A machine learning model to predict surgical site infection after surgery of lower extremity fractures
International Orthopaedics(2024)
摘要
This study aimed to develop machine learning algorithms for identifying predictive factors associated with the risk of postoperative surgical site infection in patients with lower extremity fractures. A machine learning analysis was conducted on a dataset comprising 1,579 patients who underwent surgical fixation for lower extremity fractures to create a predictive model for risk stratification of postoperative surgical site infection. We evaluated different clinical and demographic variables to train four machine learning models (neural networks, boosted generalised linear model, naïve bayes, and penalised discriminant analysis). Performance was measured by the area under the curve score, Youdon’s index and Brier score. A multivariate adaptive regression splines (MARS) was used to optimise predictor selection. The final model consisted of five predictors. (1) Operating room time, (2) ankle region, (3) open injury, (4) body mass index, and (5) age. The best-performing machine learning algorithm demonstrated a promising predictive performance, with an area under the ROC curve, Youdon’s index, and Brier score of 77.8
更多查看译文
关键词
Machine learning,Postoperative infection,Risk score,Lower extremity
AI 理解论文
溯源树
样例
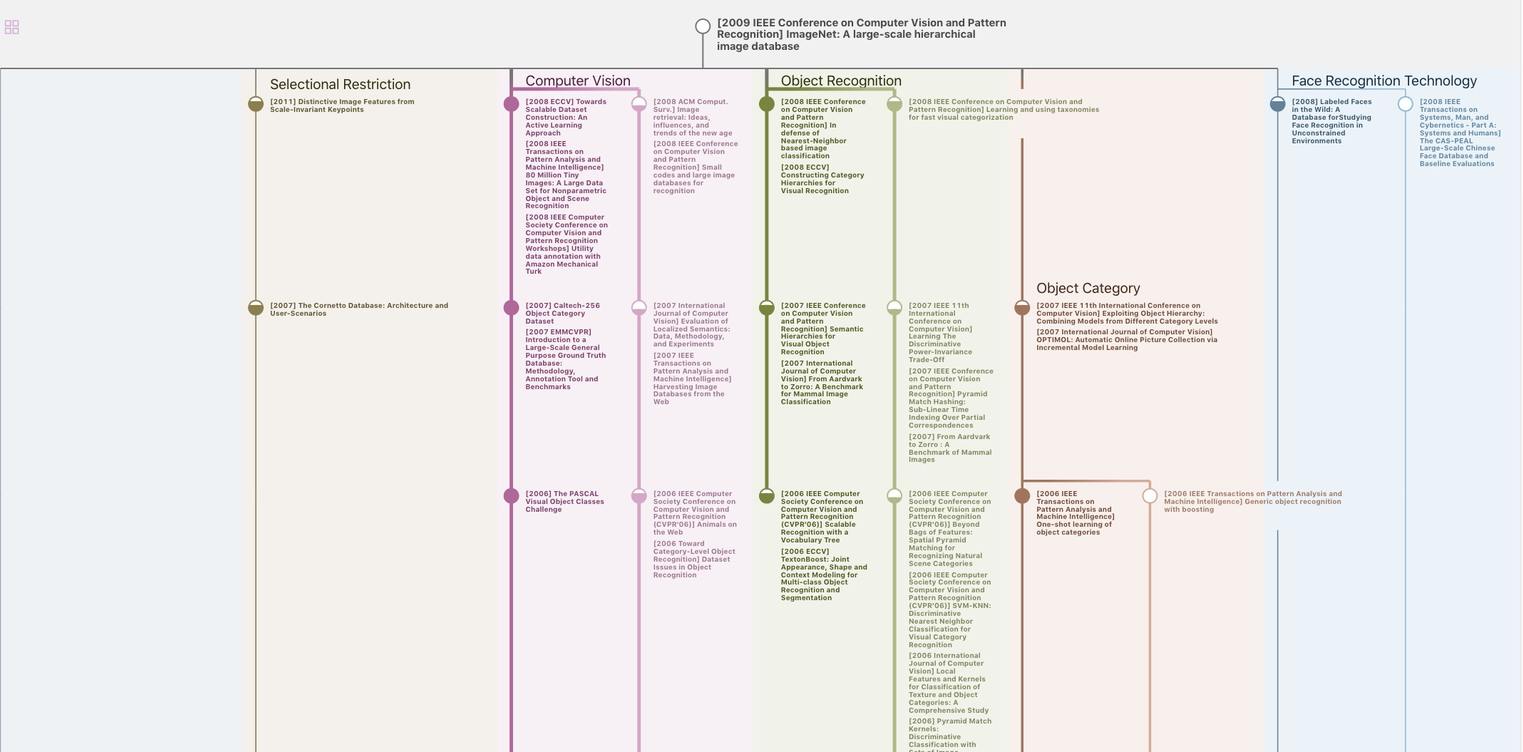
生成溯源树,研究论文发展脉络
Chat Paper
正在生成论文摘要