Subset Selection for Evolutionary Multiobjective Optimization
IEEE TRANSACTIONS ON EVOLUTIONARY COMPUTATION(2024)
摘要
Subset selection, which selects a subset of solutions according to certain criterion/indicator, is a topic closely related to evolutionary multiobjective optimization (EMO). The critical component of a multiobjective evolutionary algorithm (MOEA), environmental selection, is essentially a subset selection problem, i.e., selecting $N$ solutions as the next-generation population from usually $2N$ candidates (where $N$ denotes the size of the population). Another use of subset selection is the solution preprocessing procedure for decision-making, in which typically a few representatives are selected from the final population or a large-size archive, in order not to overwhelm the decision maker. Existing work for subset selection in EMO is focused on developing greedy algorithms, but may suffer from being trapped in local optima. In this article, we approach the problem by providing a multiobjective evolutionary algorithmic framework. We consider several popular quality indicators for subset evaluation and present accelerated variants of a well-studied MOEA in the theoretical study area, global simple evolutionary multiobjective optimizer (GSEMO), for each indicator. We conduct rigorous theoretical analyses of the acceleration procedure. Moreover, we prove that our algorithms can achieve the best-so-far approximation guarantee by the submodularity of the indicators. We finally empirically show the effectiveness and scalability of the proposed algorithms, in addition to the potentials to be further improved by introducing popular MOEAs (e.g., using NSGA-II and MOEA/D to replace GSEMO).
更多查看译文
关键词
Evolutionary computation,Evolutionary multiobjective optimization (EMO),global simple evolutionary multiobjective algorithm (GSEMO),multiobjective evolutionary optimizer (MOEAs),quality indicators,submodularity,subset selection
AI 理解论文
溯源树
样例
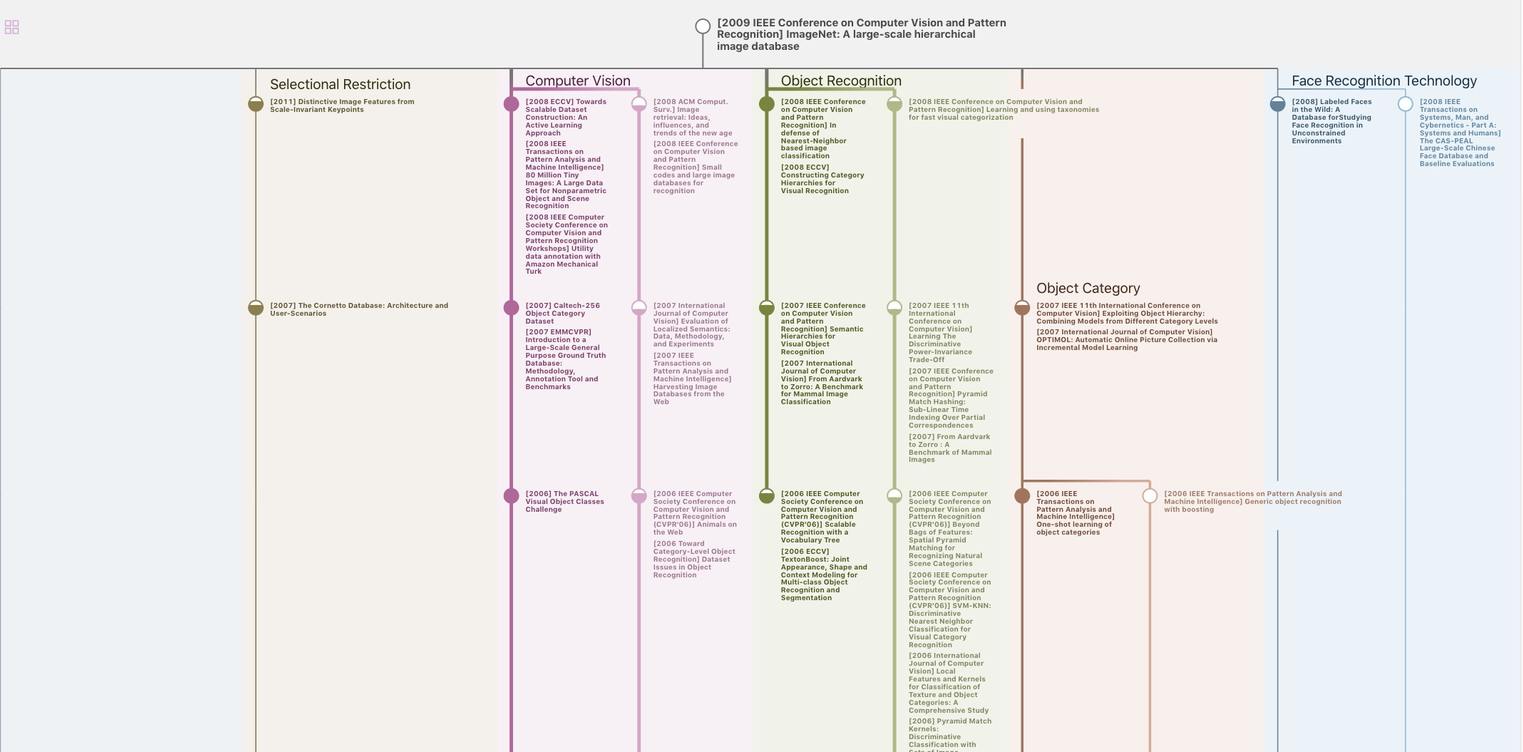
生成溯源树,研究论文发展脉络
Chat Paper
正在生成论文摘要