Multi-Objective Equilibrium Optimizer for Feature Selection in High-Dimensional English Speech Emotion Recognition
CMC-COMPUTERS MATERIALS & CONTINUA(2024)
摘要
Speech emotion recognition (SER) uses acoustic analysis to find features for emotion recognition and examines variations in voice that are caused by emotions. The number of features acquired with acoustic analysis is extremely high, so we introduce a hybrid filter -wrapper feature selection algorithm based on an improved equilibrium optimizer for constructing an emotion recognition system. The proposed algorithm implements multi -objective emotion recognition with the minimum number of selected features and maximum accuracy. First, we use the information gain and Fisher Score to sort the features extracted from signals. Then, we employ a multi -objective ranking method to evaluate these features and assign different importance to them. Features with high rankings have a large probability of being selected. Finally, we propose a repair strategy to address the problem of duplicate solutions in multi -objective feature selection, which can improve the diversity of solutions and avoid falling into local traps. Using random forest and K -nearest neighbor classifiers, four English speech emotion datasets are employed to test the proposed algorithm (MBEO) as well as other multi -objective emotion identification techniques. The results illustrate that it performs well in inverted generational distance, hypervolume, Pareto solutions, and execution time, and MBEO is appropriate for high -dimensional English SER.
更多查看译文
关键词
Speech emotion recognition,filter-wrapper,high-dimensional,feature selection,equilibrium optimizer,multi-objective
AI 理解论文
溯源树
样例
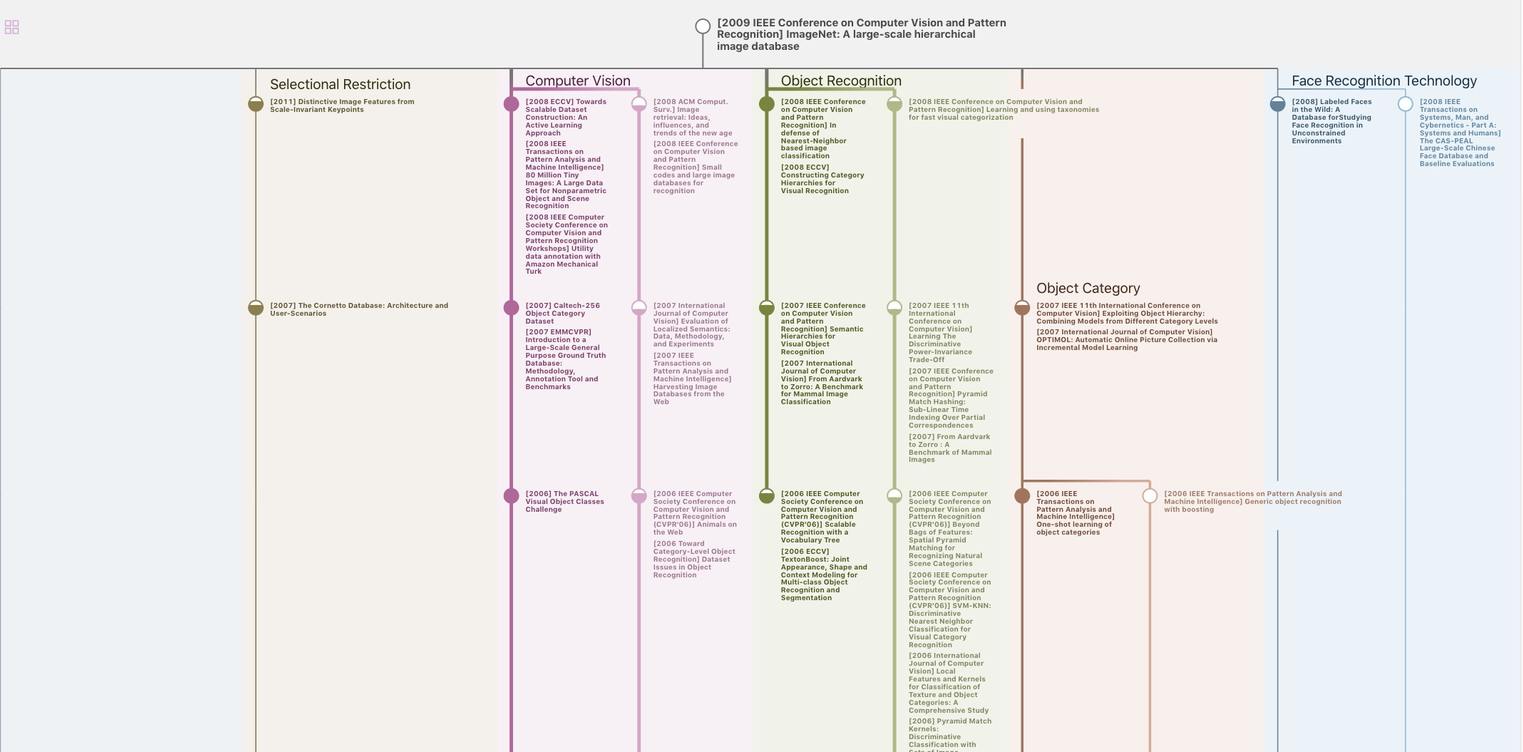
生成溯源树,研究论文发展脉络
Chat Paper
正在生成论文摘要