Unraveling the formation of oxygen vacancies on the surface of transition metal-doped ceria utilizing artificial intelligence.
Nanoscale(2024)
摘要
Ceria has been extensively utilized in different fields, with surface oxygen vacancies playing a central role. However, versatile oxygen vacancy regulation is still in its infancy. In this work, we propose an effective strategy to manipulate the oxygen vacancy formation energy via transition metal doping by combining first-principles calculations and analytical learning. We elucidate the underlying mechanism driving the formation of oxygen vacancies using combined symbolic regression and data analytics techniques. The results show that the Fermi level of the system and the electronegativity of the dopants are the paramount parameters (features) influencing the formation of oxygen vacancies. These insights not only enhance our understanding of the oxygen vacancy formation mechanism in ceria-based materials to improve their functionality but also potentially lay the groundwork for future strategies in the rational design of other transition metal oxide-based catalysts.
更多查看译文
AI 理解论文
溯源树
样例
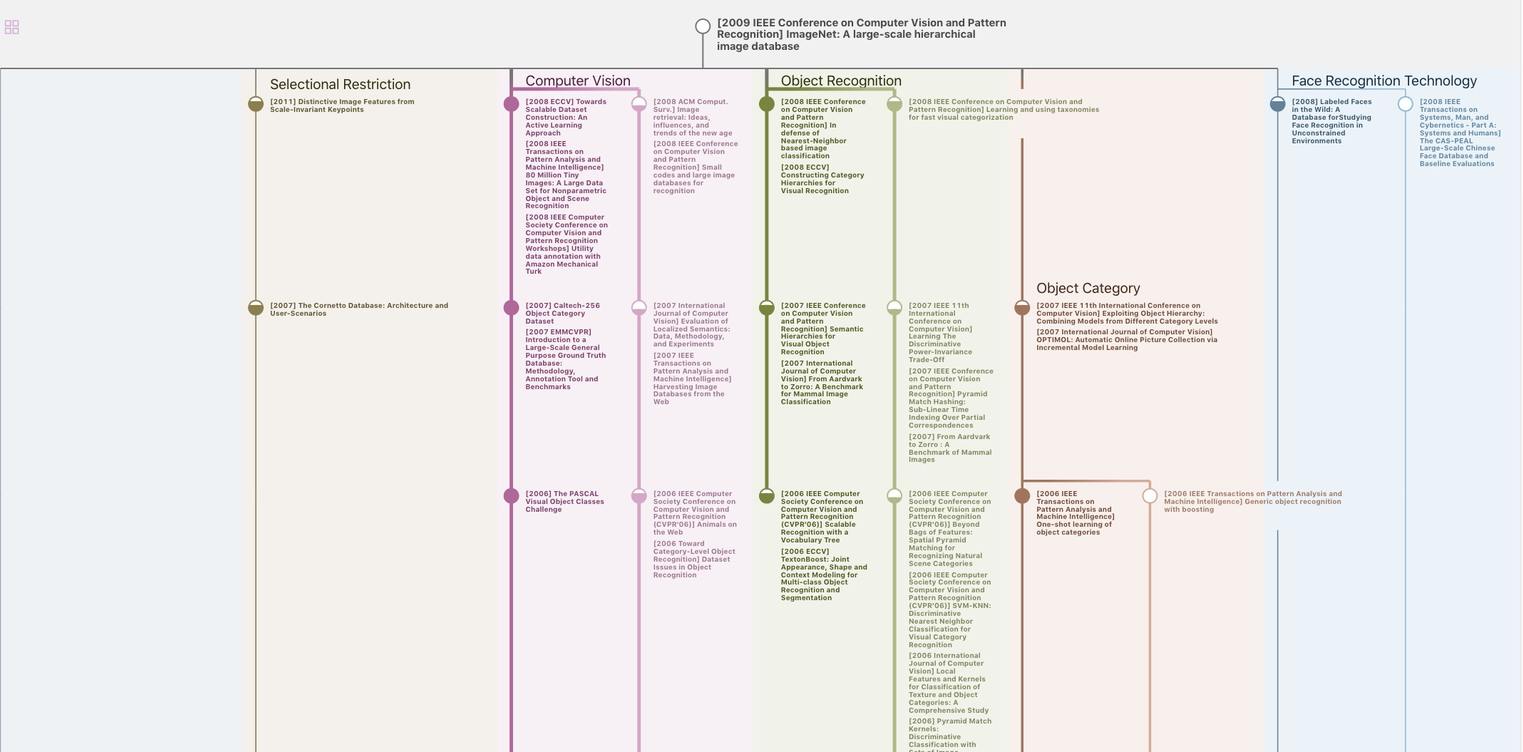
生成溯源树,研究论文发展脉络
Chat Paper
正在生成论文摘要