An advanced AI-based lightweight two-stage underwater structural damage detection model
Advanced Engineering Informatics(2024)
摘要
Images captured in underwater structural inspection often suffer from issues such as blurriness, fogging, distortion, and low resolution due to light absorption and scattering by the water medium and water flows. These problems significantly impact the performance of existing computer-aided underwater structural inspection models, leading to low damage detection rates and inaccurate damage classification. To address these challenges, this paper proposes a super lightweight two-stage damage detection model for underwater structural inspection using deep learning algorithms. The proposed model comprises two stages: Stage I, the Image Fusion Model, and Stage II, the Damage Detection Model. In Stage I, the Image Fusion Model enhances the images captured in underwater structural inspection. It consists of an improved CycleGAN model developed in this study and the Retinex model. In Stage II, a lightweight improved YOLOv5 model serves as the Damage Detection Model. Three types of reinforced concrete (RC) damage: “Concrete Spall (CS)”, “Exposed Rebar (ER)”, and “Concrete Cracking (CC)”, were used to illustrate the detection capabilities of the developed model. The model leverages the strengths of deep learning algorithms to achieve precise damage detection. The proposed model was evaluated by comparing it with existing underwater damage detection models. The results demonstrated significant improvements, including a 27.3% increase in Mean Average Precision (mAP), a 5.0% increase in Precision (P), a 31.6% increase in Recall (R), and a 46.5% decrease in storage requirements. With its advanced capabilities, the proposed model provides the potential to be the AI-assisted computer-aided tool for next-generation underwater structural inspection.
更多查看译文
关键词
Underwater structural inspection,Artificial intelligence,CycleGAN,YOLO series,Computer vision,Damage identification
AI 理解论文
溯源树
样例
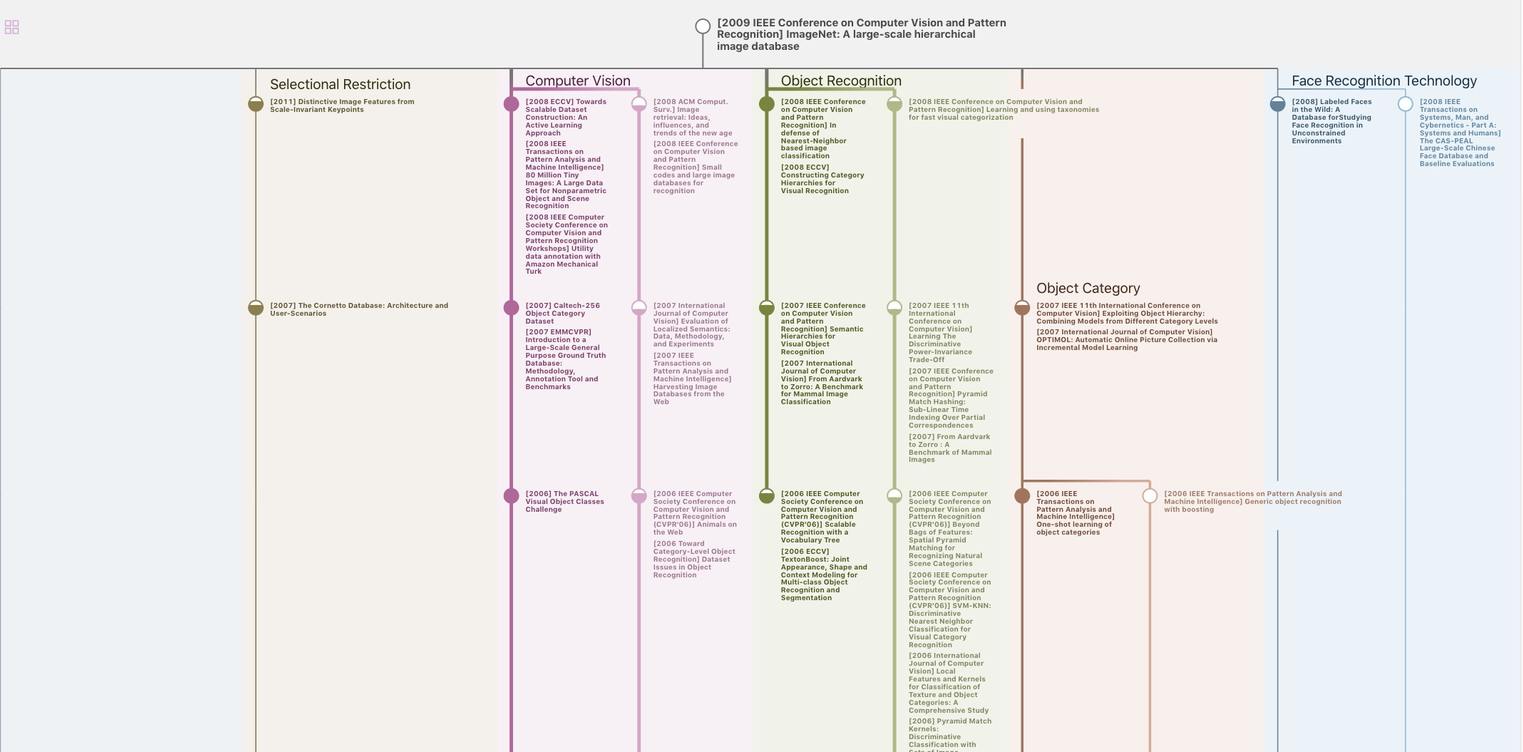
生成溯源树,研究论文发展脉络
Chat Paper
正在生成论文摘要