Fast Exact/Conservative Monte Carlo Confidence Intervals
arxiv(2024)
摘要
Monte Carlo tests about parameters can be "inverted" to form confidence sets:
the confidence set comprises all hypothesized values of the parameter that are
not rejected at level α. When the tests are exact or conservative – as
some families of such tests are – so are the confidence sets. Because the
validity of confidence sets depends only on the significance level of the test
of the true null, every null can be tested using the same Monte Carlo sample,
substantially reducing the computational burden of constructing confidence
sets: the computation count is O(n), where n is the number of data. The
Monte Carlo sample can be arbitrarily small, although the highest nontrivial
attainable confidence level generally increases as the number of Monte Carlo
replicates increases. When the parameter is real-valued and the P-value is
quasiconcave in that parameter, it is straightforward to find the endpoints of
the confidence interval using bisection in a conservative way. For some test
statistics, values for different simulations and parameter values have a simple
relationship that make more savings possible. An open-source Python
implementation of the approach for the one-sample and two-sample problems is
available.
更多查看译文
AI 理解论文
溯源树
样例
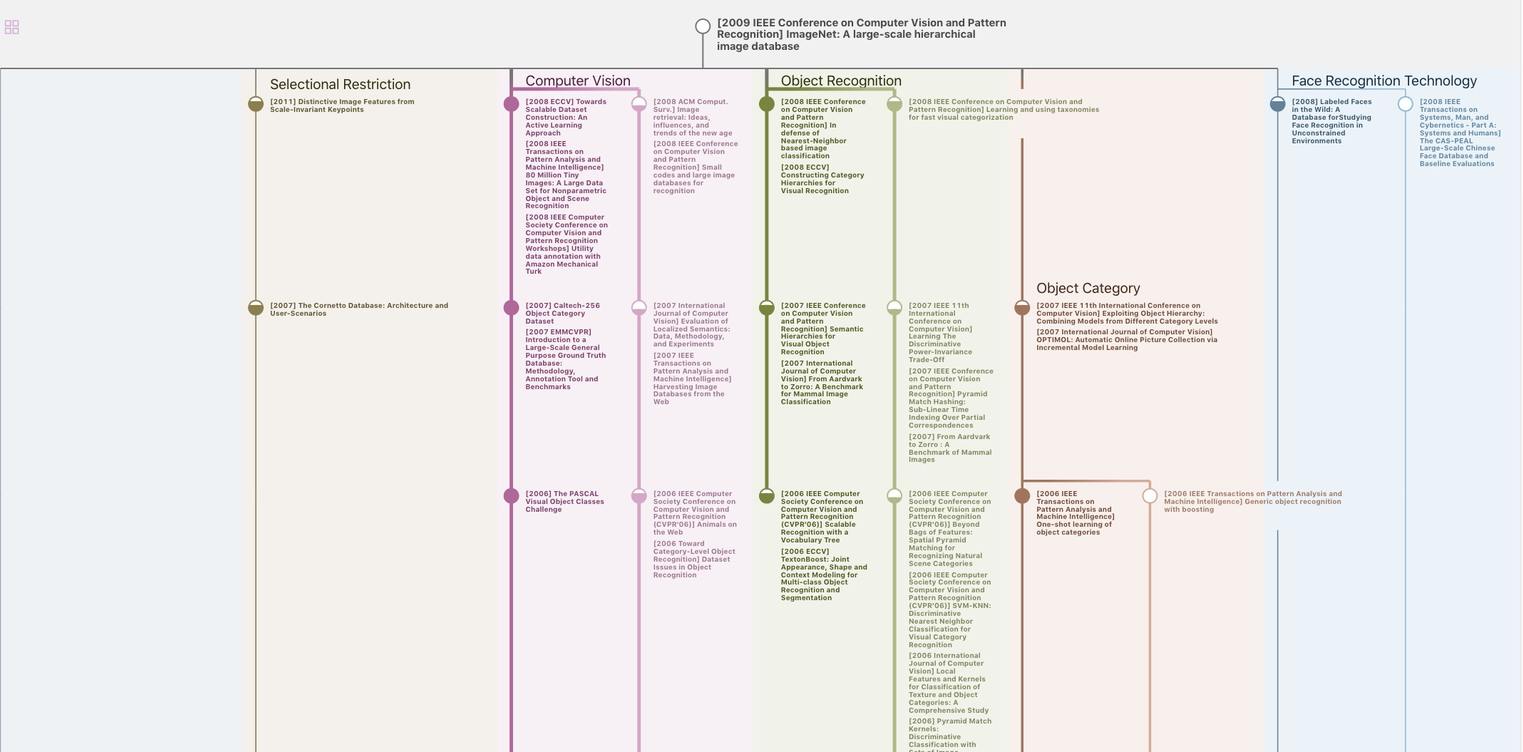
生成溯源树,研究论文发展脉络
Chat Paper
正在生成论文摘要