LSTM RNN-based excitation force prediction for the real-time control of wave energy converters
Ocean Engineering(2024)
摘要
Wave energy is a type of abundant and dense renewable energy. Wave force prediction is a critical technology that influences power absorption efficiency in the real-time control of wave energy converter (WEC). Could wave elevation be used to predict wave excitation force directly by training artificial neural network? This method results in rapid and suitable prediction for real-time control. A long short-term memory recurrent neural network (LSTM RNN) algorithm is introduced to identify characteristics of wave excitation forces based on wave elevations. In this method, the wave elevations in front of the structure are measured to obtain sufficient time to actuate the control manipulation. A total of 180 regular wave and 12 irregular wave tests are conducted, and the LSTM RNN model is trained based on the experimental results. The performance of the LSTM algorithm is verified. According to the regular cases in the study, the LSTM prediction can identify high-order harmonic loads, and the anti-noise capability of the LSTM algorithm can filter random noises from the measure signals. In the irregular cases, the LSTM RNN algorithm performs effectively to predict the wave force excited on the structure using wave elevations measured by wave probes. The best combinations of the test setting parameters are determined to guide experimental tests and WEC prototypes.
更多查看译文
关键词
Wave prediction,Wave energy converter,Machine learning,LSTM RNN,Real-time control,Experimental test
AI 理解论文
溯源树
样例
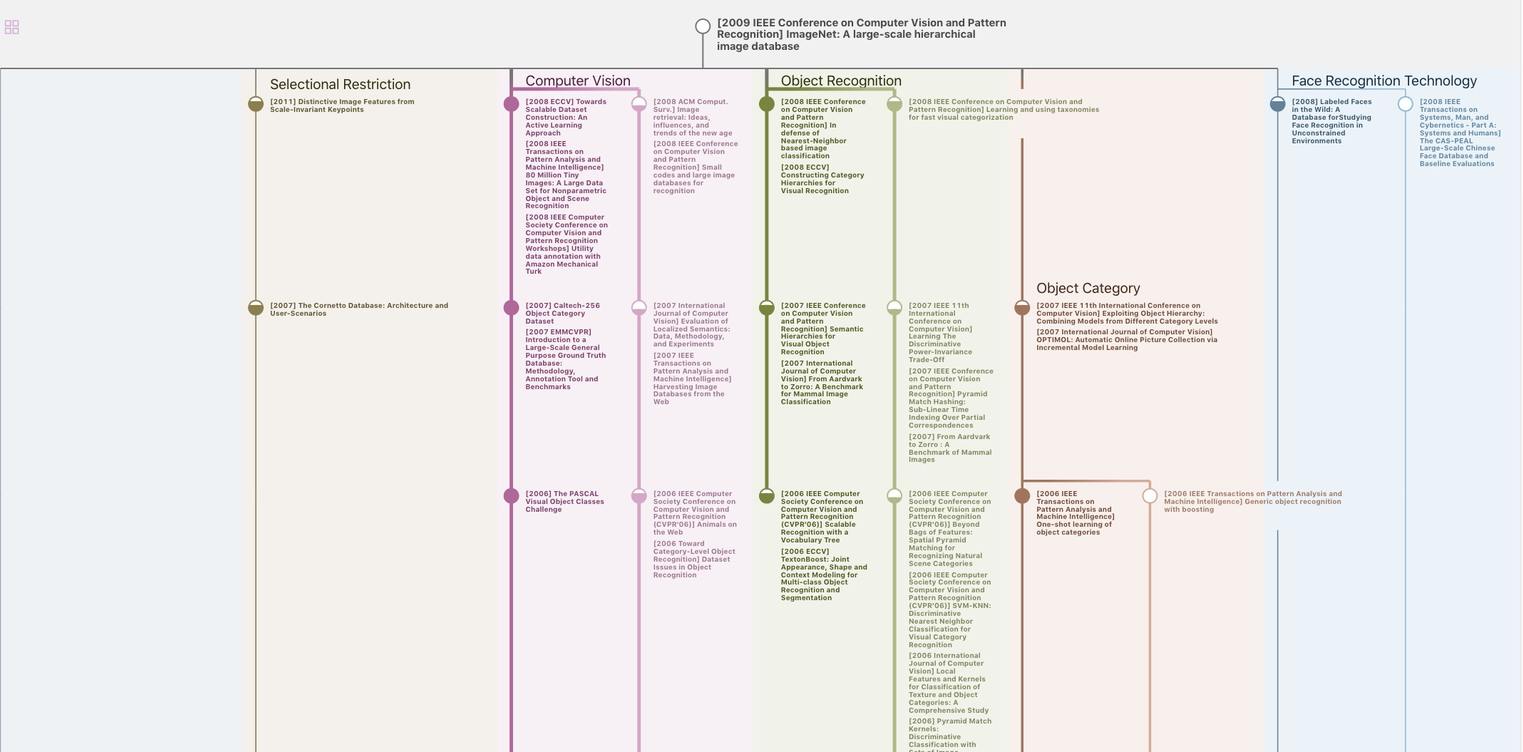
生成溯源树,研究论文发展脉络
Chat Paper
正在生成论文摘要