Structured Variational Approximations with Skew Normal Decomposable Graphical Models and Implicit Copulas
JOURNAL OF COMPUTATIONAL AND GRAPHICAL STATISTICS(2024)
摘要
Although there is much recent work developing flexible variational methods for Bayesian computation, Gaussian approximations with structured covariance matrices are often preferred computationally in high-dimensional settings. This article considers approximate inference methods for complex latent variable models where the posterior is close to Gaussian, but with some skewness in the posterior marginals. We consider skew decomposable graphical models (SDGMs), which are based on the closed skew normal family of distributions, as variational approximations. These approximations can reflect the true posterior conditional independence structure and capture posterior skewness. To increase flexibility, implicit copula SDGM approximations are also developed, where elementwise transformations of an approximately standardized SDGM random vector are considered. This implicit copula extension is an important contribution of our work, and improves the accuracy of SDGM approximations for only a modest increase in computational cost. Our parameterization of the copula approximation is novel, even in the Gaussian case. Performance of the methods is examined in a number of real examples involving generalized linear mixed models and state space models. Supplemental materials including code and appendix are available online.
更多查看译文
关键词
Closed skew normal distribution,Copula variational approximation,Decomposable graphical model
AI 理解论文
溯源树
样例
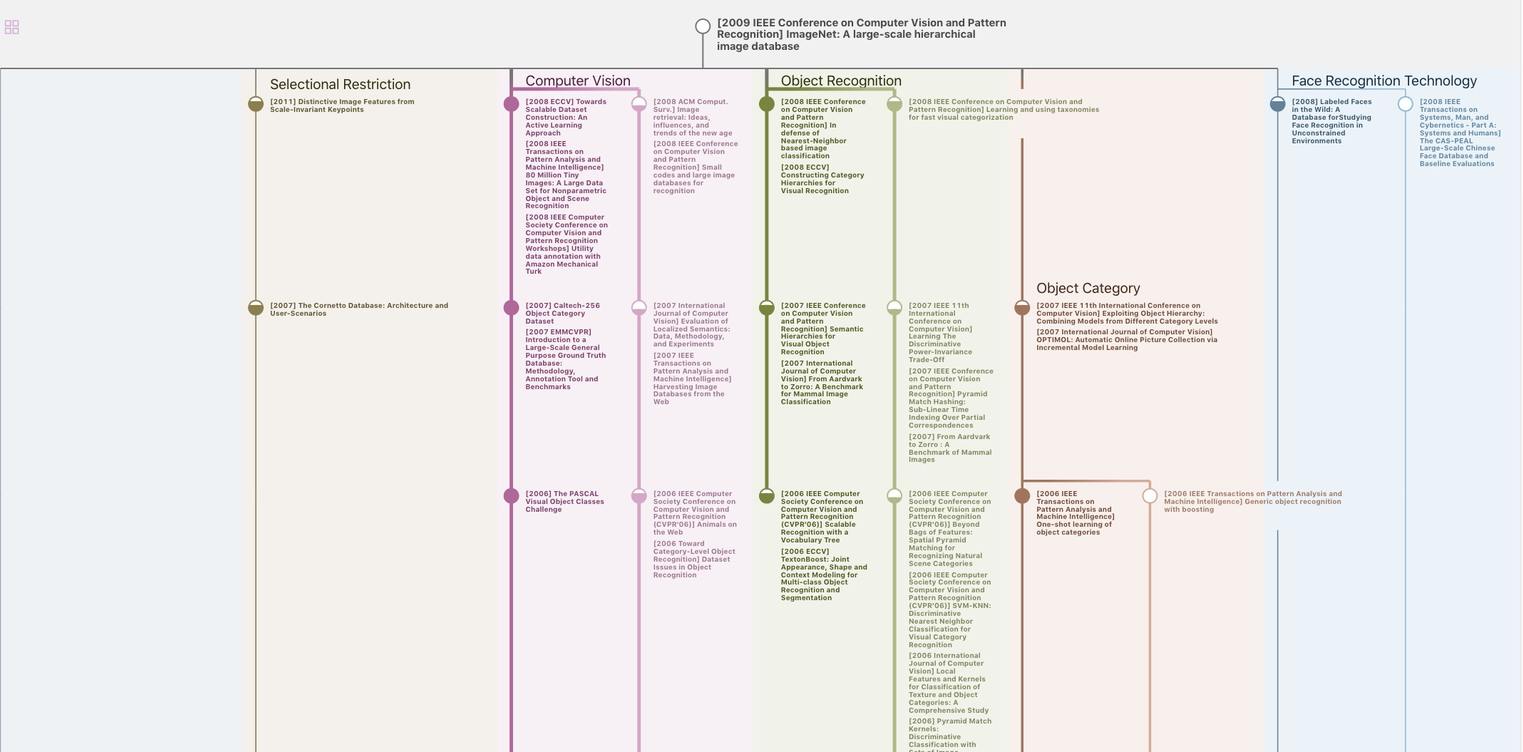
生成溯源树,研究论文发展脉络
Chat Paper
正在生成论文摘要