MCGCN: Multi-Correlation Graph Convolutional Network for Pedestrian Attribute Recognition
IEICE TRANSACTIONS ON INFORMATION AND SYSTEMS(2024)
摘要
Pedestrian attribute recognition (PAR) aims to recognize a series of a person's semantic attributes, e.g., age, gender, which plays an important role in video surveillance. This paper proposes a multi-cor relation graph convolutional network named MCGCN for PAR, which includes a semantic graph, visual graph, and synthesis graph. We construct a semantic graph by using attribute features with semantic constraints. A graph convolution is employed, based on prior knowledge of the dataset, to learn the semantic correlation. 2D features are projected onto visual graph nodes and each node corresponds to the feature region of each attribute group. Graph convolution is then utilized to learn regional correlation. The visual graph nodes are connected to the semantic graph nodes to form a synthesis graph. In the synthesis graph, regional and semantic correlation are embedded into each other through inter-graph edges, to guide each other's learning and to update the visual and semantic graph, thereby constructing semantic and regional correlation. On this basis, we use a better loss weighting strategy, the suit_polyloss, to address the imbalance of pedestrian attribute datasets. Experiments on three benchmark datasets show that the proposed approach achieves superior recognition performance compared to existing technologies, and achieves state-of-the-art performance.
更多查看译文
关键词
key pedestrian attribute recognition,synthesis graph,semantic correlation,regional correlation
AI 理解论文
溯源树
样例
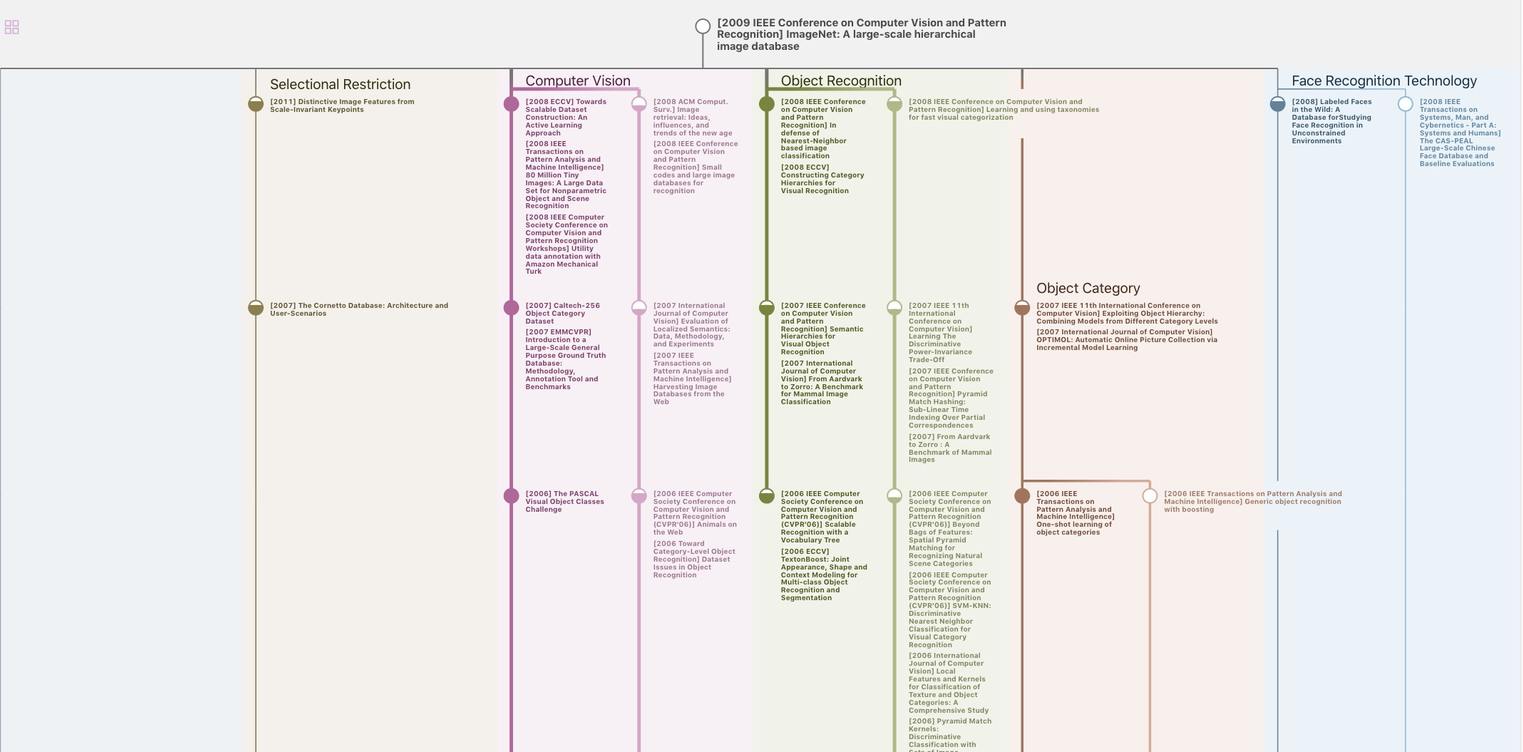
生成溯源树,研究论文发展脉络
Chat Paper
正在生成论文摘要