ECG-surv: A deep learning-based model to predict time to 1-year mortality from 12-lead electrocardiogram
Biomedical Journal(2024)
摘要
Background
Electrocardiogram (ECG) abnormalities have demonstrated potential as prognostic indicators of patient survival. However, the traditional statistical approach is constrained by structured data input, limiting its ability to fully leverage the predictive value of ECG data in prognostic modeling.
Methods
This study aims to introduce and evaluate a deep-learning model to simultaneously handle censored data and unstructured ECG data for survival analysis. We herein introduce a novel deep neural network called ECG-surv, which includes a feature extraction neural network and a time-to-event analysis neural network. The proposed model is specifically designed to predict the time to 1-year mortality by extracting and analyzing unique features from 12-lead ECG data. ECG-surv was evaluated using both an independent test set and an external set, which were collected using different ECG devices.
Results
The performance of ECG-surv surpassed that of the Cox proportional model, which included demographics and ECG waveform parameters, in predicting 1-year all-cause mortality, with a significantly higher concordance index (C-index) in ECG-surv than in the Cox model using both the independent test set (0.860 [95% CI: 0.859- 0.861] vs. 0.796 [95% CI: 0.791- 0.800]) and the external test set (0.813 [95% CI: 0.807- 0.814] vs. 0.764 [95% CI: 0.755- 0.770]). ECG-surv also demonstrated exceptional predictive ability for cardiovascular death (C-index of 0.891 [95% CI: 0.890- 0.893]), outperforming the Framingham risk Cox model (C-index of 0.734 [95% CI: 0.715-0.752]).
Conclusion
ECG-surv effectively utilized unstructured ECG data in a survival analysis. It outperformed traditional statistical approaches in predicting 1-year all-cause mortality and cardiovascular death, which makes it a valuable tool for predicting patient survival.
更多查看译文
关键词
Survival prediction,Electrocardiography,Censored data,Deep neural network,Deep learning
AI 理解论文
溯源树
样例
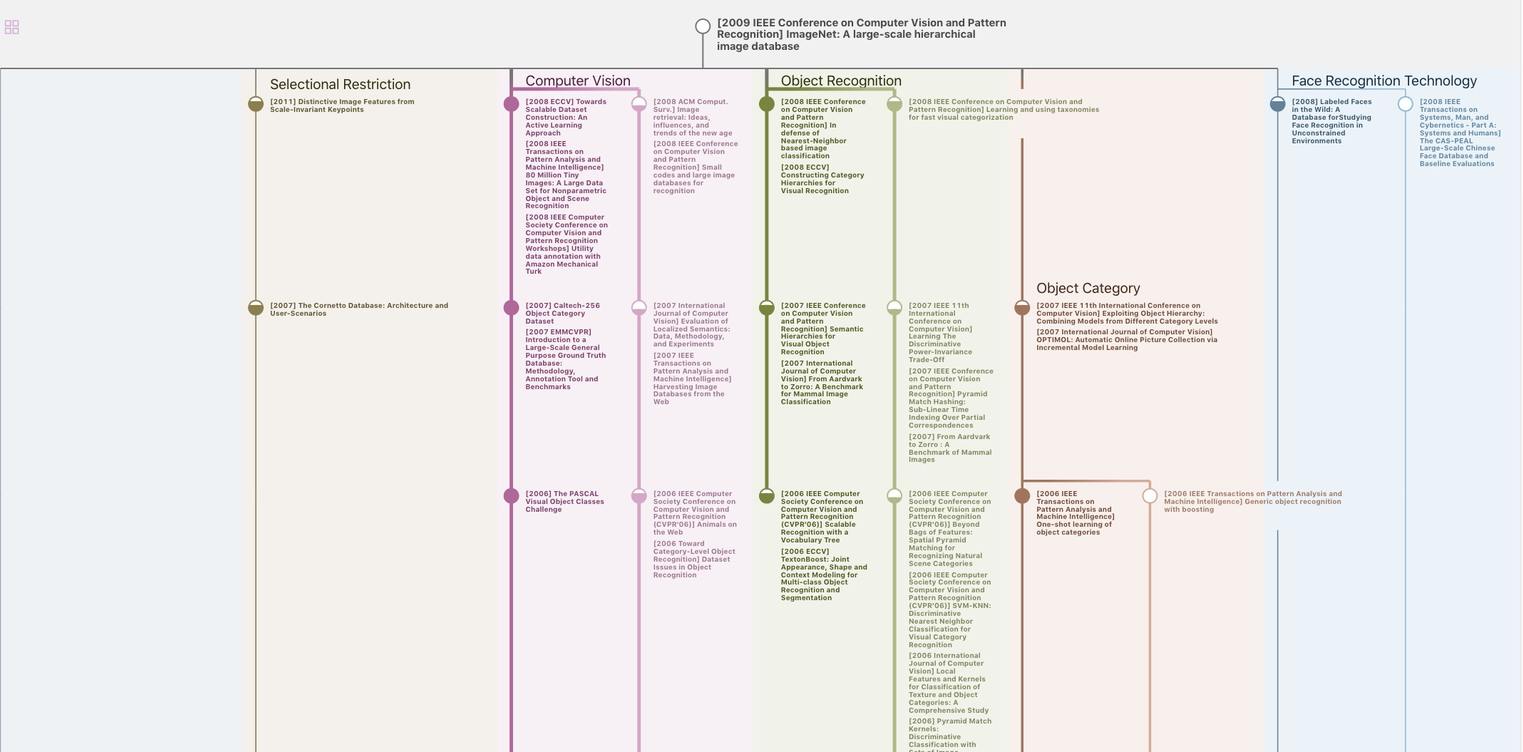
生成溯源树,研究论文发展脉络
Chat Paper
正在生成论文摘要