An interpretable lightweight deep network with lp(0 < p < 1) model-driven for single image super-resolution
NEUROCOMPUTING(2024)
摘要
In order to address the expensive computation cost of deep networks, some Single Image Super -Resolution (SISR) methods tried to design the lightweight networks by means of recursion or expert prior. However, they discuss the theoretical interpretation for the network's design less. To address this issue, we propose a novel method for constructing an interpretable lightweight deep network for SISR by fusing the idea of model -driven and data -driven. That is, we give a theoretical interpretation for the lightweight network's design from the optimization model of image degeneration. Considering that l(p)(0 < p < 1) -norm is sparser than the l(1)-norm and can describe the noises of image degeneration better, our proposed SISR method firstly deduces an iteration algorithm from the l(p)(0 < p < 1) degeneration model. Then according to this theoretical deduction, an effective deep network is designed. Since our proposed deep network is designed according to the iteration algorithm, our network can not only realize the lightweight structure because of the weight sharing decided by the iterative principle, but also show a theoretical interpretation for designing the deep network. Extensive experimental results illustrate that our proposed method is superior to some related popular SISR methods with the lightweight structure.
更多查看译文
关键词
Single image super-resolution,Deep learning,Interpretable,Lightweight,Expert prior
AI 理解论文
溯源树
样例
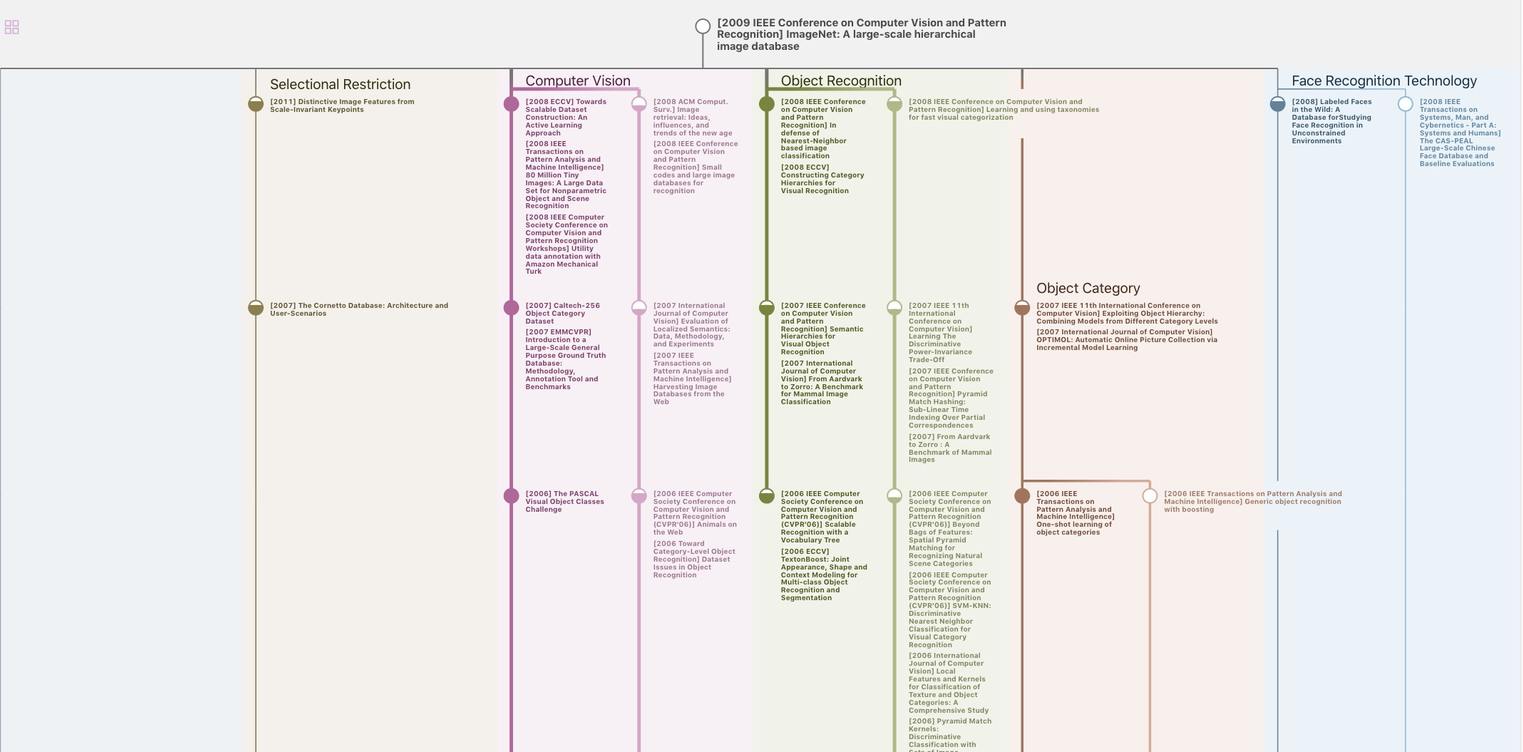
生成溯源树,研究论文发展脉络
Chat Paper
正在生成论文摘要