Gaussian Process-Accelerated Multiobjective Evolutionary Design of Charging Process Considering Multiple User Preferences
IEEE Transactions on Industrial Informatics(2024)
摘要
The charging process design is crucial for optimizing the performance of lithium-ion batteries by identifying protocols that meet diverse demands. The main challenges include: 1) the high costs of battery experiments; 2) the multiple user preferences associated with the demands; and 3) the intricate high-dimensional search space of charging protocols. In light of this, this article presents a Gaussian process-accelerated multiobjective evolutionary design method for effective charging process design. To resolve the first concern, an electrochemical-thermal-aging model is constructed to evaluate charging protocols precisely, substituting the need for expensive battery experiments. Besides, the Gaussian process is applied to accelerate the evaluation process further. Regarding the second issue, a Gaussian process-accelerated two-archive evolutionary algorithm (GPA-TAEA) is developed to efficiently search for a set of optimal charging protocols that satisfy multiple user preferences. To address the third challenge, differential evaluation—an evolutionary algorithm proven effective for large-scale optimization—is employed to enhance the search process. The simulation results demonstrate that: 1) the proposed method effectively reduces the time required for charging process design, yielding a collection of optimal charging protocols that includes 530 solutions within 1000 simulations; 2) compared with five other multiobjective optimization algorithms, GPA-TAEA exhibits superior convergence and diversity; and 3) compared with fixed preference-based methods, GPA-TAEA demonstrates greater efficiency, saving 54% in simulation evaluations and 70% in time when considering seven user preferences.
更多查看译文
关键词
Charging process design,electrochemical-thermal-aging model,Gaussian process (GP),lithium-ion battery,multiobjective evolutionary design
AI 理解论文
溯源树
样例
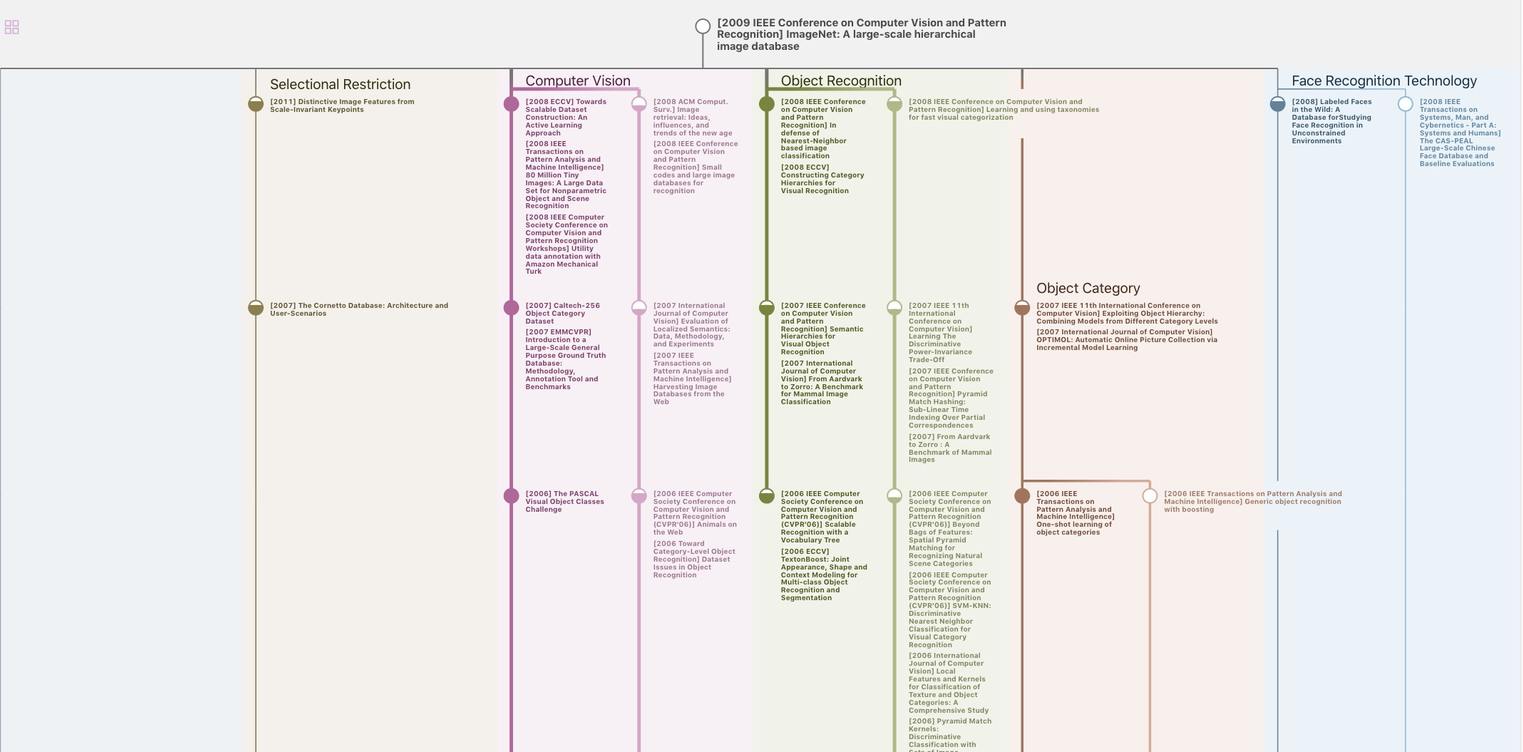
生成溯源树,研究论文发展脉络
Chat Paper
正在生成论文摘要