Using MsfNet to Predict the ISUP Grade of Renal Clear Cell Carcinoma in Digital Pathology Images
CMC-COMPUTERS MATERIALS & CONTINUA(2024)
摘要
Clear cell renal cell carcinoma (ccRCC) represents the most frequent form of renal cell carcinoma (RCC), and accurate International Society of Urological Pathology (ISUP) grading is crucial for prognosis and treatment selection. This study presents a new deep network called Multi -scale Fusion Network (MsfNet), which aims to enhance the automatic ISUP grade of ccRCC with digital histopathology pathology images. The MsfNet overcomes the limitations of traditional ResNet50 by multi -scale information fusion and dynamic allocation of channel quantity. The model was trained and tested using 90 Hematoxylin and Eosin (H&E) stained whole slide images (WSIs), which were all cropped into 320 x 320 -pixel patches at 40x magnification. MsfNet achieved a microaveraged area under the curve (AUC) of 0.9807, a macro -averaged AUC of 0.9778 on the test dataset. The Gradient -weighted Class Activation Mapping (Grad -CAM) visually demonstrated MsfNet's ability to distinguish and highlight abnormal areas more effectively than ResNet50. The t -Distributed Stochastic Neighbor Embedding (t-SNE) plot indicates our model can efficiently extract critical features from images, reducing the impact of noise and redundant information. The results suggest that MsfNet offers an accurate ISUP grade of ccRCC in digital images, emphasizing the potential of AI -assisted histopathological systems in clinical practice.
更多查看译文
关键词
Renal cell carcinoma,computer-aided diagnosis,pathology image,deep learning,machine learning
AI 理解论文
溯源树
样例
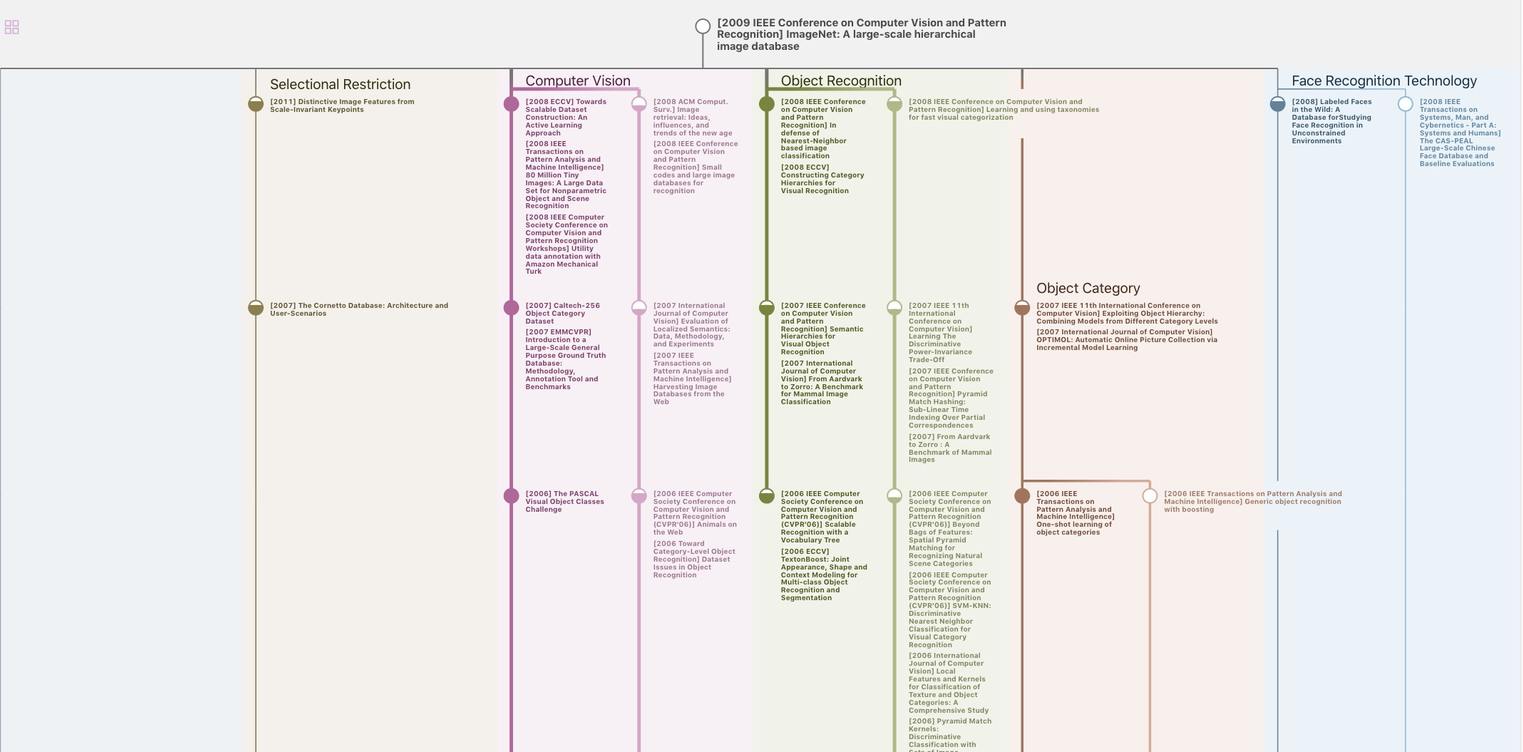
生成溯源树,研究论文发展脉络
Chat Paper
正在生成论文摘要