Infusing internalized knowledge of language models into hybrid prompts for knowledgeable dialogue generation
Knowledge-Based Systems(2024)
摘要
Existing knowledge-grounded dialogue (KGD) systems access the knowledge from an external knowledge base, then generate the context-coherent response accordingly. However, the knowledge access capability is constrained to the scale of a knowledge base. On the one hand, a small-scale knowledge base makes a model hard to generalize on unseen topics, while the improper shift of topics may induce an unsmooth conversation flow. On the other hand, a large-scale knowledge base requires a strong retrieval component to accurately index the context-relevant knowledge from many plausible candidates, costing significant amounts of time and resources. To address this, we regard the language model as a virtual knowledge base and propose homogenizing internalized knowledge of different language models into hybrid prompts. The hybrid prompts are a set of continuous vectors learned to represent knowledge inherently encoded in different language models. Furthermore, we devise a two-stage knowledge-grounding manner, in which both the knowledge internalized in language models and the knowledge provided by evidence can be jointly optimized to generate a knowledgeable response. We compare our proposed method with two groups of methods, including methods with explicit knowledge retrieval and those with implicit knowledge access. Experimental results on three knowledge-grounded dialogue corpora demonstrate advantages over these competitive methods.
更多查看译文
关键词
Knowledge-grounded dialogue,Dialogue response generation,Pre-trained language models,Parameter-efficient fine-tuning
AI 理解论文
溯源树
样例
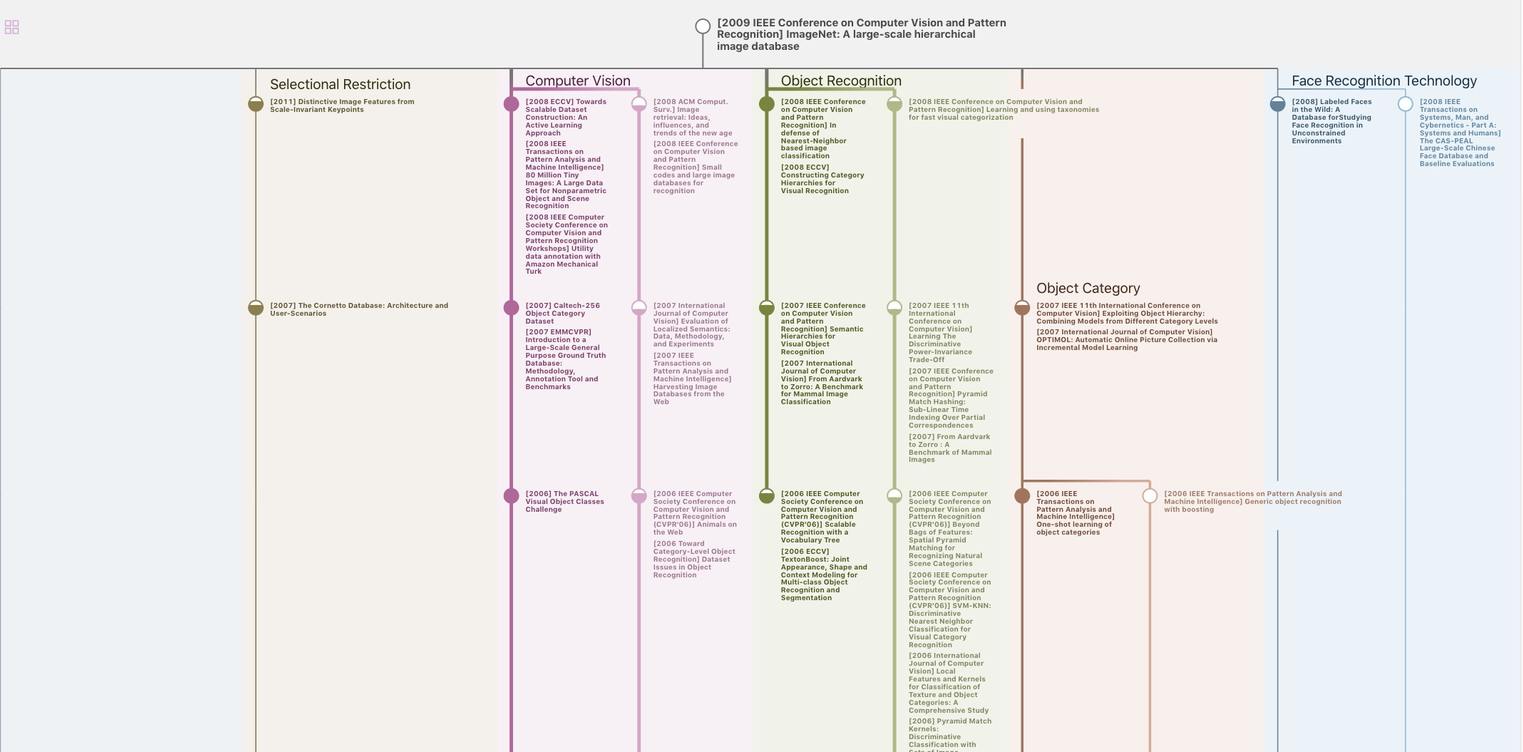
生成溯源树,研究论文发展脉络
Chat Paper
正在生成论文摘要