DSRL: A low-resolution stellar spectral of LAMOST automatic classification method based on discrete wavelet transform and deep learning methods
Experimental Astronomy(2024)
摘要
Automatic classification of stellar spectra contributes to the study of the structure and evolution of the Milky Way and star formation. Currently available methods exhibit unsatisfactory spectral classification accuracy. This study investigates a method called DSRL, which is primarily used for automated and accurate classification of LAMOST stellar spectra based on MK classification criteria. The method utilizes discrete wavelet transform to decompose the spectra into high-frequency and low-frequency information, and combines residual networks and long short-term memory networks to extract both high-frequency and low-frequency features. By introducing self-distillation (DSRL-1, DSRL-2, and DSRL-3), the classification accuracy is improved. DSRL-3 demonstrates superior performance across multiple metrics compared to existing methods. In both three-class(F ,G ,K) and ten-class(A0, A5, F0, F5, G0, G5, K0, K5, M0, M5) experiments, DSRL-3 achieves impressive accuracy, precision, recall, and F1-Score results. Specifically, the accuracy performance reaches 94.50
更多查看译文
关键词
LAMOST,Spectral classification of stars,DWT,ResNet,LSTM,Self-distillation,Z-Score standardization
AI 理解论文
溯源树
样例
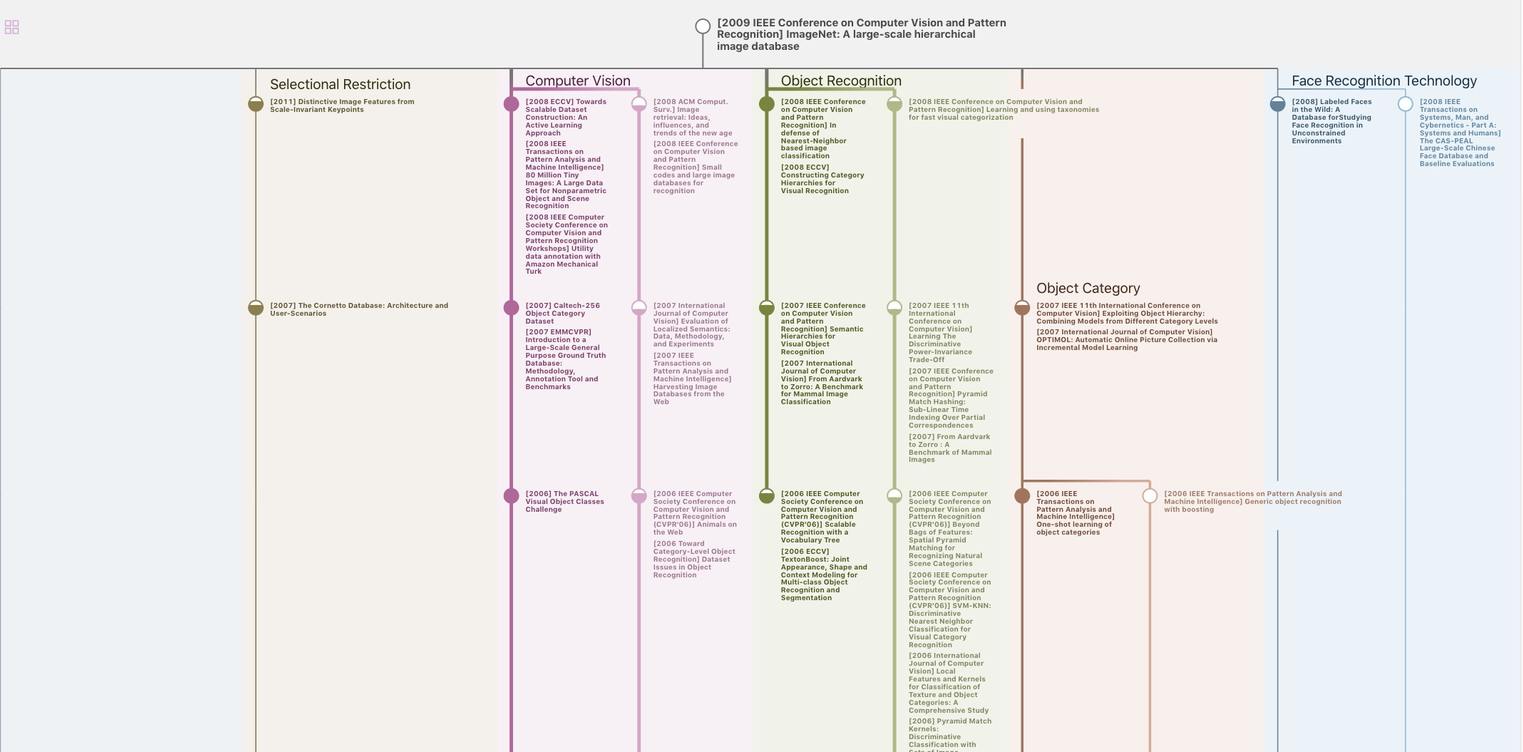
生成溯源树,研究论文发展脉络
Chat Paper
正在生成论文摘要