Reinforcement Learning for Efficient Power Systems Planning: A Review of Operational and Expansion Strategies
Energies(2024)
摘要
The efficient planning of electric power systems is essential to meet both the current and future energy demands. In this context, reinforcement learning (RL) has emerged as a promising tool for control problems modeled as Markov decision processes (MDPs). Recently, its application has been extended to the planning and operation of power systems. This study provides a systematic review of advances in the application of RL and deep reinforcement learning (DRL) in this field. The problems are classified into two main categories: Operation planning including optimal power flow (OPF), economic dispatch (ED), and unit commitment (UC) and expansion planning, focusing on transmission network expansion planning (TNEP) and distribution network expansion planning (DNEP). The theoretical foundations of RL and DRL are explored, followed by a detailed analysis of their implementation in each planning area. This includes the identification of learning algorithms, function approximators, action policies, agent types, performance metrics, reward functions, and pertinent case studies. Our review reveals that RL and DRL algorithms outperform conventional methods, especially in terms of efficiency in computational time. These results highlight the transformative potential of RL and DRL in addressing complex challenges within power systems.
更多查看译文
关键词
reinforcement learning,optimal power flow,economic dispatch,expansion planning
AI 理解论文
溯源树
样例
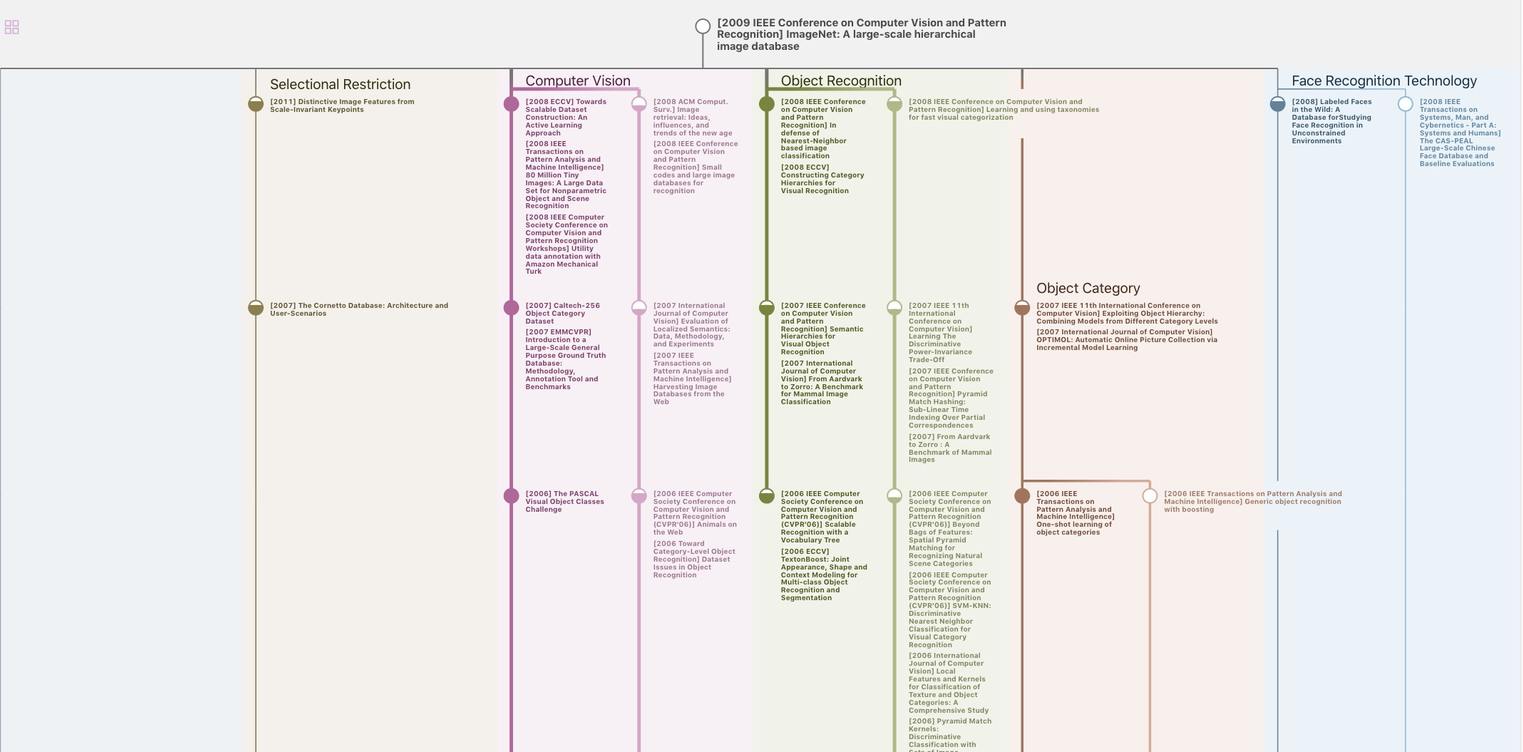
生成溯源树,研究论文发展脉络
Chat Paper
正在生成论文摘要