IntraMix: Intra-Class Mixup Generation for Accurate Labels and Neighbors
arxiv(2024)
摘要
Graph Neural Networks (GNNs) demonstrate excellent performance on graphs,
with their core idea about aggregating neighborhood information and learning
from labels. However, the prevailing challenges in most graph datasets are
twofold of Insufficient High-Quality Labels and Lack of Neighborhoods,
resulting in weak GNNs. Existing data augmentation methods designed to address
these two issues often tackle only one. They may either require extensive
training of generators, rely on overly simplistic strategies, or demand
substantial prior knowledge, leading to suboptimal generalization abilities. To
simultaneously address both of these two challenges, we propose an elegant
method called IntraMix. IntraMix innovatively employs Mixup among low-quality
labeled data of the same class, generating high-quality labeled data at minimal
cost. Additionally, it establishes neighborhoods for the generated data by
connecting them with data from the same class with high confidence, thereby
enriching the neighborhoods of graphs. IntraMix efficiently tackles both
challenges faced by graphs and challenges the prior notion of the limited
effectiveness of Mixup in node classification. IntraMix serves as a universal
framework that can be readily applied to all GNNs. Extensive experiments
demonstrate the effectiveness of IntraMix across various GNNs and datasets.
更多查看译文
AI 理解论文
溯源树
样例
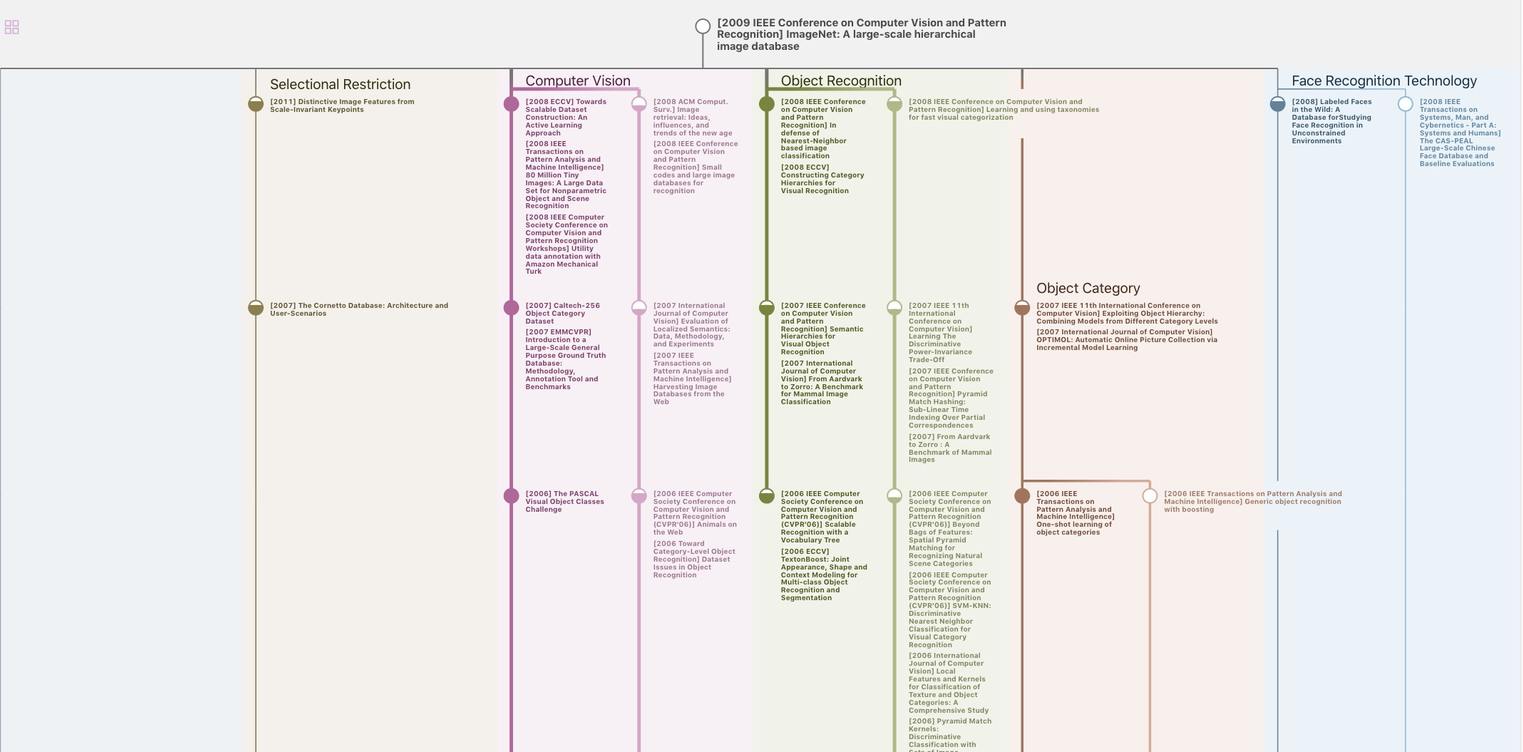
生成溯源树,研究论文发展脉络
Chat Paper
正在生成论文摘要