A sequence decomposition genetic algorithm for petrophysical inversion with spatial correlation
GEOPHYSICS(2024)
摘要
Predicting petrophysical properties based on seismic attributes is essential for accurate subsurface characterization. Typically, a forward model is first established to relate petrophysical properties to geophysical measurements, and then the petrophysical properties are inverted from actual measurements. Imposing prior constraints of spatial relationship is also desirable to regularize the ill-posed inverse problem. However, petrophysical inversion involving spatial correlation presents a high-dimensional optimization problem with multiple local extrema. This problem can be challenging to solve, particularly when discrete variables (such as lithofacies) and continuous variables (such as porosity and fluid saturation) are considered together. Therefore, we propose a global optimization algorithm that utilizes Markov chain decomposition and a genetic algorithm for joint discrete-continuous petrophysical inversion with spatial correlation. The innovation of this algorithm is to spatially decouple the sequence optimization problem into multiple low-dimensional sub-problems and overcome the initial value dependence to converge to the global optimal solution. Furthermore, an approximation operation is introduced to expedite the optimization algorithm. Numerical experiments demonstrate that after algorithmic acceleration, the spatially correlated inversion requires the same computational time as a simple spatially uncorrelated inversion and achieves significantly better accuracy than the latter. Finally, we apply the method to a case study in East China, where lithofacies, clay content, porosity, and water saturation are inferred from the data of wave velocities and density. The inversion results around the wells generally match the well logging data, verifying the feasibility of the new method.
更多查看译文
AI 理解论文
溯源树
样例
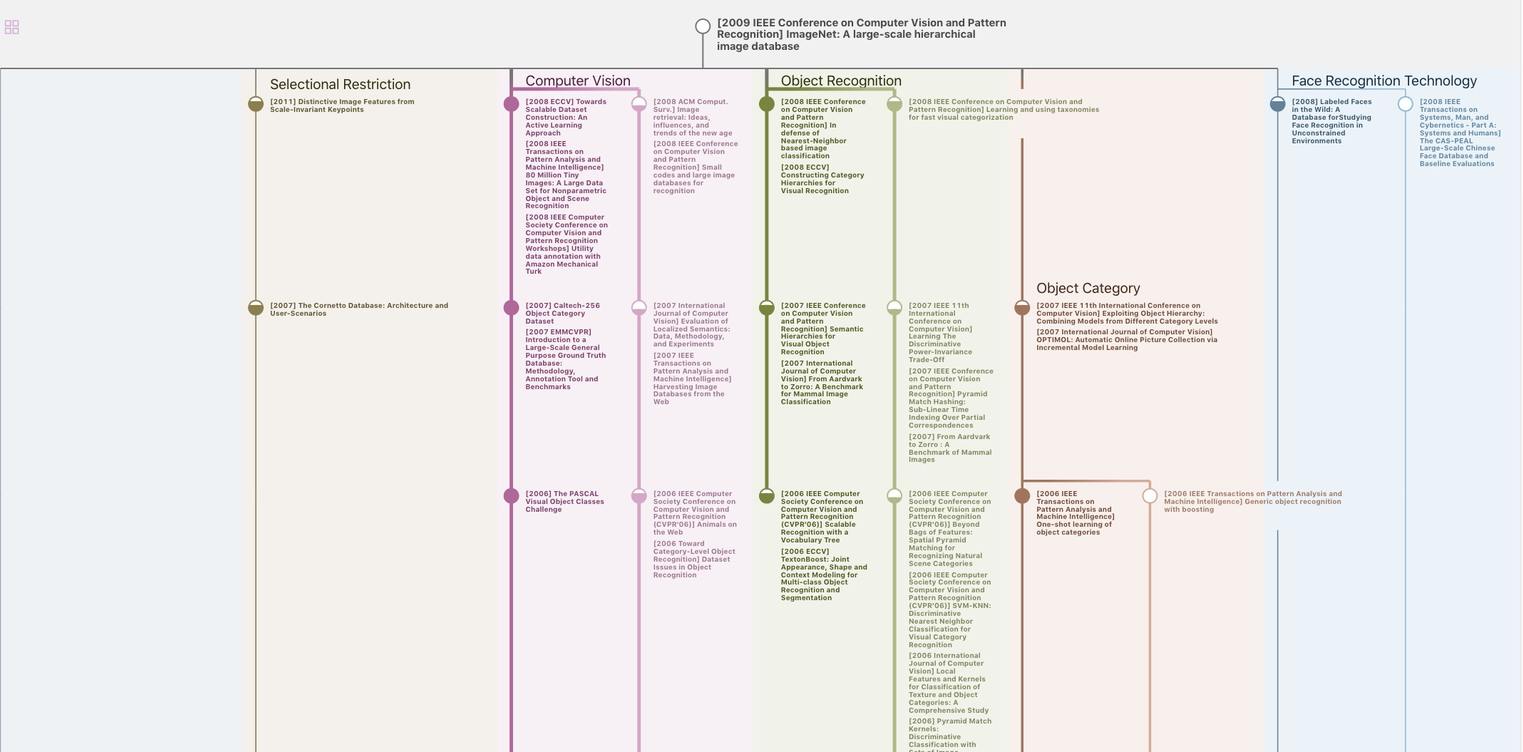
生成溯源树,研究论文发展脉络
Chat Paper
正在生成论文摘要