TB-Net: Intra- and inter-video correlation learning for continuous sign language recognition
Information Fusion(2024)
摘要
Visual feature extraction is the key to continuous sign language recognition (CSLR). However, current CSLR methods based on single-branch networks only rely on intra-video correlation learning to facilitate visual feature optimization. Obviously, this is not conducive to obtaining more robust visual feature representations. Hence, we pioneered a novel CSLR method via intra- and inter-video correlation learning with a two-branch network, named TB-Net. TB-Net explicitly establishes intra-video correlation between glosses and the most relevant video clips at each branch and then introduces inter-video correlation to enhance visual feature extraction at the branch confluence. Specifically, we introduce a contrastive learning-based inter-video correlation module (IEM), which co-optimizes visual features from both branches by designing inter- and intra-video losses to enhance their generalization. In addition, we propose an intra-video correlation module (IAM) based on a gloss-guided attention feature generator to adaptively build mappings between glosses and video clips, which in turn contributes to the acquisition of preliminary gloss-guided visual features within a single video. Extensive experiments on four public CSLR benchmarks show the superior performance of our method.
更多查看译文
关键词
Continuous sign language recognition,Correlation learning,Contrastive learning
AI 理解论文
溯源树
样例
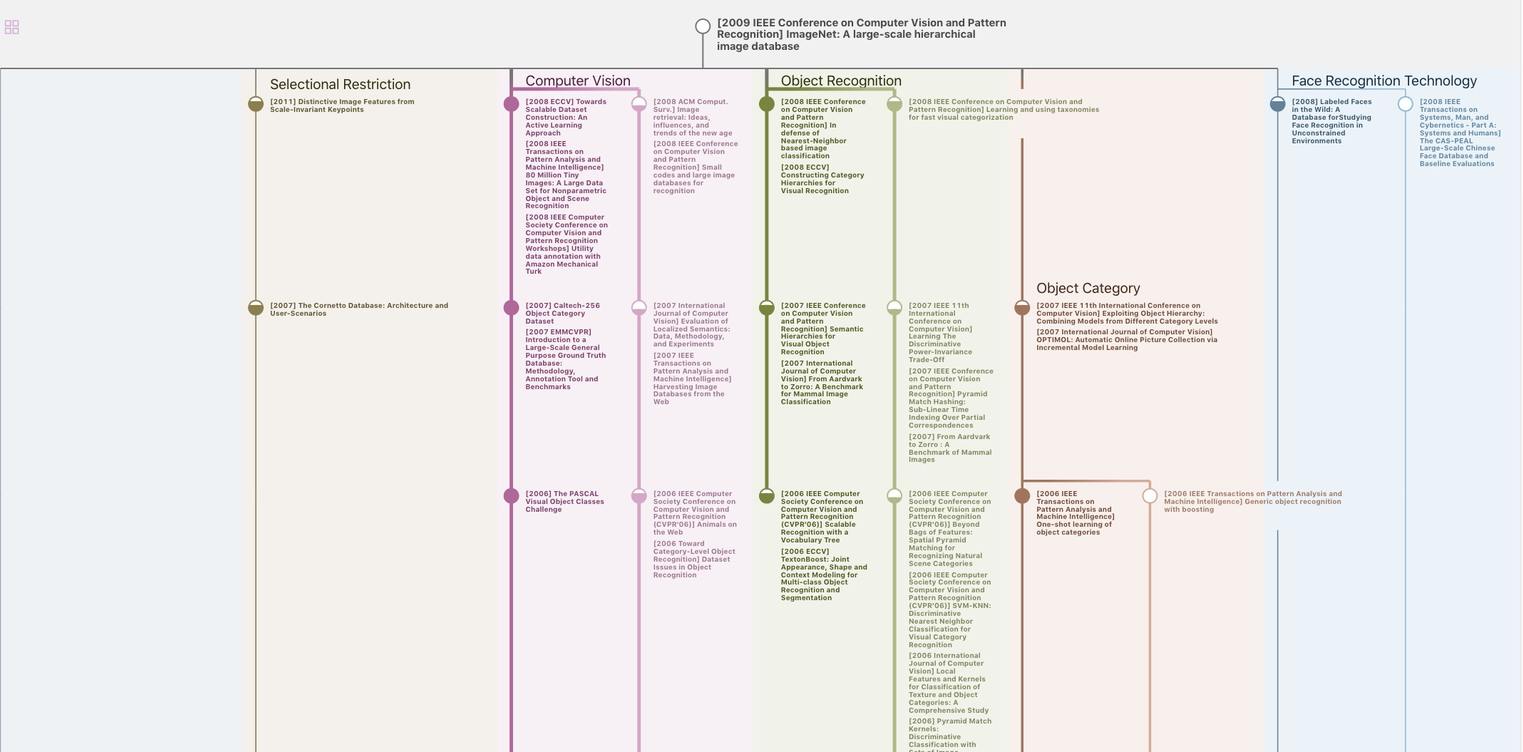
生成溯源树,研究论文发展脉络
Chat Paper
正在生成论文摘要