation is used to define the final predictors. The procedure is applied for the investigation of single nucleotide polymorphism (SNP) predictors associated with Alzheimer's disease in the Alzheimer's Disease Neuroimaging Initiative (ADNI) dataset. Furthermore, the two following data scenarios are investigated: one that solely considers the set of SNPs, and another with the covariates of age, sex, educational level, and epsilon 4 allele of the Apolipoprotein E (APOE4) genotype. The SNP rs2075650 and the APOE4 genotype are provided as risk factors for Alzheimer's disease, which is in line with the literature, and another four new SNPs are indicated, thus cultivating new hypotheses for in vivo analyses. These experiments demonstrate the potential of the new method for stable feature selection.
更多查看译文
关键词
penalized regression,Akaike's information criterion,high-dimensional data,stability,overall weighted coefficients,Alzheimer's disease,SNP
AI 理解论文
溯源树
样例
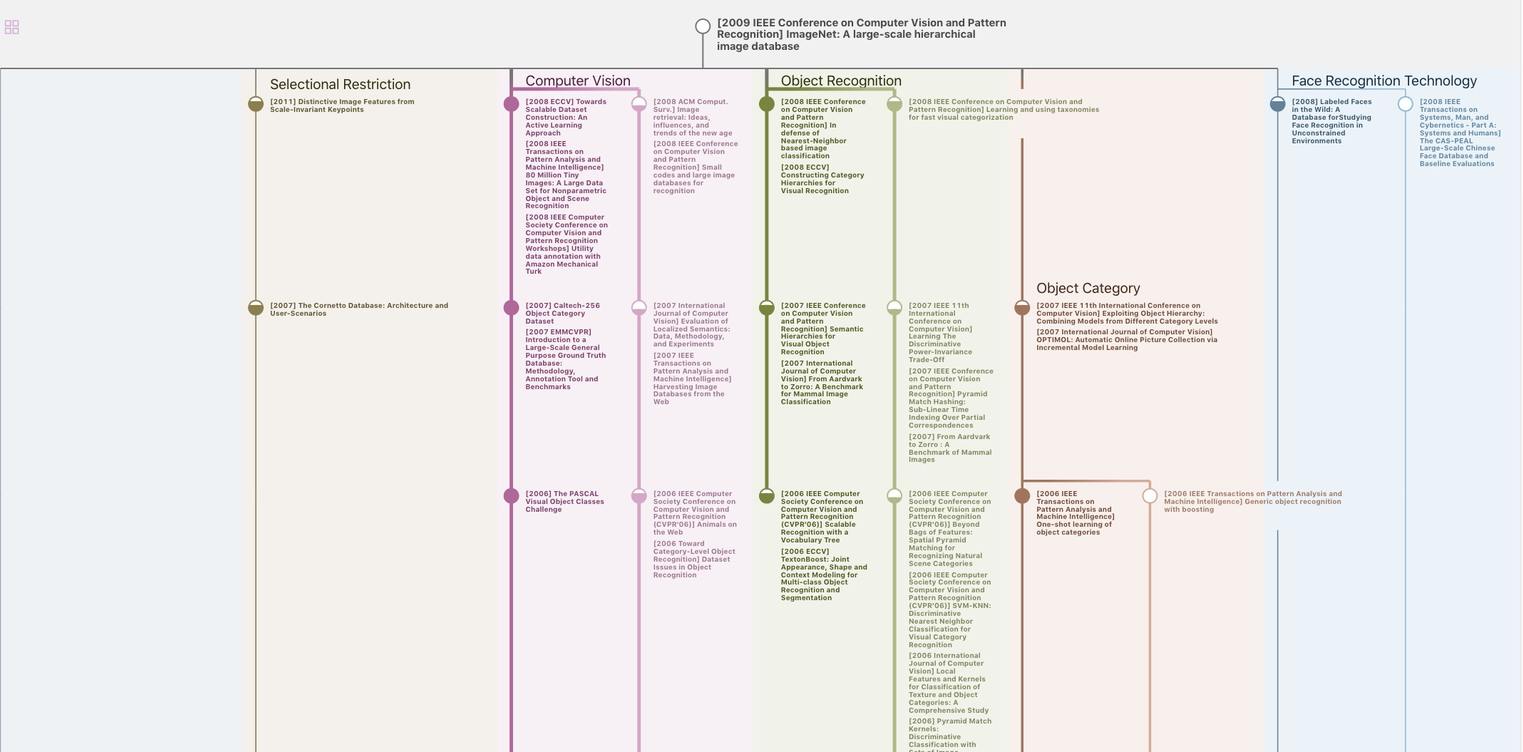
生成溯源树,研究论文发展脉络
Chat Paper
正在生成论文摘要