Harnessing causal forests for epidemiologic research: key considerations
AMERICAN JOURNAL OF EPIDEMIOLOGY(2024)
摘要
Assessing heterogeneous treatment effects (HTEs) is an essential task in epidemiology. The recent integration of machine learning into causal inference has provided a new, flexible tool for evaluating complex HTEs: causal forest. In a recent paper, Jawadekar et al (Am J Epidemiol. 2023;192(7):1155-1165) introduced this innovative approach and offered practical guidelines for applied users. Building on their work, this commentary provides additional insights and guidance to promote the understanding and application of causal forest in epidemiologic research. We start with conceptual clarifications, differentiating between honesty and cross-fitting, and exploring the interpretation of estimated conditional average treatment effects. We then delve into practical considerations not addressed by Jawadekar et al, including motivations for estimating HTEs, calibration approaches, and ways to leverage causal forest output with examples from simulated data. We conclude by outlining challenges to consider for future advancements and applications of causal forest in epidemiologic research.
更多查看译文
关键词
causal forests,heterogeneous treatment effects,conditional average treatment effects
AI 理解论文
溯源树
样例
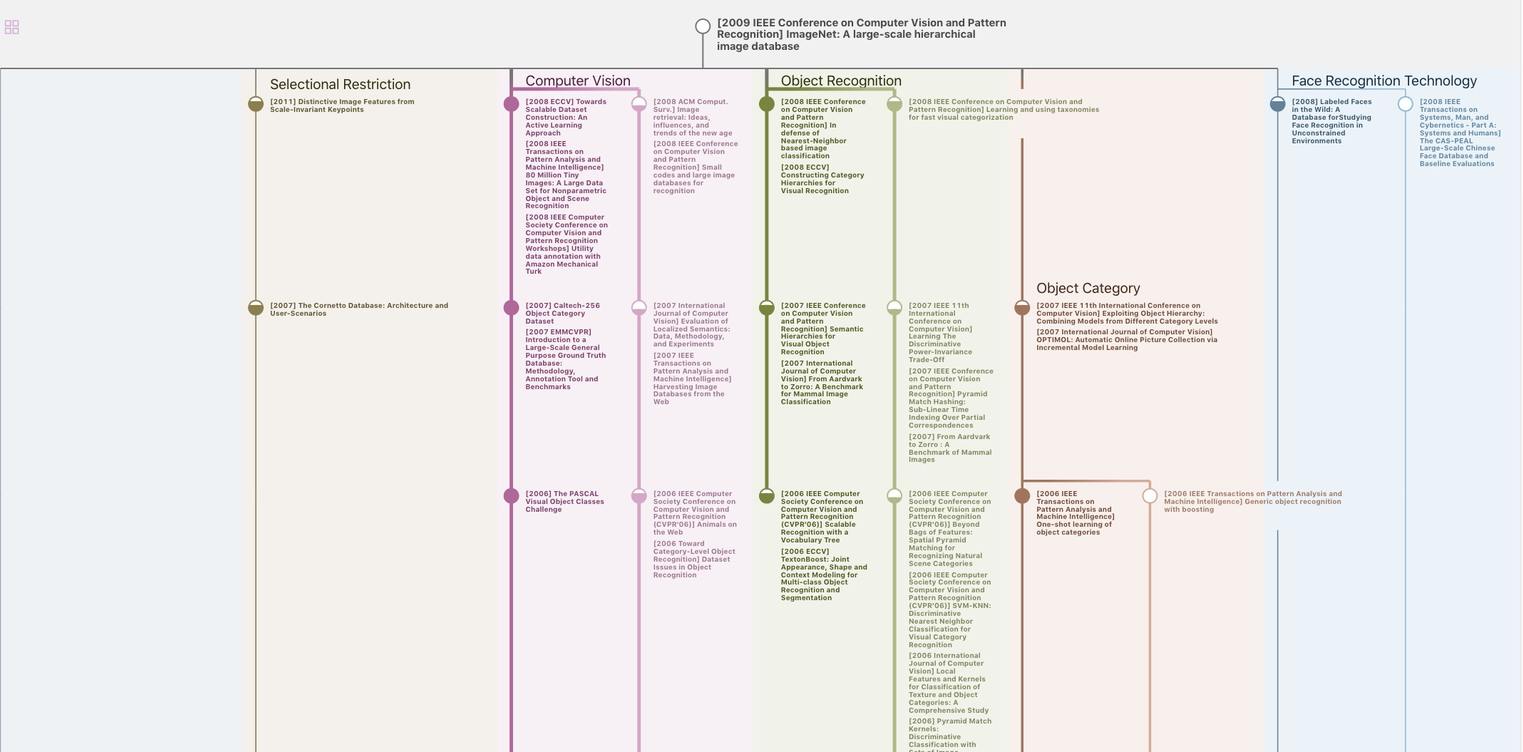
生成溯源树,研究论文发展脉络
Chat Paper
正在生成论文摘要