Augment Single-cell RNA-seq data with Surface Protein Levels using Gene set-based Deep Learning and Transfer Learning Methods
crossref(2024)
摘要
As scRNA-seq becomes increasingly accessible, providing a cost-efficient method to augment surface protein levels from gene expression measurements are desirable. We proposed a machine learning approach that includes a novel geneset neural network (GS-NN) that aims to learn robust and biologically meaningful features and a highly efficient transfer learning strategy to address cross-dataset differences. We conducted comprehensive experiments to show the improvements of the proposed methods. Specifically, we demonstrate that GS-NN learns more robust features to achieve better cross-subject performance than other machine learning approaches. Transfer learning further improves that of GS-NN by reducing dataset differences through highly efficient fine-tuning. The unique genesets design of GS-NN also allows identification of functions contributing to the prediction and improvement of the proposed strategy. Overall, this study reports a novel approach to robustly augment.
更多查看译文
AI 理解论文
溯源树
样例
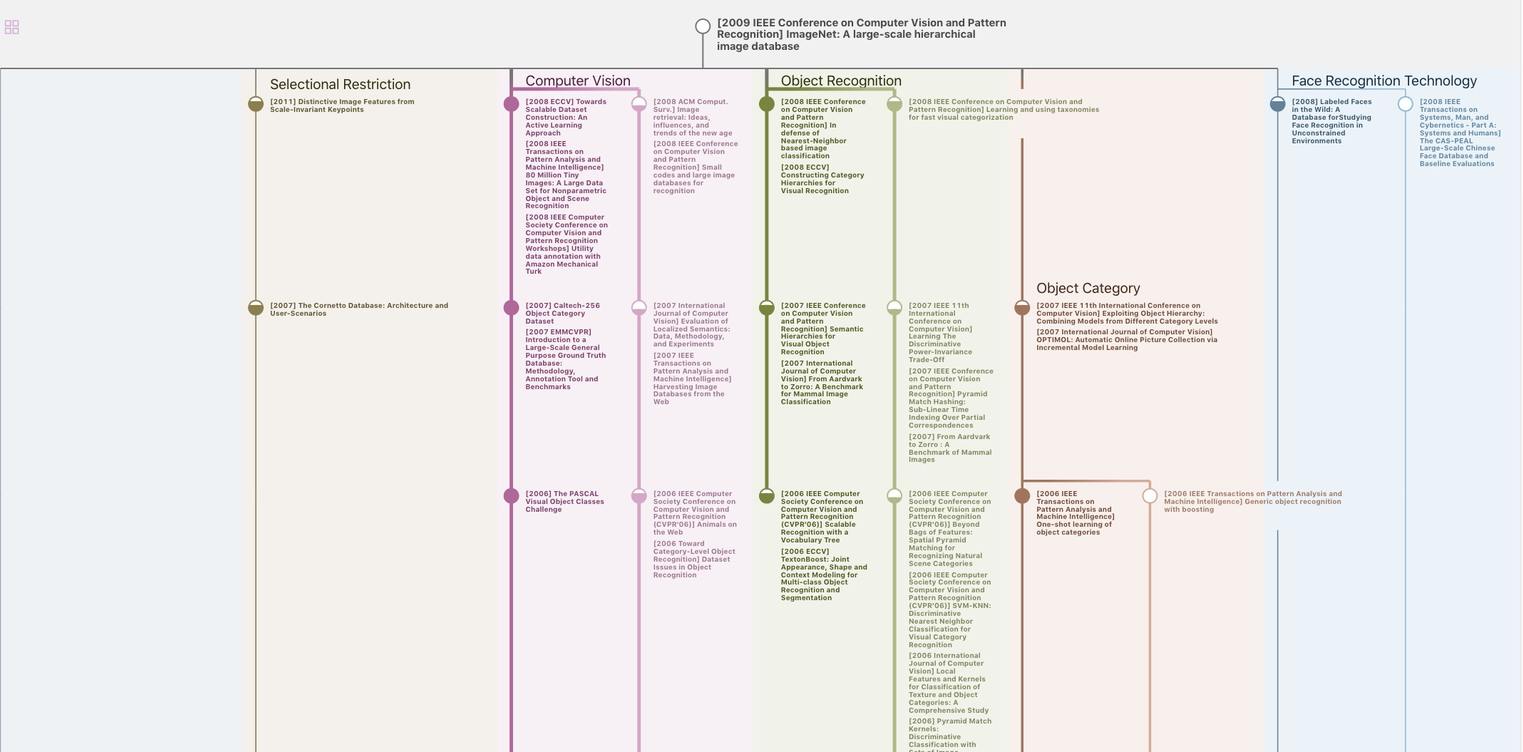
生成溯源树,研究论文发展脉络
Chat Paper
正在生成论文摘要