Dimensionality reduction of rotor fault dataset based on joint embedding of multi-class graphs
EKSPLOATACJA I NIEZAWODNOSC-MAINTENANCE AND RELIABILITY(2024)
摘要
Traditional dimensionality reduction techniques usually rely on a single or a limited number of similar graphs for graph embedding, which limits their ability to extract more information about the internal structure of the data. To address this problem, this study proposes a rotor fault dataset dimensionality reduction algorithm based on multi -class graph joint embedding (MCGJE). The algorithm first overcomes the defect that the traditional feature space cannot take both local and global information into account by constructing local and global median feature line graphs; secondly, based on the graph embedding framework, the algorithm also constructs a hypergraph structure for inscribing complex multivariate relationships between high -dimensional data in the feature space, which in turn enables it to contain more fault information. Finally, we conducted two different rotor fault simulation experiments. The results show that the MCGJE-based algorithm has robust dimensionality reduction capability and can significantly improve the accuracy of fault identification.
更多查看译文
关键词
Multi-class graphs,dimensionality reduction,hypergraph structure,median feature line graph
AI 理解论文
溯源树
样例
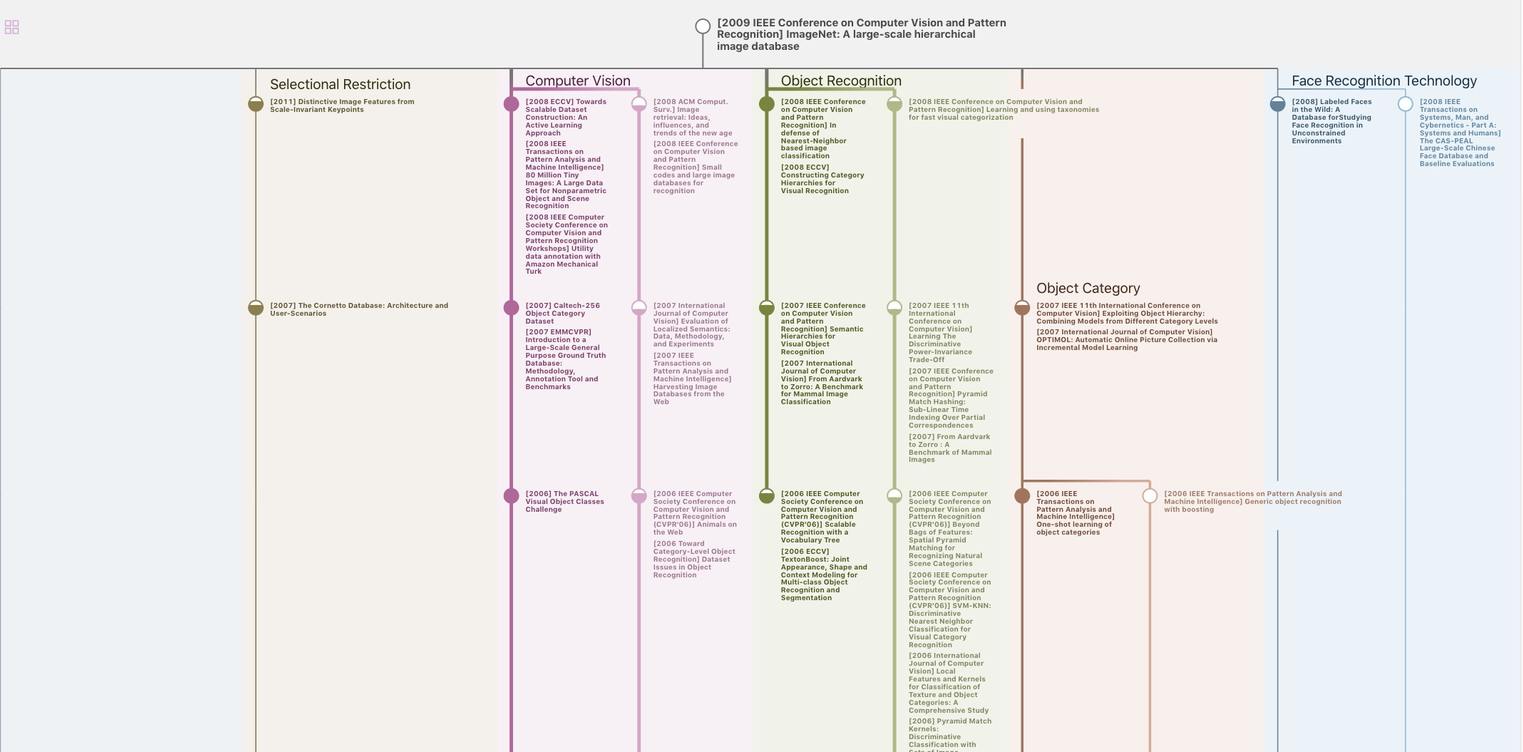
生成溯源树,研究论文发展脉络
Chat Paper
正在生成论文摘要