Many-Task Differential Evolutionary Algorithm Based on Bi-Space Similarity
IEEE Transactions on Evolutionary Computation(2024)
摘要
Many-task differential evolutionary algorithm is an effective way to optimize multiple tasks simultaneously. The optimization performance of the algorithm decreases due to the negative transfer when the number of tasks is large. To address this problem, a many-task differential evolutionary algorithm based on bi-space similarity (MaTDE-BSS) is proposed to improve the positive transfer. First, the bi-space similarity metric is designed to characterize inter-task similarity quantitatively. The decision space similarity and objective space similarity are considered simultaneously in the bi-space similarity metric. Second, a task selection strategy based on evolutionary state is proposed to select the optimal source task from the source task library accurately. The source task library based on bi-space similarity metric is built for storing source tasks. Finally, a dynamic knowledge transfer strategy is proposed to improve the efficiency of knowledge positive transfer in the many-task optimization. Parameters of the knowledge transfer strategy are adjusted according to bi-space similarity metric adaptively. In addition, the experimental results show that MaTDE-BSS is able to evaluate the inter-task similarity more comprehensively. And MaTDE-BSS is more competitive compared to other many-task evolutionary algorithms.
更多查看译文
关键词
Many-task optimization,differential evolutionary algorithm,bi-space similarity,task selection,knowledge transfer
AI 理解论文
溯源树
样例
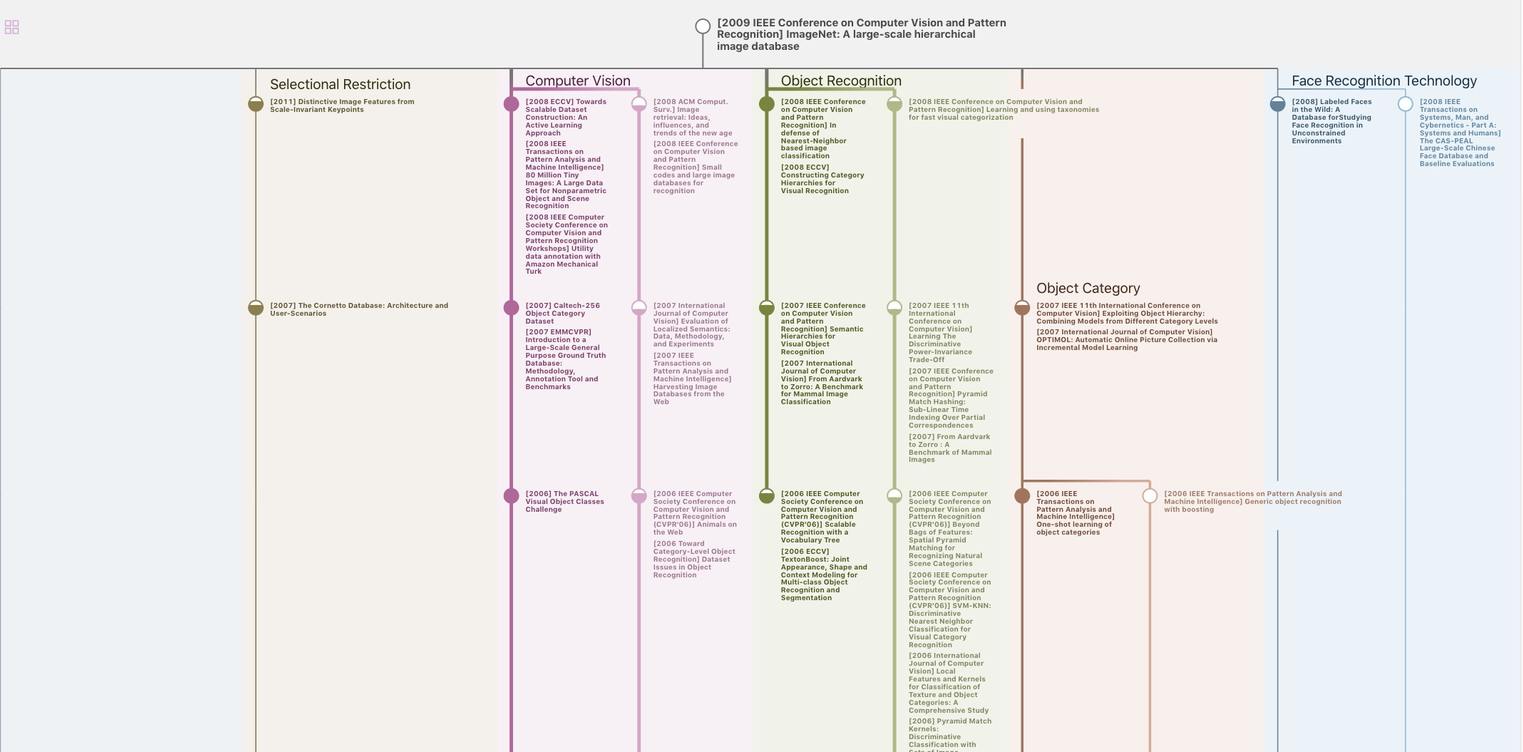
生成溯源树,研究论文发展脉络
Chat Paper
正在生成论文摘要