Accelerating Speculative Decoding using Dynamic Speculation Length
arxiv(2024)
摘要
Speculative decoding is a promising method for reducing the inference latency
of large language models. The effectiveness of the method depends on the
speculation length (SL) - the number of tokens generated by the draft model at
each iteration. The vast majority of speculative decoding approaches use the
same SL for all iterations. In this work, we show that this practice is
suboptimal. We introduce DISCO, a DynamIc SpeCulation length Optimization
method that uses a classifier to dynamically adjust the SL at each iteration,
while provably preserving the decoding quality. Experiments with four
benchmarks demonstrate average speedup gains of 10.3
baselines.
更多查看译文
AI 理解论文
溯源树
样例
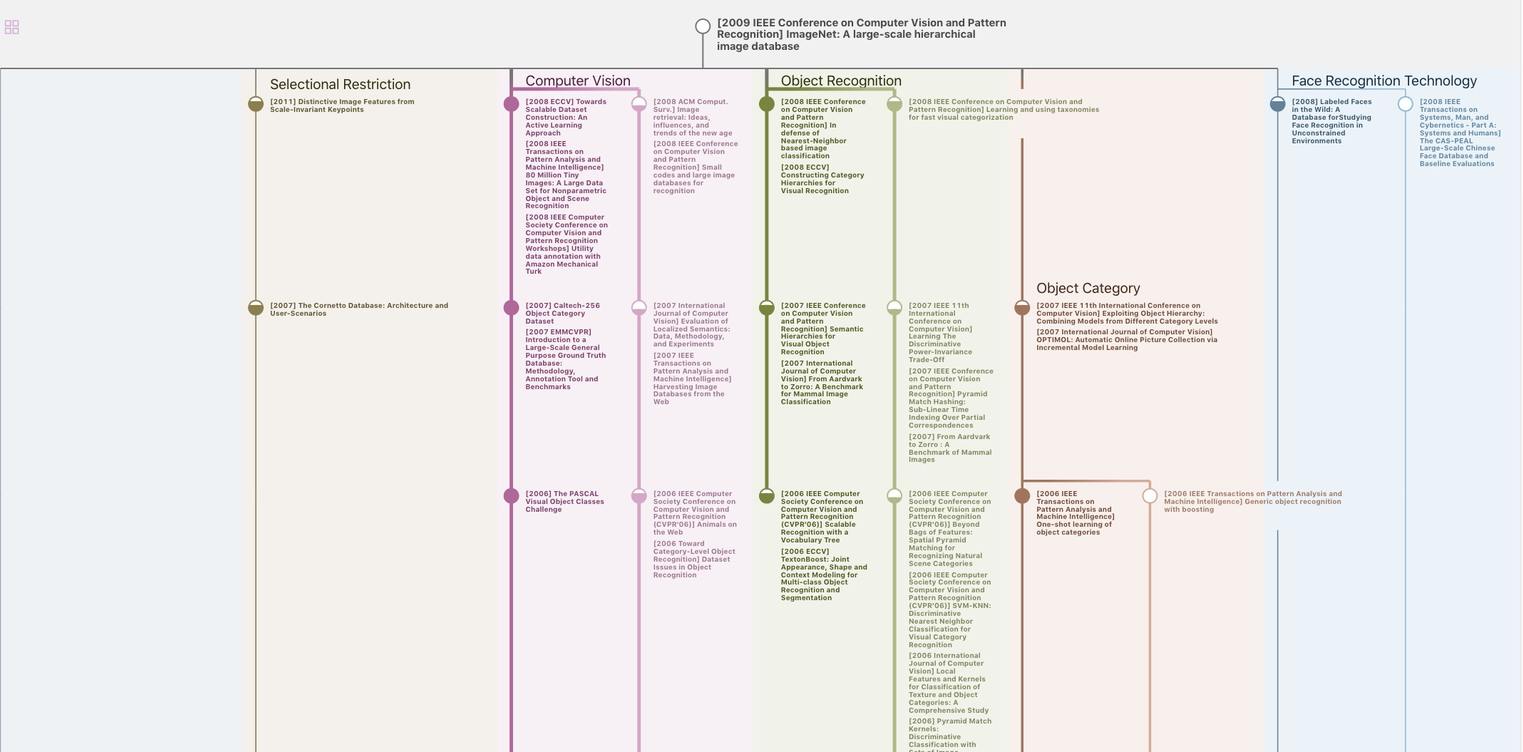
生成溯源树,研究论文发展脉络
Chat Paper
正在生成论文摘要