GraphAlign plus plus : An Accurate Feature Alignment by Graph Matching for Multi-Modal 3D Object Detection
IEEE TRANSACTIONS ON CIRCUITS AND SYSTEMS FOR VIDEO TECHNOLOGY(2024)
摘要
LiDAR and camera are complementary sensors for 3D object detection in autonomous driving. However, it is challenging to explore the unnatural interaction between point clouds and images, and the critical factor is how to conduct feature alignment of these heterogeneous modalities. Currently, many methods achieve feature alignment through projection calibration, without accounting for the impact of sensors misalignment errors, resulting in sub-optimal performance. In this paper, we present GraphAlign++, a more accurate feature alignment framework for 3D object detection by graph matching. Specifically, we construct the nearest neighbor relationship by calculating Euclidean distances of point cloud features within the subspaces. Through the projection calibration between the image and point cloud pairs, we project the nearest neighbors of point cloud features onto the corresponding image. Then by matching the nearest neighbors of a single point-feature of the point cloud with multiple pixel-features of the image, we search for a more appropriate feature alignment. In addition, we provide a self-attention module to enhance the weights of significant relations to fine-tune the feature alignment between these two heterogeneous modalities. Extensive experiments on nuScenes benchmark demonstrate the effectiveness and efficiency of GraphAlign++. Notably, due to the more accurate feature alignment, which contributes to increase mAP by 3.10% on KITTI test hard level, our method is remarkably beneficial for long-range object detection.
更多查看译文
关键词
Three-dimensional displays,Point cloud compression,Feature extraction,Object detection,Laser radar,Semantics,Cameras,3D object detection,multi-modal fusion,feature alignment,local graphs
AI 理解论文
溯源树
样例
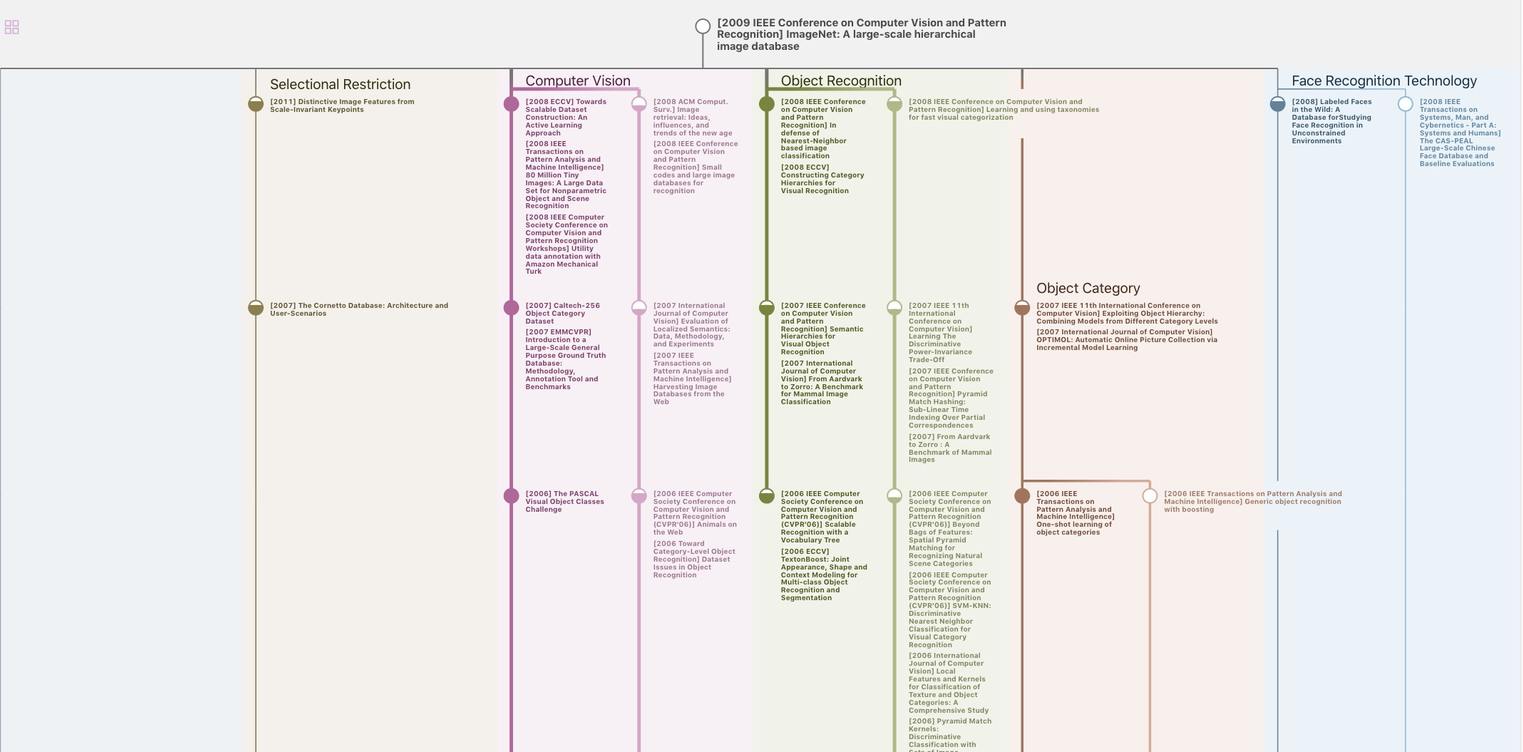
生成溯源树,研究论文发展脉络
Chat Paper
正在生成论文摘要