A degressive quantum convolutional neuralnetwork for quantum state classificationand code recognition
ISCIENCE(2024)
摘要
With the rapid development of quantum computing, a variety of quantum convolutional neural networks(QCNNs) are proposed. However, only1=2n2features of ann-qubits input are transferred to the next layerin a quantum pooling layer, which results in the accuracy reduction. To solve this problem, a QCNN with adegressive circuit is proposed. In order to enhance the ability of extracting global features, we remove theparameters sharing strategy in the quantum convolutional layer and design a quantum convolutionalkernel with global eyesight. In addition, to prevent a sharp feature reduction, a degressive parameterizedquantum circuit is adopted to construct the pooling layer. Then theZ-basis measurement is only per-formed on the first qubit to control the operations on other qubits. Compared with the state-of-the-artQCNN, i.e., hybrid quantum-classical convolutional neural network, the accuracy of our model increasedby 0.9%, 1%, and 3%, respectively, in three tasks: quantum state classification, binary code recognition,and quaternary code recognition.
更多查看译文
AI 理解论文
溯源树
样例
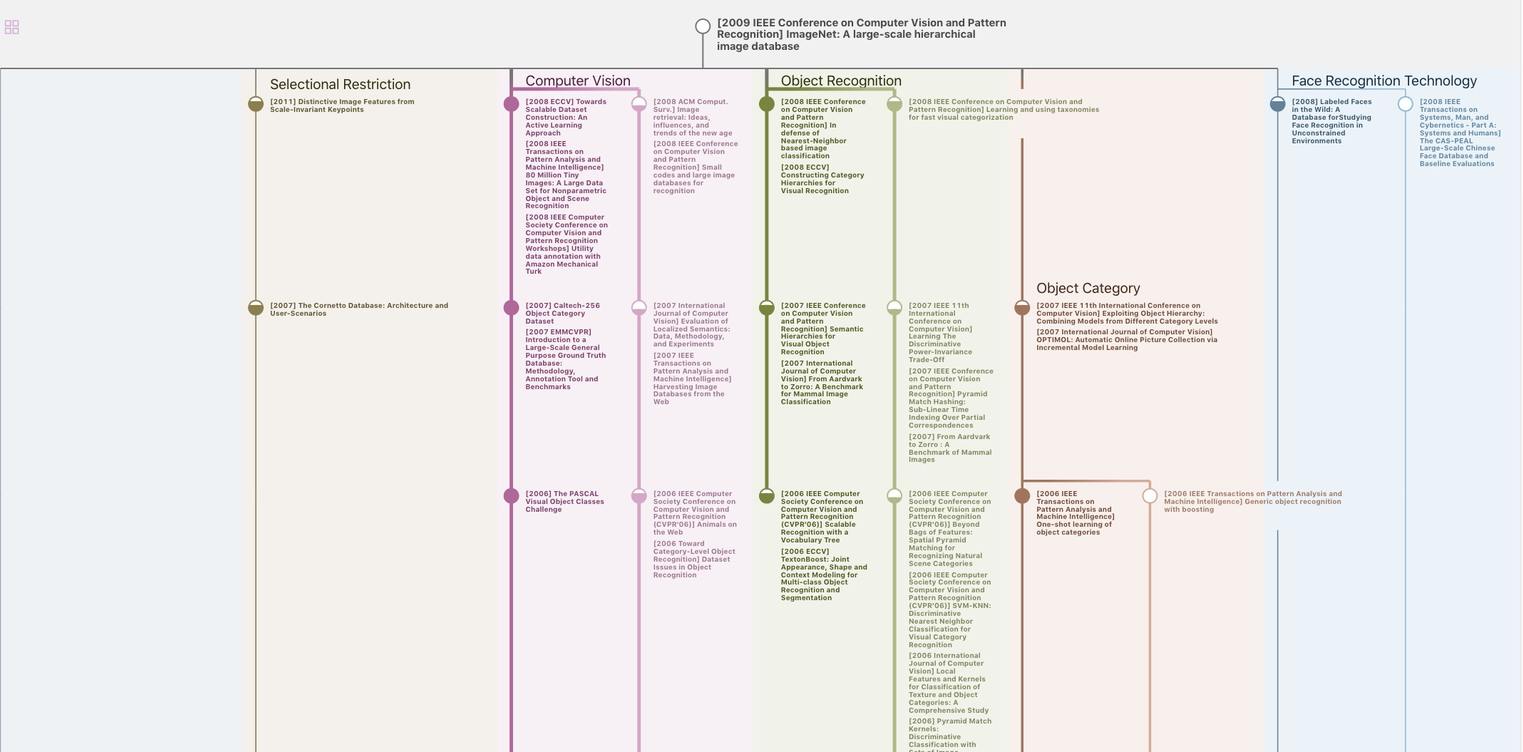
生成溯源树,研究论文发展脉络
Chat Paper
正在生成论文摘要