Abstract PO5-21-03: Cosmetic assessment in the UNICANCER HypoG-01 trial: a deep learning approach
Cancer Research(2024)
摘要
Abstract Introduction Cosmetic evaluation after breast cancer treatment is a clinical indicator of toxicity. User bias and inter-subject variability hamper this objective. To address this limitation, a deep learning approach was developed on the basis of the HYPOG-01 trial (NCT03127995), a phase III trial comparing hypo to normo-fractionated radiotherapy (RT) in breast cancer patients requiring nodal irradiation. Material and Methods Cosmetic outcomes using the Harris scale were assessed by a radiation oncologist using a 4-level rating system from excellent to poor. This evaluation involved photographs from 581 female patients included in the intention-to-treat population of the HYPOG-01 study analysis (mastectomy/pamectomy and non-usable cases excluded). Front images were taken with the arms along the body at baseline, 3-weeks after radiotherapy start, end of treatment, then 6 months and every year after randomization up to 5 years. Comparing manual landmark annotation with the semi-automated software BCCT.core©, the agreement rate was moderate, with an intra-class correlation coefficient (ICC) of 0.66 (95%CI 0.57-0.73). The dataset consisted of 2,348 images, which were divided into exclusive patient-based training (1,661), validation (308), and testing (377) datasets. The distribution of Harris scores in the dataset was highly imbalanced: 7% excellent, 33% good, 45% fair, and 15% poor. Nipple landmarks were used as landmarks to address picture acquisition variations by cropping and resizing images to 224 × 224 resolution. Feature extraction was performed using a Swin-TransformerV2, an advanced attention-based vision model initially trained on ImageNet. Newly integrated fully connected layers categorized the extracted features. The model was trained for 300 epochs, and the highest F1-score model was selected. Asymmetry in texture, marks, and breast geometry played a crucial role in Harris scoring. To improve the model's performance, we generated symmetrical images from the region of interest, averaging them with the original images, and we incorporated the timestamps from image captures as an additional influencing factor. We employed techniques such as contrast modulation, lighting adjustments, and geometric transformations to augment the dataset, introducing additional variations and enhancing the model's generalization and accuracy. Results The performance of our model was evaluated using balanced binary classification, multi-class accuracy, and F1-score. Comparatively, our model performed similarly to BCCT in terms of overall accuracy but demonstrated better performance in separating multiple classes, as indicated in Table 1. In Table 2, we present a confusion matrix that provides insights into the model's performance Conclusion The proposed solution simplifies and accelerates the evaluation process by utilizing only two nipple landmarks, surpassing manual and semi-automated tools. This advancement opens doors for automated, large-scale cosmetic toxicity evaluation. Continuous improvement and validation contribute to its robustness and reinforce its significant impact in assessing cosmetic outcomes after breast cancer treatment. Table. Evaluation of performance on the test set (only on cases with evaluation by BCCT (327 images)). Table. Confusion matrix between our predictions and the labels on the test set (only on cases with evaluation by BCCT (327 images)). Citation Format: Alexandre Cafaro, Amandine Ruffier, Gabriele Bielinyte, Youlia Kirova, Séverine Racadot, Mohamed Benchalal, Jean-Baptiste Clavier, Claire Charra-Brunaud, Marie-Eve Chand-Fouche, Delphine Argo-Leignel, Karine Peignaux, Ahmed Benyoucef, David Pasquier, Philippe Guilbert, Julien Blanchecotte, Agnès Tallet, Adeline Petit, Guillemette Bernadou, Xavier Zasadny, Claire Lemanski, Jacques Fourquet, Emmanuelle Malaurie, Honorine Kouto, Carole Massabeau, Alexandre Henni, Pauline Regnault, Aurélie Belliere, Yazid Belkacemi, Magali Le Blanc-Onfroy, Julien Geffrelot, Jean-Briac Prevost, Eleni Karamouza, Stefan Michiels, Marie Bergeaud, Assia Lamrani-Ghaouti, Sami Romdhani, Alexis Bombezin-Domino, Nikos Paragios, Sofia Rivera. Cosmetic assessment in the UNICANCER HypoG-01 trial: a deep learning approach [abstract]. In: Proceedings of the 2023 San Antonio Breast Cancer Symposium; 2023 Dec 5-9; San Antonio, TX. Philadelphia (PA): AACR; Cancer Res 2024;84(9 Suppl):Abstract nr PO5-21-03.
