Reservoir temperature prediction based on characterization of water chemistry data-case study of western Anatolia, Turkey.
Scientific reports(2024)
摘要
Reservoir temperature estimation is crucial for geothermal studies, but traditional methods are complex and uncertain. To address this, we collected 83 sets of water chemistry and reservoir temperature data and applied four machine learning algorithms. These models considered various input factors and underwent data preprocessing steps like null value imputation, normalization, and Pearson coefficient calculation. Cross-validation addressed data volume issues, and performance metrics were used for model evaluation. The results revealed that our machine learning models outperformed traditional fluid geothermometers. All machine learning models surpassed traditional methods. The XGBoost model, based on the F-3 combination, demonstrated the best prediction accuracy with an R2 of 0.9732, while the Bayesian ridge regression model using the F-4 combination had the lowest performance with an R2 of 0.8302. This study highlights the potential of machine learning for accurate reservoir temperature prediction, offering geothermal professionals a reliable tool for model selection and advancing our understanding of geothermal resources.
更多查看译文
关键词
Reservoir temperature,Hydrogeochemistry,Geothermometer,Machine learning
AI 理解论文
溯源树
样例
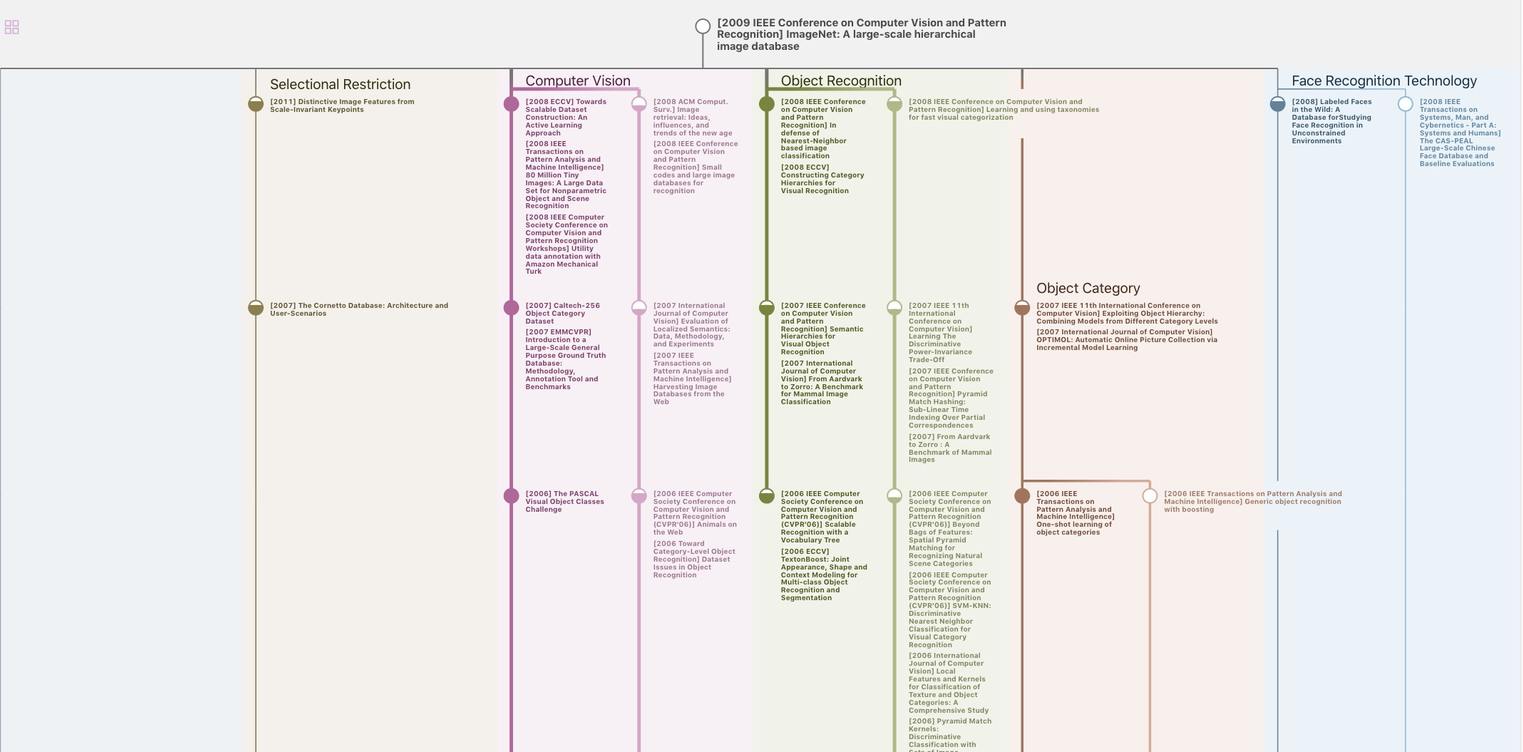
生成溯源树,研究论文发展脉络
Chat Paper
正在生成论文摘要