Channel Estimation and Equalization for SC-FDMA Using Machine Learning.
International ITG Workshop on Smart Antennas(2024)
摘要
We design neural network (NN)-based schemes for channel estimation and equalization tasks in Single-Carrier Frequency Division Multiple Access (SC-FDMA) transmission over a dispersive block-fading channel. It is demonstrated that the proposed schemes outperform their traditional counterparts for the 5G Clustered Delay Line (CDL) channel model. A significant gain is achieved compared to linear minimum mean-squared error (MMSE) equalization and Bahl-Cocke-Jelinek-Raviv (BCJR) equalizer using a pre-filter in the case of perfect channel state information (CSI) available at the receiver. The proposed NN-based channel estimator can be combined with conventional and NN-based equalizers, as well as the proposed NN-based channel equalizer can be combined with conventional channel estimators. When the proposed NN-based channel estimator and equalizer are combined, it is possible to optimize them separately or jointly. Additionally, we derive a Cramer-Rao Bound (CRB) for unbiased channel estimation error in our proposed pilot insertion regime.
更多查看译文
关键词
SC-FDMA,Channel Estimation,Channel Equalization,Machine Learning for Communications
AI 理解论文
溯源树
样例
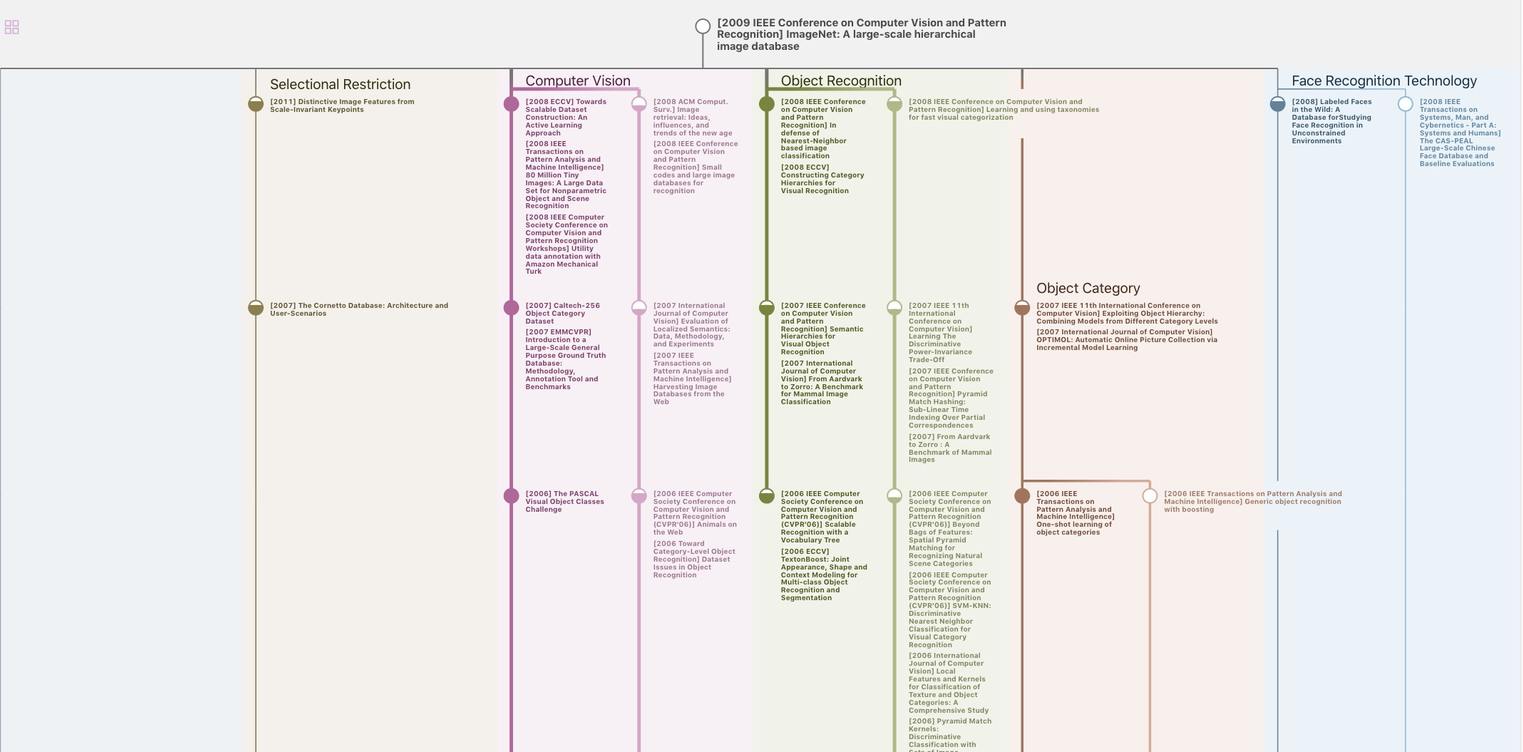
生成溯源树,研究论文发展脉络
Chat Paper
正在生成论文摘要