更多查看译文
AI 理解论文
溯源树
样例
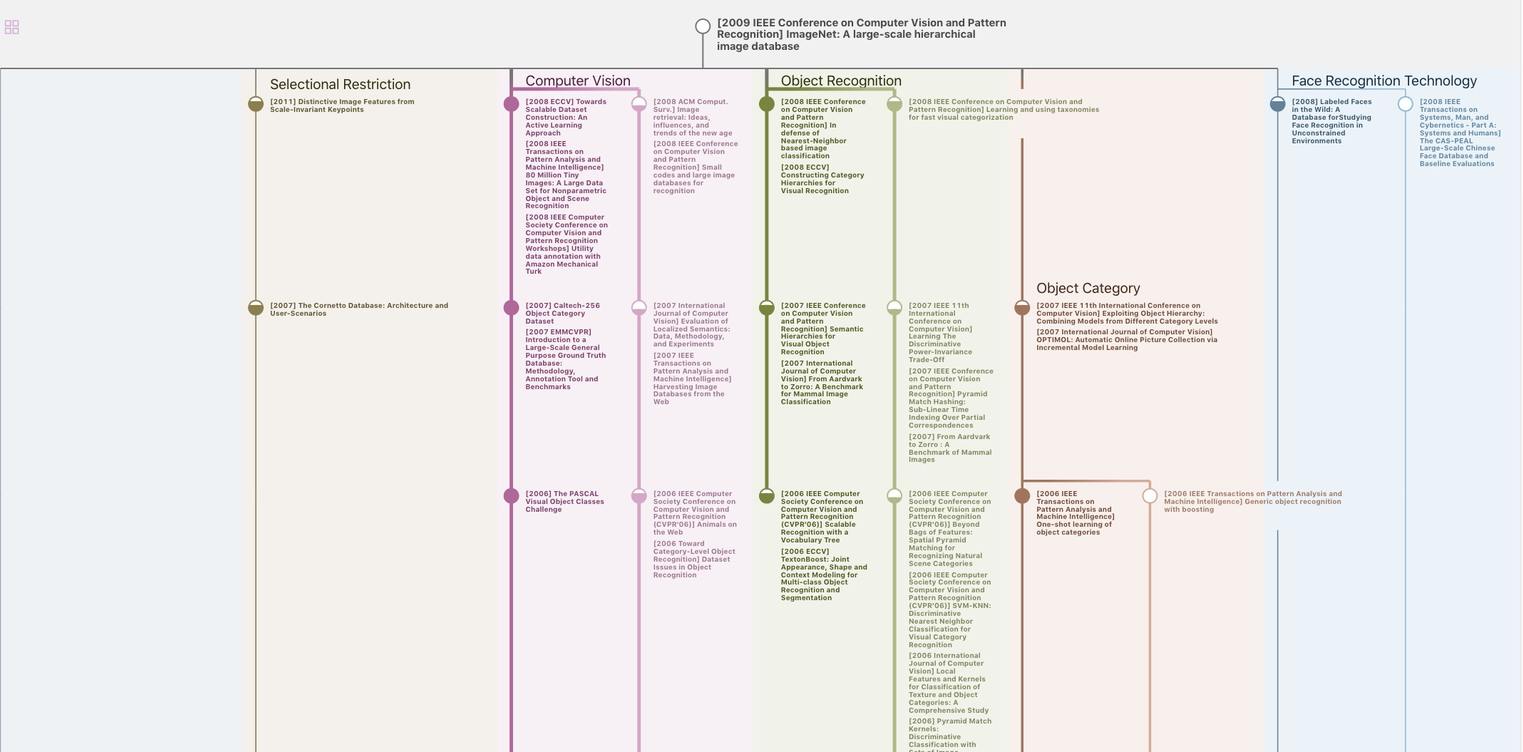
生成溯源树,研究论文发展脉络
Chat Paper
正在生成论文摘要