Application of reinforcement learning to deduce nuclear power plant severe accident scenario
Annals of Nuclear Energy(2024)
摘要
Severe accident scenarios for nuclear power plants are determined through probabilistic safety analysis (PSA). In this process, it is possible to identify the failure sequence of specific components, but assessing the impact of component failure time on the severity of accident remains a challenge. In this study, a novel approach is presented that utilizes machine learning methodologies such as reinforcement learning (RL) to complement traditional PSA. The proposed process was validated by comparing whether the most severe accident scenarios obtained through critical accident simulations can be reproduced by a RL, since this is a novel use of machine learning techniques. The comparison shows feasibility of exploring critical accident scenarios using RL. To implement the reinforcement learning methodology based on the existing system code, supervised learning model that can predict the remaining time of reactor vessel failure was implemented in this study. Based on this prediction model and data from existing catastrophic accident simulation, RL was implemented. The results obtained from RL were subsequently validated with the results of severe accident code simulation. In summary, new methodology for applying machine learning techniques to the nuclear accident analysis process was presented, and the feasibility and potential of the proposed methodology were discussed.
更多查看译文
关键词
Severe accident,Severe accident simulation,Severe accident scenario,Machine learning,Supervised learning,Reinforcement learning
AI 理解论文
溯源树
样例
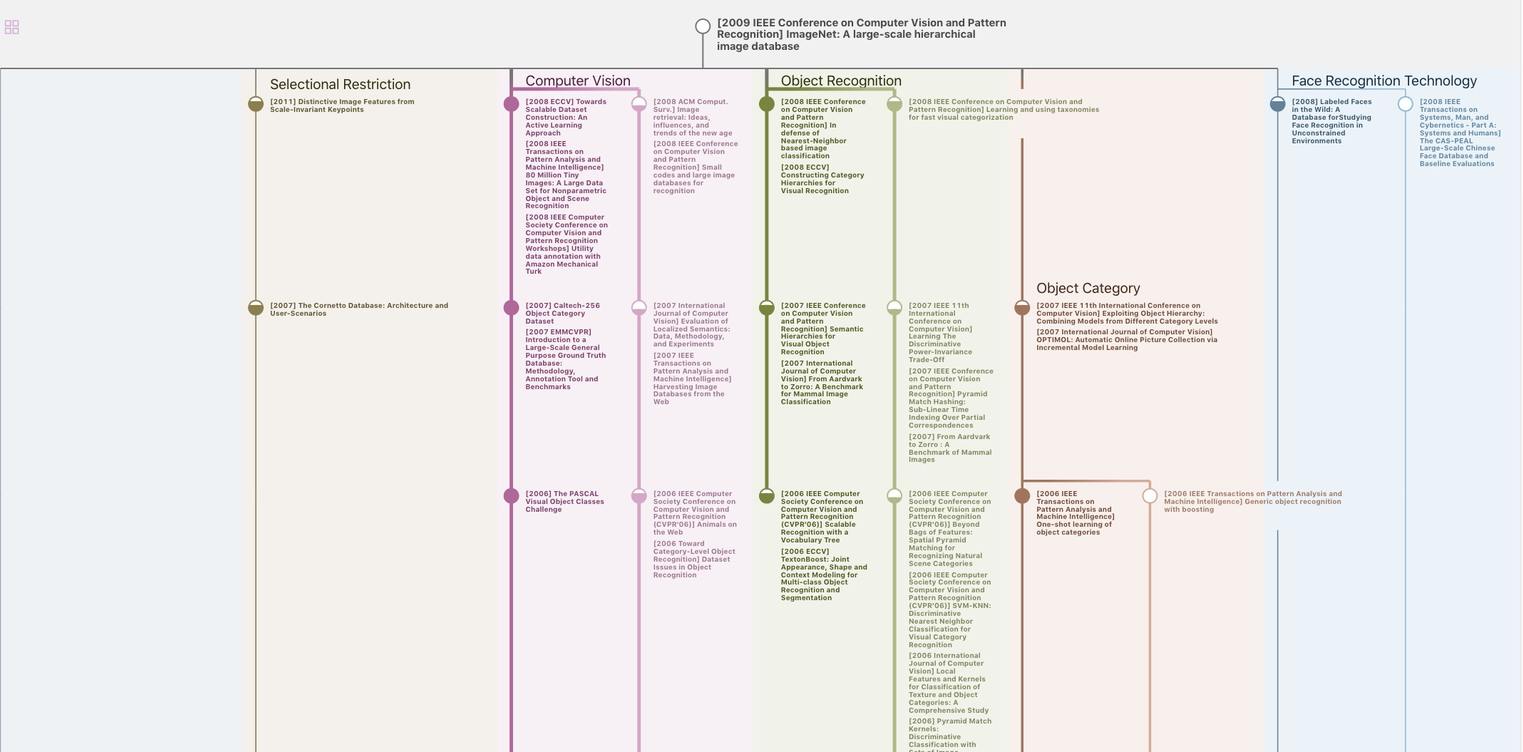
生成溯源树,研究论文发展脉络
Chat Paper
正在生成论文摘要