Closing the Gap: Achieving Global Convergence (Last Iterate) of Actor-Critic under Markovian Sampling with Neural Network Parametrization
arxiv(2024)
摘要
The current state-of-the-art theoretical analysis of Actor-Critic (AC)
algorithms significantly lags in addressing the practical aspects of AC
implementations. This crucial gap needs bridging to bring the analysis in line
with practical implementations of AC. To address this, we advocate for
considering the MMCLG criteria: Multi-layer neural network
parametrization for actor/critic, Markovian sampling,
Continuous state-action spaces, the performance of the Last
iterate, and Global optimality. These aspects are practically
significant and have been largely overlooked in existing theoretical analyses
of AC algorithms. In this work, we address these gaps by providing the first
comprehensive theoretical analysis of AC algorithms that encompasses all five
crucial practical aspects (covers MMCLG criteria). We establish global
convergence sample complexity bounds of
𝒪̃(ϵ^-3). We achieve this result
through our novel use of the weak gradient domination property of MDP's and our
unique analysis of the error in critic estimation.
更多查看译文
AI 理解论文
溯源树
样例
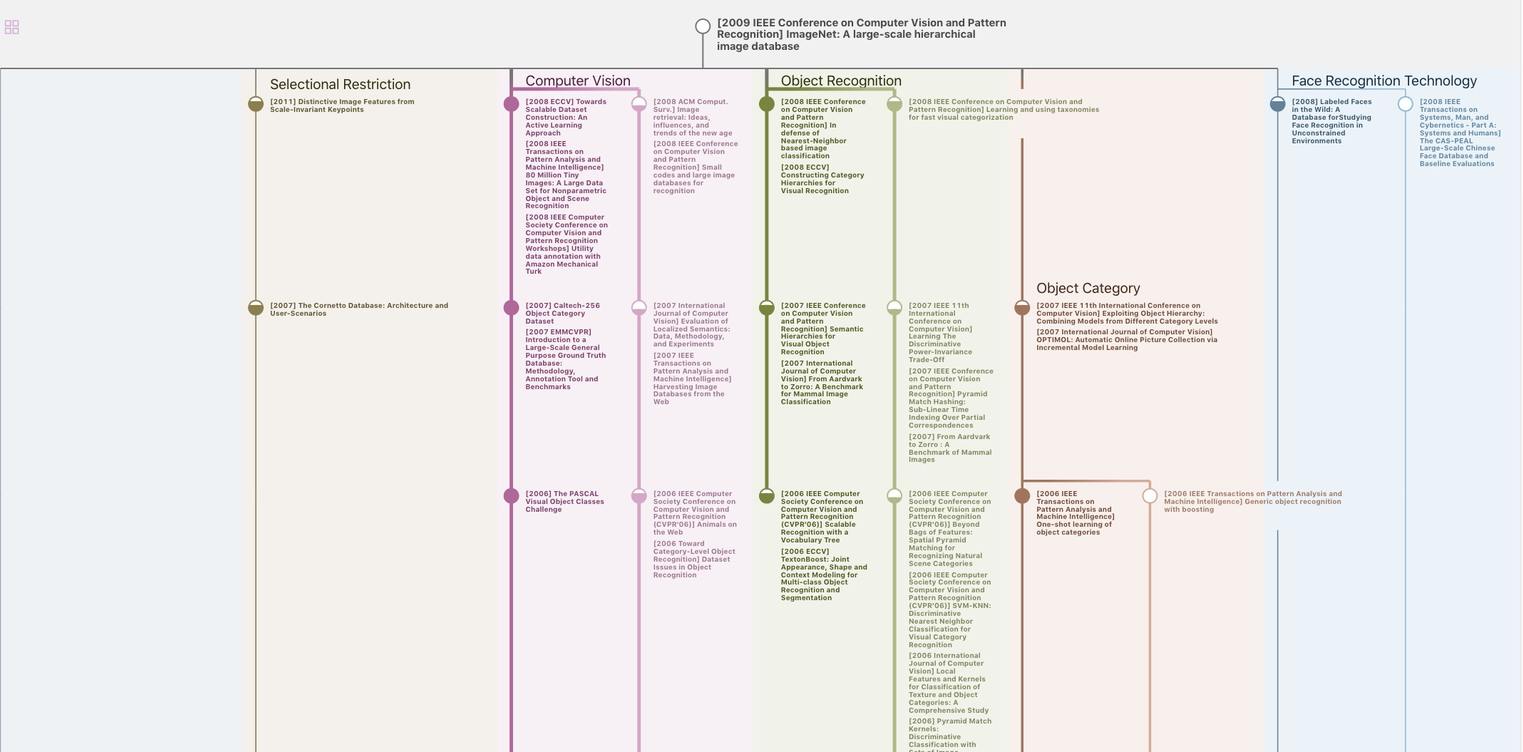
生成溯源树,研究论文发展脉络
Chat Paper
正在生成论文摘要