Federated Deep Recurrent Q-Learning for Task Partitioning and Resource Allocation in Satellite Mobile Edge Computing Assisted Industrial IoT
IEEE Internet of Things Journal(2024)
摘要
Mobile Edge Computing (MEC) deploys servers at cellular base stations to provide computing services for Industrial Internet of Things (IIoT) applications. However, in remote areas lacking cellular coverage, traditional MEC systems are ineffective. The advancement of Low Earth Orbit (LEO) satellite communication networks introduces satellite-based MEC, deploying servers on satellites, as a promising solution for remote areas. Yet, the limited resources for IIoT devices pose challenges for delivering low-latency computing services. In this paper, we investigate the problem of task partitioning and resource allocation in satellite-assisted MEC systems, aiming to minimize the task completion latency of IIoT devices. Tasks can be divided into multiple subtasks executed by local IIoT devices, MEC servers, or cloud servers. The subtasks can be independent of or dependent on each other. A proposed approach based on Deep Recurrent Q-learning Networks (DRQN) utilizes recurrent neural networks to learn temporal features resulting from satellite movement and optimize accordingly. Given the difficulty in obtaining global state information in large-scale networks, federated learning (FL) is integrated into the DRQN framework to enhance efficient decision-making by aggregating local models. The simulation validates the effectiveness of the proposed approach, demonstrating significant reductions in average latency.
更多查看译文
关键词
Satellite-assisted mobile edge computing,IIoT,task partitioning,DRQN,FL
AI 理解论文
溯源树
样例
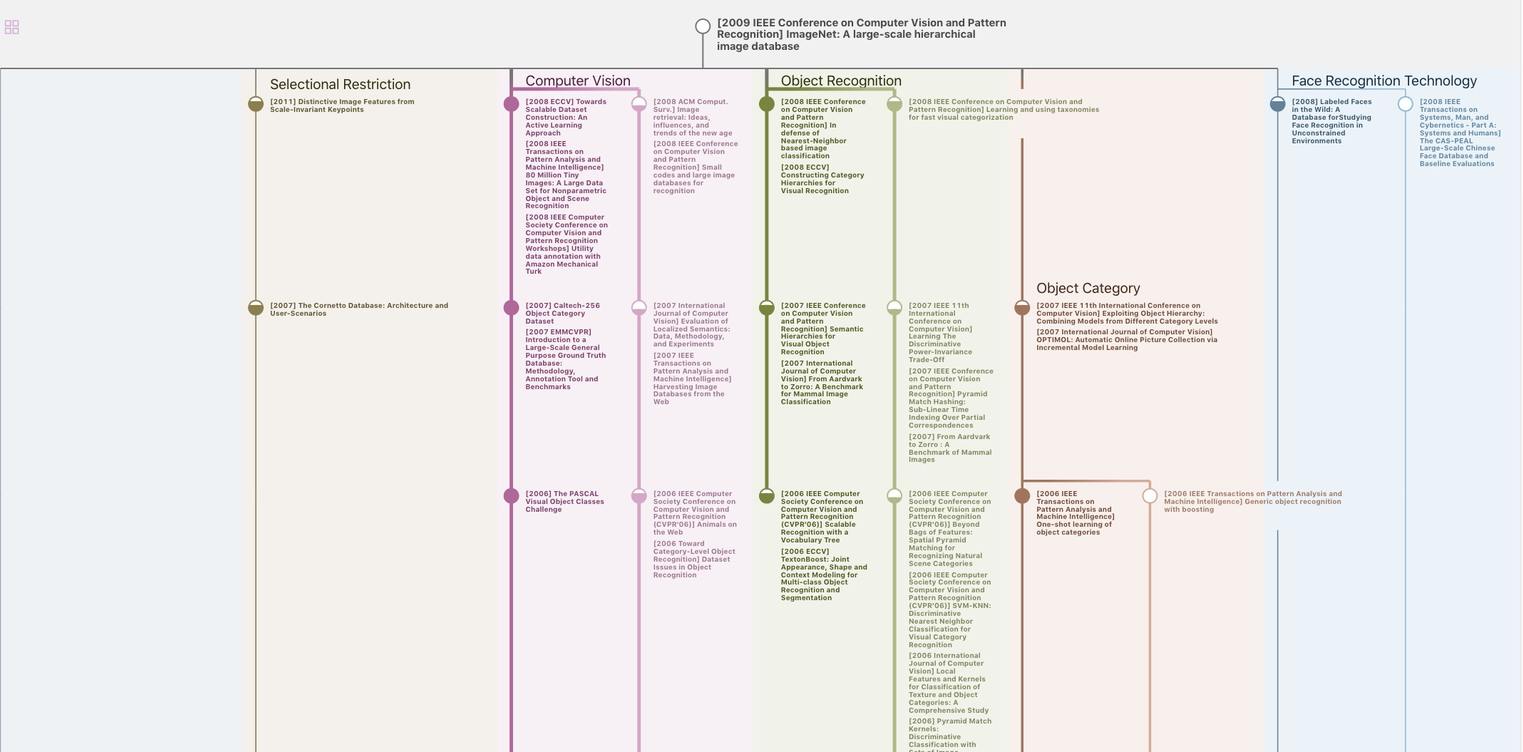
生成溯源树,研究论文发展脉络
Chat Paper
正在生成论文摘要