Remaining Useful Life Prediction via Frequency Emphasizing Mix-Up and Masked Reconstruction
IEEE Transactions on Artificial Intelligence(2024)
摘要
The prediction of the Remaining Useful Lifetime (RUL) of machines and tools is crucial in the realm of modern manufacturing and in the framework of Industry 4.0. Recent deep learning techniques offer an opportunity for the utilization of data-driven methods in RUL prediction. However, the persistent data scarcity issue presents a thorny bottleneck in the machinery RUL prediction tasks due to the high cost of labeled training data collection. In this paper, we propose an effective learning framework for RUL prediction consisting of two novel methodological modules to improve data utilization efficiency. The first module is to leverage the intrinsicallydecomposed frequency-domain information to boost the effective feature extraction with a novel-designed
Frequency Emphasizing Mix-up Module (FEMM)
. The second one involves incorporating semi-supervised learning to make use of unrestricted domain unlabeled data to overcome the constraints associated with data scarcity where we introduced
Masked Autoencoder Reconstruction Auxiliary Learning (MARAL)
to the model. In addition, to better obtain temporal information, an LSTM temporal projection layer is designed. The proposed method was evaluated through experiments conducted on the C-MAPSS datasets, and our results show that the proposed method outperforms existing other methods in terms of both accuracy and effectiveness in the RUL prediction. In addition, our experimental results demonstrate that the proposed method is able to leverage unrestricted domain datasets to significantly boost model performance under low-data scenarios.
更多查看译文
关键词
transformer,feature fusion,long short-term memory (LSTM),semi-supervised learning (SSL),machinery remaining useful lifetime (RUL) prediction,prognostics,and health management (PHM)
AI 理解论文
溯源树
样例
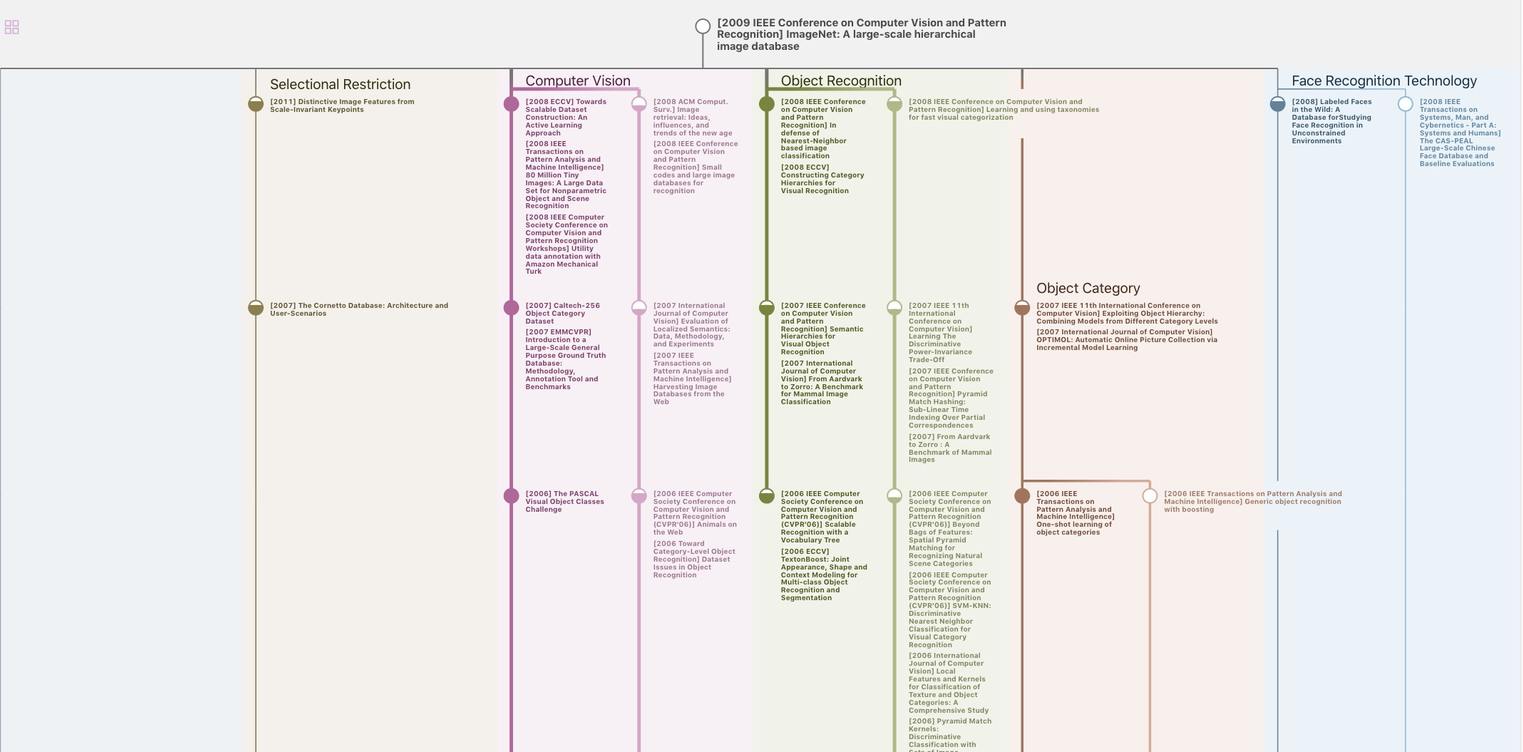
生成溯源树,研究论文发展脉络
Chat Paper
正在生成论文摘要