Deep learning prediction of yields of fluid catalytic cracking via differential evolutionary dual-stage attention-based LSTM
Fuel(2024)
摘要
Fluid catalytic cracking (FCC) was one of the major technologies for converting low-quality heavy oil into valuable light oil in the refining industry. The prediction of FCC yields was of great significance to the online optimization of yield distribution and quality control. In this work, the deep learning with differential evolutionary dual-stage attention-based long short-term memory (LSTM) method was proposed for FCC yields prediction. The first layer of attention mechanism was introduced to extract information from process parameters, feed properties, and clustering of catalyst data, and the second layer of attention mechanism adaptively evaluated the weights for process features of different time-step. The adaptive differential evolution algorithm based on phased mutation strategy was used to optimize the weights to avoid the algorithm falling into local optimum. The FCC yields were predicted by five LSTM-based models under scenarios of clustering and non-clustering of catalyst data. The results indicate that the root-mean-square errors (RMSEs) of FCC yields prediction via clustering method were reduced by 1.5–95.8% compared with non-clustering. The proposed method outperformed all other models, of which the RMSEs of dry gas, liquefied petroleum gas (LPG), gasoline, and diesel yields prediction in clustering scenarios were 0.10761, 0.00582, 0.40749, and 0.00670, respectively. Compared with the conventional machine learning, basic LSTM, and dual-stage attention-based LSTM models, the RMSEs of proposed model decreased by 11.3–94.6%, 1.9–94.6%, and 5.1%–96.6%, respectively.
更多查看译文
关键词
Deep learning,LSTM,Attention mechanism,Differential evolution,FCC
AI 理解论文
溯源树
样例
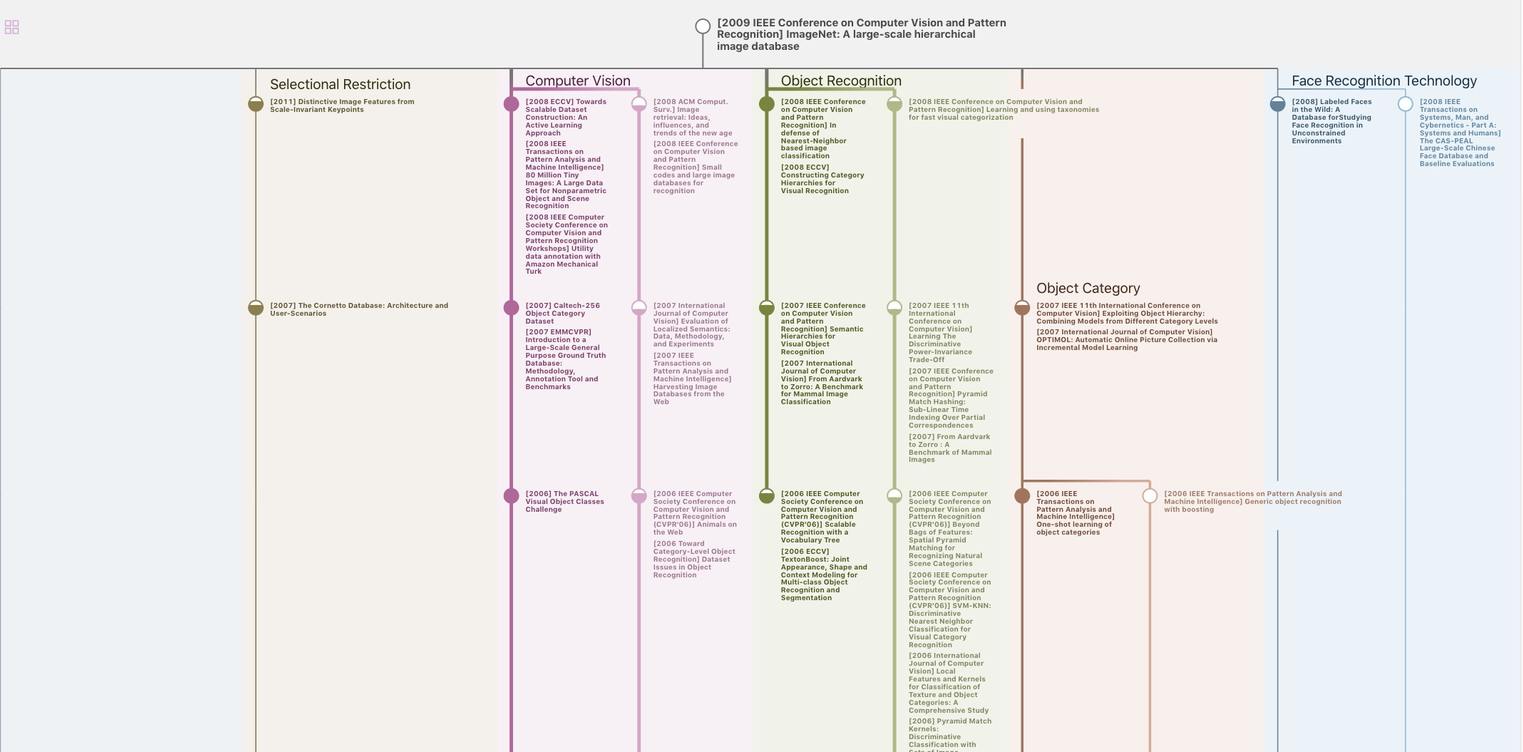
生成溯源树,研究论文发展脉络
Chat Paper
正在生成论文摘要