Sampled-Data Adaptive Iterative Learning Control for Uncertain Nonlinear Systems
IEEE Transactions on Systems, Man, and Cybernetics: Systems(2024)
摘要
In the realm of data-driven adaptive iterative learning control (AILC), the emphasis in designing and analyzing control schemes mainly concentrates on discrete-time systems, while fewer results are developed for the more common continuous-time plants. To overcome this limitation, a practical sampled-data AILC (SDAILC) is developed for continuous-time nonaffine nonlinear plants. A sampled-data iterative dynamic linearization (SDIDL) method is devised to build the dynamic connection between input and output (I/O) data throughout different iterations. On this basis, the SDAILC method, including a sampled-data parameter estimation algorithm and a learning control law, is proposed by utilizing optimization-based design. In SDAILC, the sampling period is treated as a parameter to compensate for its influence on the control performance, and an error feedback is naturally involved, improving the robustness against uncertainties and the closed-loop stability of the plant. Notably, SDAILC is a data-driven approach independent of model information. The validity of SDAILC is proved mathematically and demonstrated by simulations.
更多查看译文
关键词
Data-driven control,iterative learning control (ILC),nonaffine nonlinear system,sampled-data control
AI 理解论文
溯源树
样例
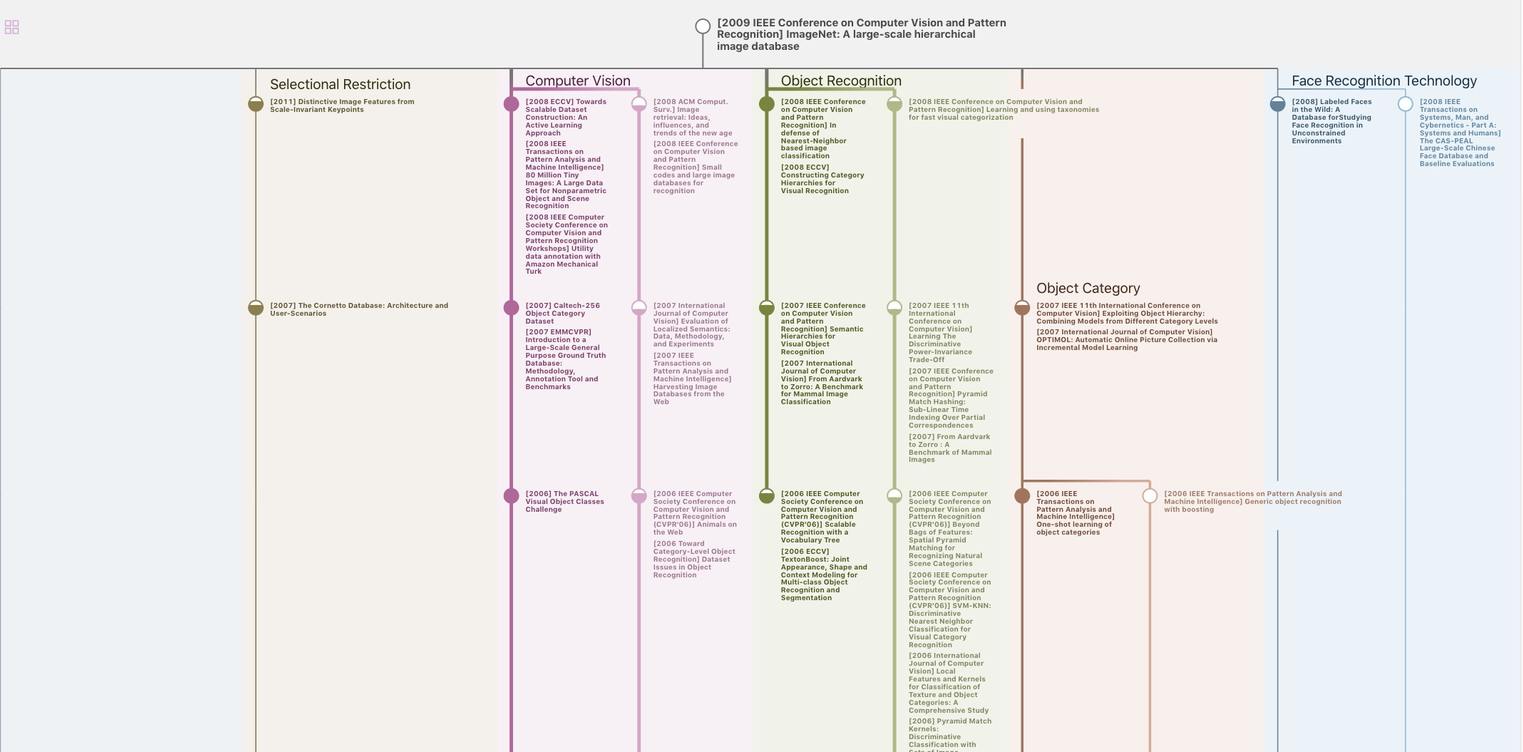
生成溯源树,研究论文发展脉络
Chat Paper
正在生成论文摘要