Enhancing Marketing Activity Prediction: A Framework for Early and Continuous Insights.
IEEE International Conferences on Internet of Things(2023)
摘要
Online marketing activities have become a pivotal driver of growth in various industries. Despite the consensus that early predictions facilitate timely strategic adjustments, many companies still only rely on human professionals due to the inadequacies of existing marketing activity prediction methods, resulting in uncertain outcomes and high labor costs. There is an urgent need for a methodology capable of learning patterns from extensive historical data and providing precise predictions. In this research, we propose a marketing activity prediction framework consisting of two components: static prediction and dynamic prediction, which can make predictions in the initial phases and throughout the entire course of an activity, respectively. We primarily face two challenges: (1) effectively incorporating sparse textual data into machine learning models, and (2) addressing the pronounced randomness in the time series of activities, particularly in predicting patterns not previously observed. To mitigate these problems, we introduce a text-value mapping approach and a method that enhances predictions by leveraging similarities between different activities and pre-trained models. We assess their performance in regression and temporal prediction tasks, with experimental findings validating the effectiveness of our framework in the realm of marketing activity prediction.
更多查看译文
关键词
Marketing activity,Text utilization,Correlation of different time series,Temporal prediction
AI 理解论文
溯源树
样例
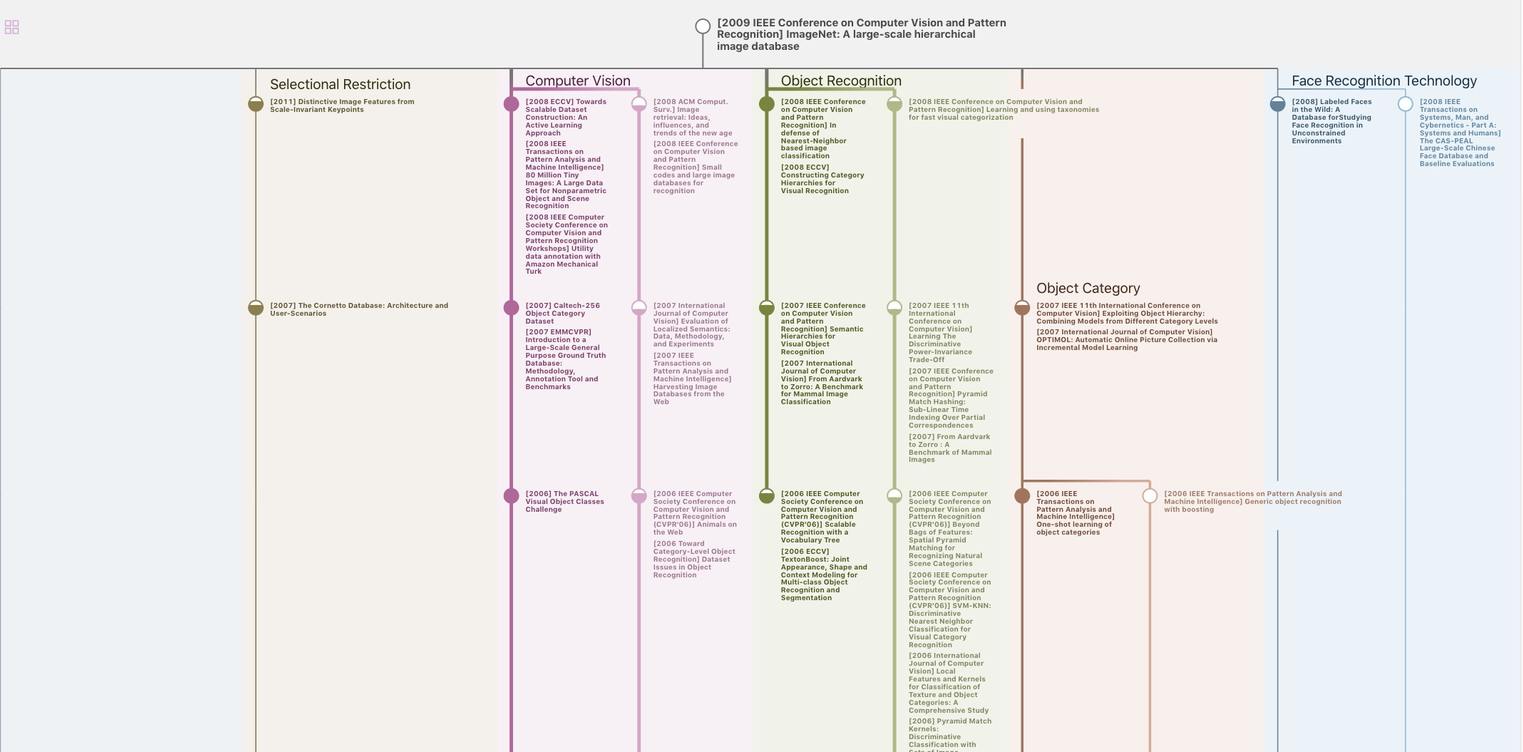
生成溯源树,研究论文发展脉络
Chat Paper
正在生成论文摘要