mRNA-CLA: An Interpretable Deep Learning Approach for Predicting mRNA Subcellular Localization
Methods(2024)
摘要
Messenger RNA (mRNA) is vital for post-transcriptional gene regulation, acting as the direct template for protein synthesis. However, the methods available for predicting mRNA subcellular localization need to be improved and enhanced. Notably, few existing algorithms can annotate mRNA sequences with multiple localizations. In this work, we propose the mRNA-CLA, an innovative multi-label subcellular localization prediction framework for mRNA, leveraging a deep learning approach with a multi-head self-attention mechanism. The framework employs a multi-scale convolutional layer to extract sequence features across different regions and uses a self-attention mechanism explicitly designed for each sequence. Paired with Position Weight Matrices (PWMs) derived from the convolutional neural network layers, our model offers interpretability in the analysis. In particular, we perform a base-level analysis of mRNA sequences from diverse subcellular localizations to determine the nucleotide specificity corresponding to each site. Our evaluations demonstrate that the mRNA-CLA model substantially outperforms existing methods and tools.
更多查看译文
关键词
Subcellular localization,Self-attention weights,Model interpretability,Sequence analysis
AI 理解论文
溯源树
样例
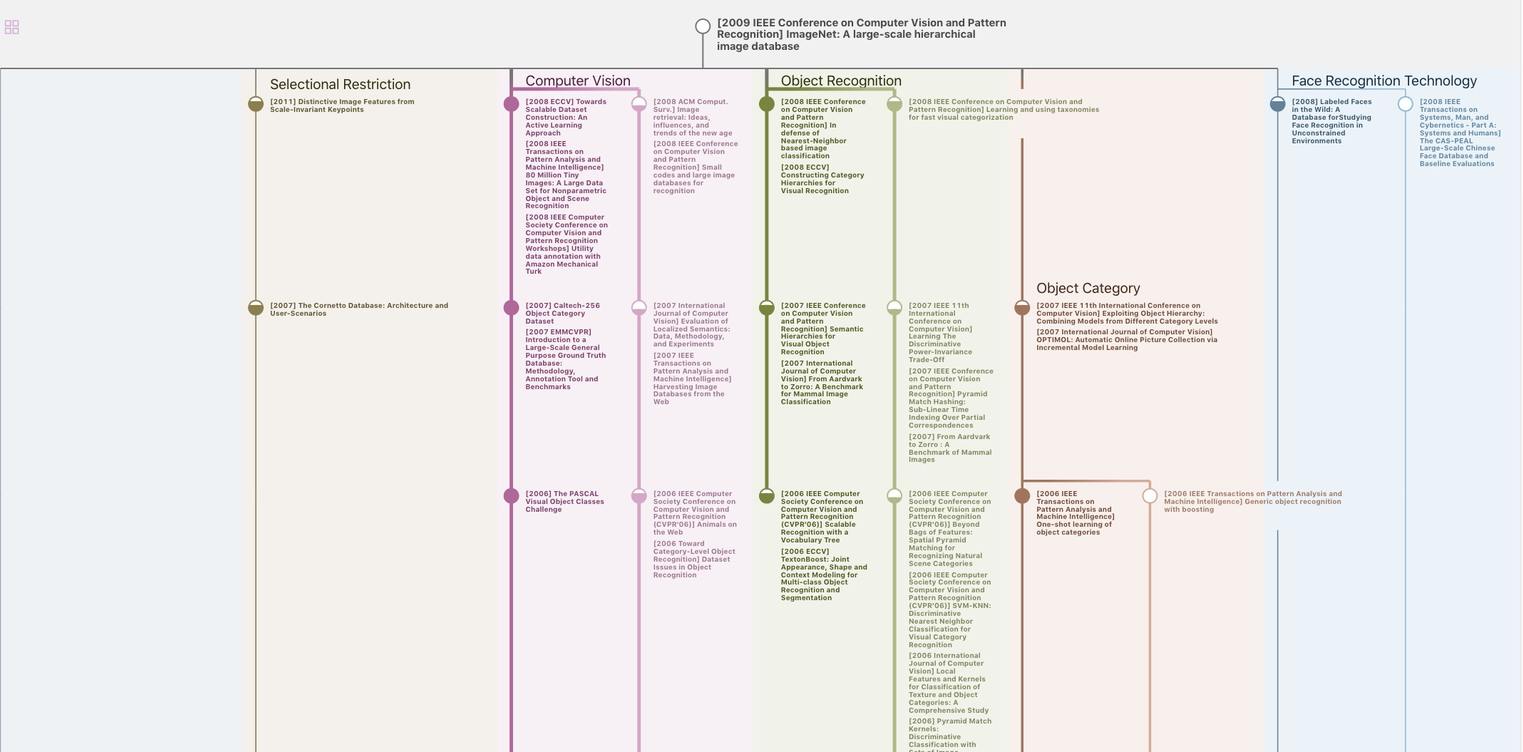
生成溯源树,研究论文发展脉络
Chat Paper
正在生成论文摘要