A NOVEL l0 MINIMIZATION FRAMEWORK OF TENSOR TUBAL RANK AND ITS MULTI-DIMENSIONAL IMAGE COMPLETION APPLICATION
INVERSE PROBLEMS AND IMAGING(2024)
摘要
. Recently, minimizing the tensor tubal rank based on the tensor singular value decomposition (t-SVD) has attracted significant attention in the tensor completion task. The widely-used solutions of tensor-tubal-rank minimization rely upon various convex and nonconvex surrogates of the tensor rank. However, these tensor rank surrogates usually lead to inaccurate descriptions of the tensor rank. To mitigate the limitation, we propose an innovative L0 minimization framework with guaranteed convergence to provide a novel paradigm for minimization of the tensor rank. To demonstrate the effectiveness of our framework, we develop a new tensor completion model employing a tensor adaptive sparsity-deduced rank (TASR). Subsequently, we formulate an algorithm rooted in the proposed t0 minimization framework to address this model effectively. Experimental results on multi-dimensional image data demonstrate that our method is superior to several state-of-the-art approaches. The code is accessible at https://github.com/Jin-liangXiao/L0-TC.
更多查看译文
关键词
Rank minimization,rank surrogate,tensor completion,mathematical program with equilibrium constraints (MPEC),proximal alternating direction method of multipliers (PADMM)
AI 理解论文
溯源树
样例
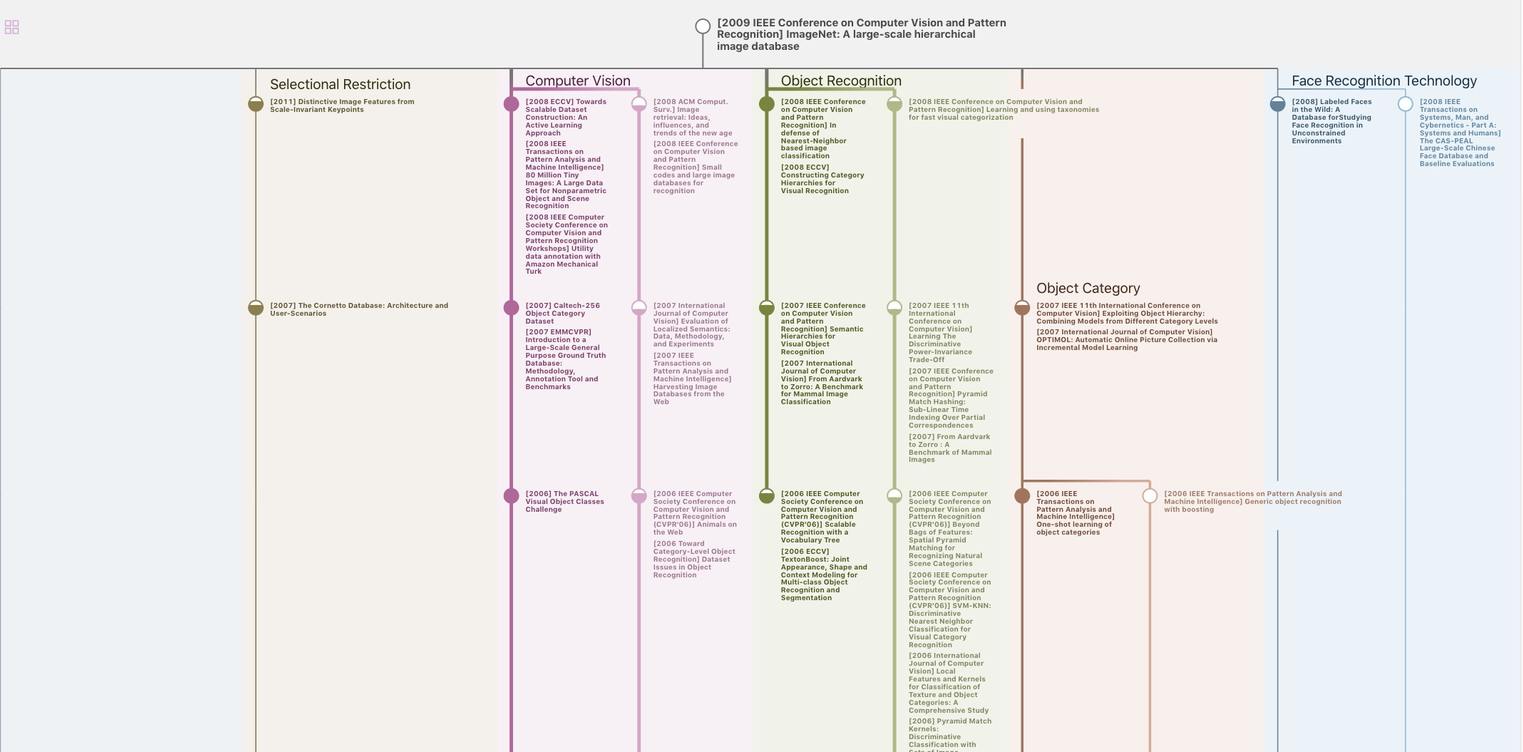
生成溯源树,研究论文发展脉络
Chat Paper
正在生成论文摘要