A Laplace operator-based active contour model with improved image edge detection performance
Digital Signal Processing(2024)
摘要
Active contour model (ACM) is an important branch in the field of image segmentation, since it adapts to different image types and scenes. However, traditional ACMs rely on local image information, which makes them sensitive to initial contours and time-consuming. In this paper, a Laplace operator based ACM (LOACM) with improved image edge detection performance is proposed. LOACM combines the global pre-fitting function which is constructed by using the image's second-order gradient information with the adaptive boundary indicator function to build a hybrid model. Its compact code size allows it to be executed on a wide range of edge devices. To substantiate the segmentation capability of the LOACM model, the BSD500 dataset was utilized for experimentation. The results indicate that LOACM exhibits excellent performance, with average IOU and DSC scores of 0.861 and 0.924 respectively. Additionally, it consistently handles processes within an average time of less than 0.3s, significantly less than that of comparative models, providing compelling evidence of LOACM's exceptional efficiency and precision.
更多查看译文
关键词
Image segmentation,Active contour model,Level set method,Global pre-fitting,Edge indicator function
AI 理解论文
溯源树
样例
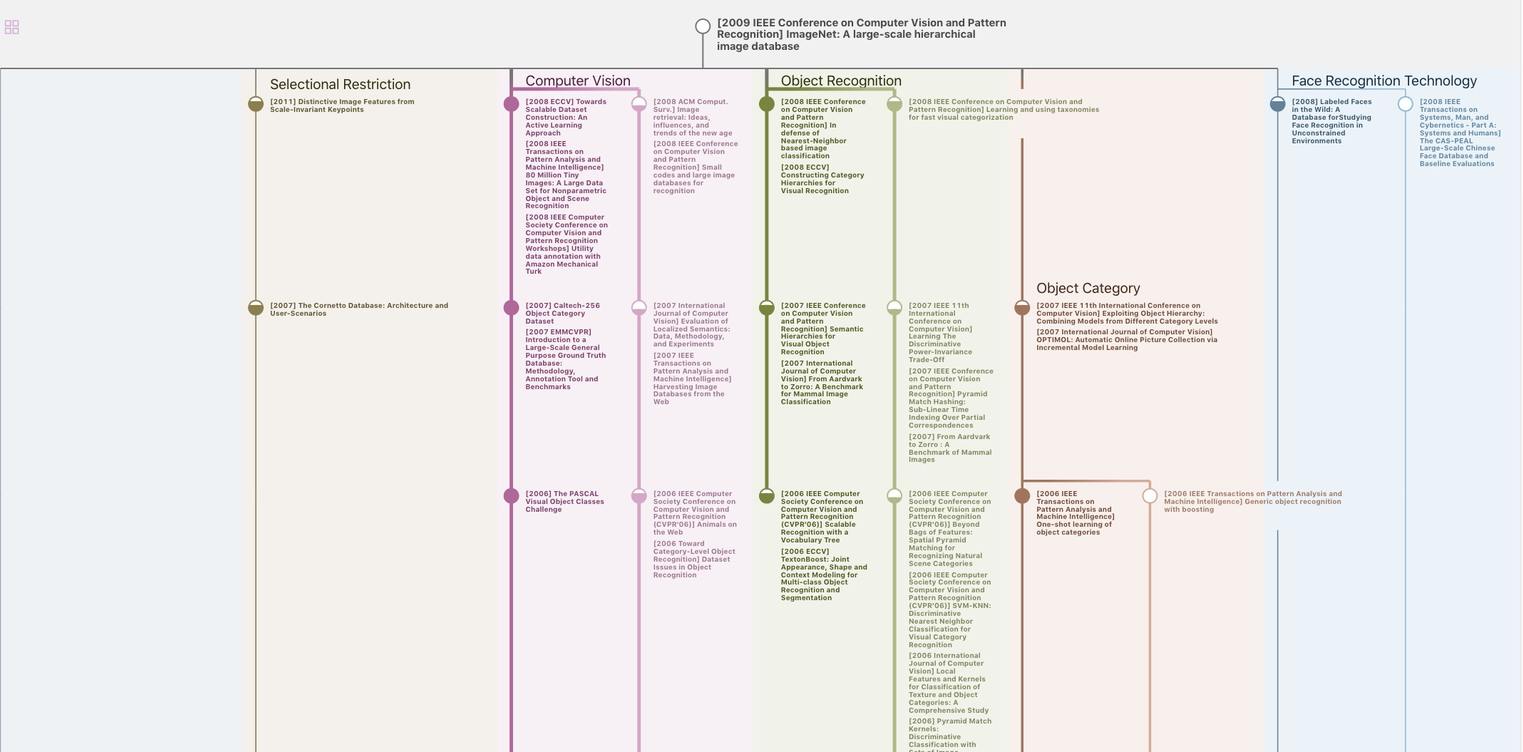
生成溯源树,研究论文发展脉络
Chat Paper
正在生成论文摘要