230 Using phenotypes with uncertain identification leveraging computer vision models
Journal of Animal Science(2024)
摘要
Abstract There has been an increase in high throughput phenotyping using sensors in livestock farming. The major obstacle when analyzing phenotypic data in studies using cameras is identifying the animals using computer vision. To overcome this problem, an analysis method that uses keypoint detection in conjunction with classification algorithms can identify animals in images. However, these algorithms generally output a prediction probability for different classes, reflecting uncertainty. In most practices, the highest probability will be assigned as the putative identification (ID). In some cases, this may introduce incorrect identification and affect downstream decisions. Here, we propose an analytical approach to incorporate all the uncertainty in the putative ID distribution into downstream inferences. We used a publicly available keypoint detection dataset of 17 keypoints detected in 3,226 images from 30 diverse thoroughbred horses. We performed feature engineering with Linear Discriminant Analysis (LDA) using 5-fold cross-validation to build horse classifiers. The best model selected had an accuracy of 88.12% to identify animals in the testing set. Afterward, we performed Monte Carlo simulations to add a phenotype that was assumed independent of the image keypoints. This generated a set of plasmodes to evaluate methods that accounted for ID uncertainty. Each simulation included different means for two groups of 15 horses. In total nine simulation scenarios were considered including varying effect sizes of group mean difference and various repeatability values (horse variance to total variance ratio). We analyzed the simulated data with three different mixed models: 1) Fixed and random effects were based on using ground truth assignment of ID, 2) Fixed and random effects were based on using the putative ID with the highest posterior probability from the best classifier, 3) Finally, prediction probabilities were used to account for putative ID uncertainty. As expected, the model that used ground truth ID had the highest power to detect group differences, and it had the lowest root mean square error (rMSE) to estimate variance components across all scenarios. The linear model that used highest posterior probability putative ID, showed the lowest power and highest rMSE. For instance, as shown in Table 1, the mean rMSE value for group mean differences in the model using ground truth is 0.01 and the model using highest posterior probabilities is 0.13. The model that incorporated ID uncertainty had intermediate properties, showing a rMSE of 0.03 for group differences. Overall, incorporating uncertainty in Animal ID results in improved performance in ultimate test statistics used in animal studies. This is very important in breeding and precision livestock farming contexts where animal identification is challenging. We will further investigate this.
更多查看译文
AI 理解论文
溯源树
样例
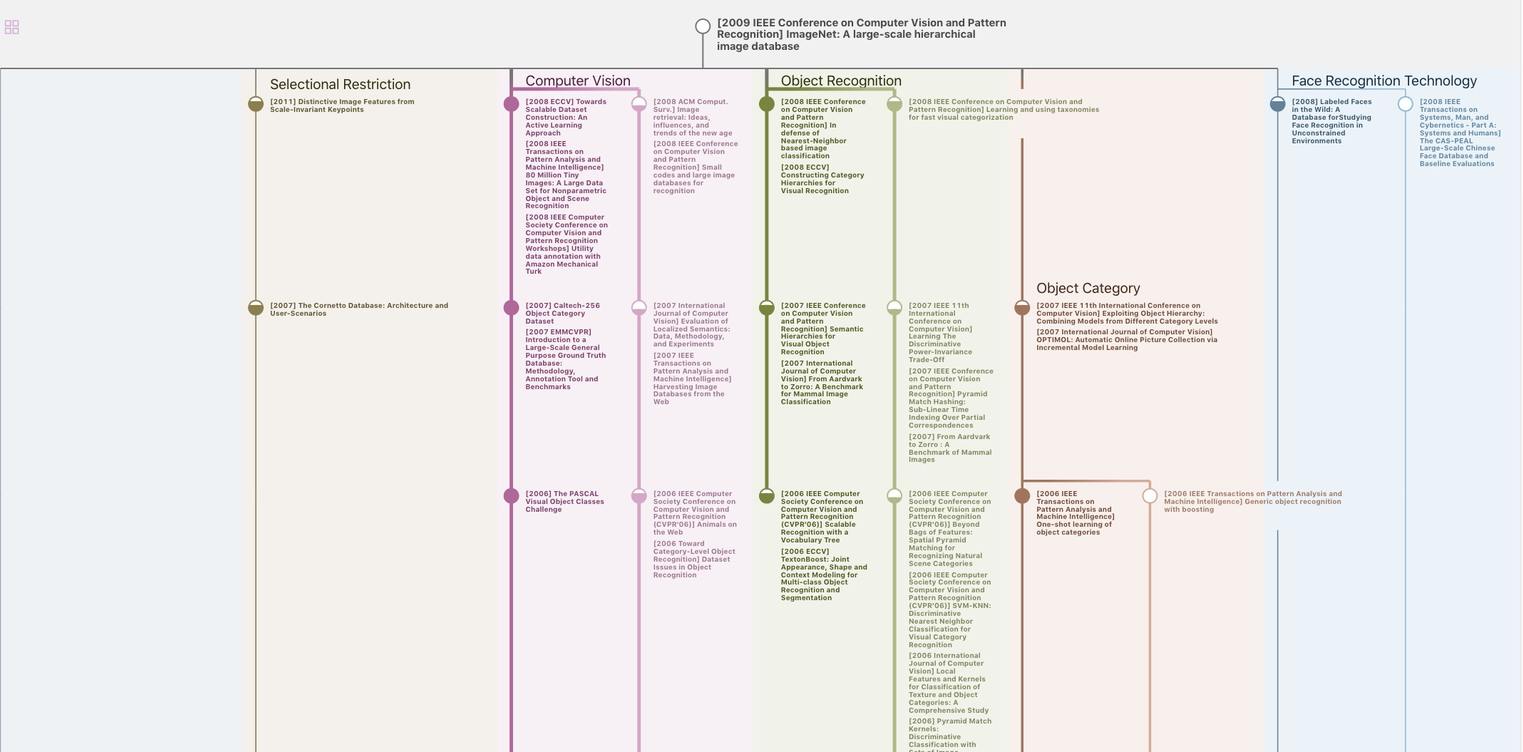
生成溯源树,研究论文发展脉络
Chat Paper
正在生成论文摘要