A Self-Distillation Contrastive Learning Architecture for Global and Local Underwater Terrain Feature Extraction and Matching
IEEE Sensors Journal(2024)
摘要
Terrain matching is a core component of underwater terrain-aided navigation system, which determines the accuracy of the underwater vehicle’s localization. Traditional terrain matching methods are lacking in improving the matching performance by extracting effective terrain features, and the effects of sample noise and rotational changes in the measurement data on the matching precision and matching accuracy are not well addressed. This paper proposes a deep learning method for extracting global style and local detail terrain features, which yields terrain feature mappings with enhanced representational capabilities. Meanwhile, without using any sample labels and negative samples, we propose an end-to-end learning framework that combines self-distillation and contrastive learning to achieve characterization learning for two augmented samples of the same terrain data. To improve the model’s ability to resist sample noise and rotational variations, we implement data augmentation by simulating measurement noise and rotational variations in underwater topographic measurements. In addition, we achieved high-precision terrain matching by comparing the abstract representations between samples rather than the terrain elevation values themselves. We have done a large number of comparison experiments with other methods, and the results show that our method has gained superior matching performance. The proposed method also enables high-precision matching of terrain data with varying sizes and resolutions, addressing the issue of limited high-resolution terrain data for training in real-world applications. Simulation test results show that our proposed method can obtain good localization accuracy in both rough and flat areas with good robustness.
更多查看译文
关键词
Attention Mechanism,Contrastive Learning,Knowledge Distillation,Terrain Matching,Underwater Vehicles
AI 理解论文
溯源树
样例
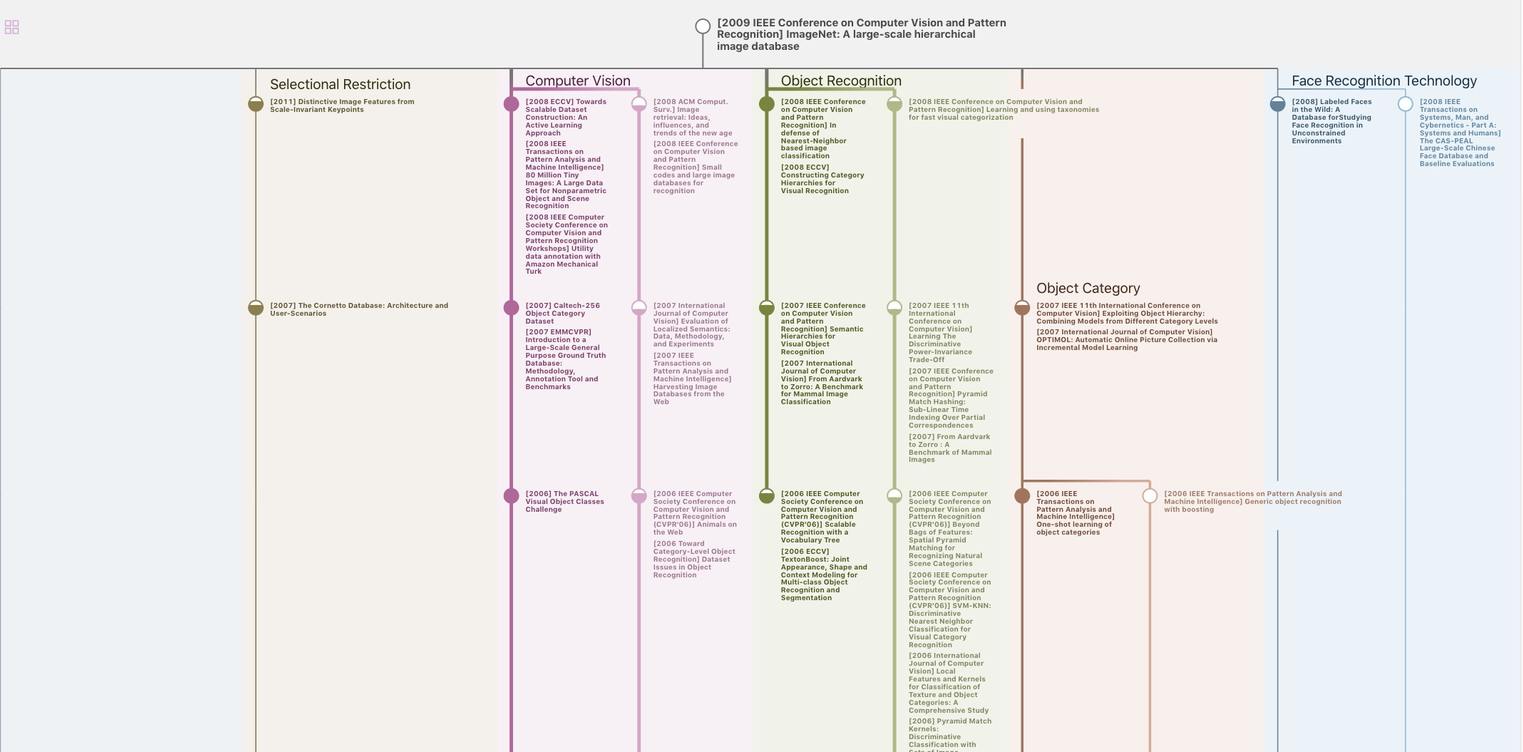
生成溯源树,研究论文发展脉络
Chat Paper
正在生成论文摘要