Machine learning aided parameter analysis in Perovskite X-ray Detector
arxiv(2024)
摘要
Many factors in perovskite X-ray detectors, such as crystal lattice and
carrier dynamics, determine the final device performance (e.g., sensitivity and
detection limit). However, the relationship between these factors remains
unknown due to the complexity of the material. In this study, we employ machine
learning to reveal the relationship between 15 intrinsic properties of halide
perovskite materials and their device performance. We construct a database of
X-ray detectors for the training of machine learning. The results show that the
band gap is mainly influenced by the atomic number of the B-site metal, and the
lattice length parameter b has the greatest impact on the carrier
mobility-lifetime product (μτ). An X-ray detector (m-F-PEA)2PbI4 were
generated in the experiment and it further verified the accuracy of our ML
models. We suggest further study on random forest regression for X-ray detector
applications.
更多查看译文
AI 理解论文
溯源树
样例
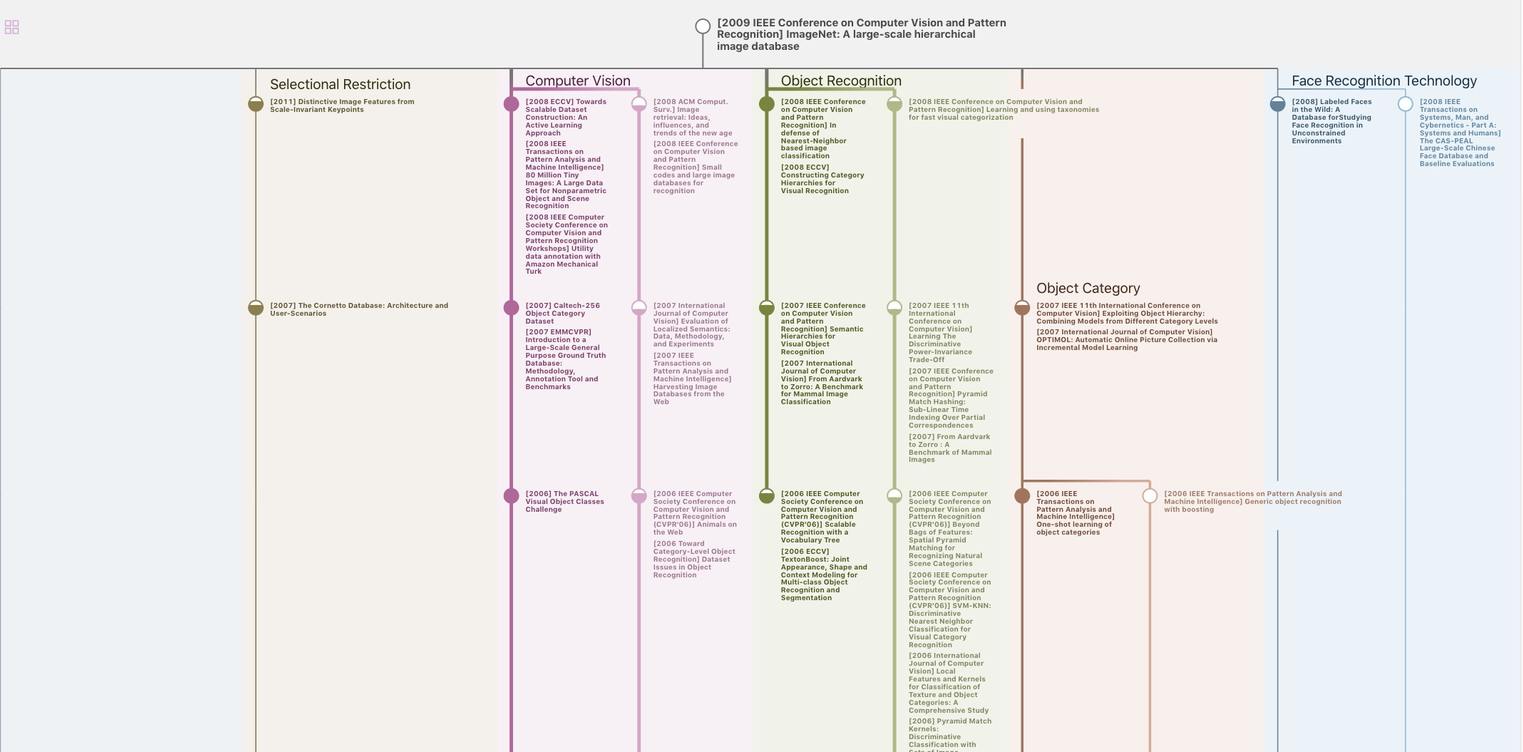
生成溯源树,研究论文发展脉络
Chat Paper
正在生成论文摘要