Application of machine learning to forecast agricultural drought impacts for large scale sub-seasonal drought monitoring in Brazil
crossref(2024)
摘要
Abstract. Drought events have increased in frequency and severity in recent years, and result in significant economic losses. Although the Brazilian semi-arid northeast has been historically associated with the impacts of drought, drought is of national concern, from 2011–2019, drought events were recorded in all Brazilian territories. Droughts can have major consequences for agricultural production, which is of particular concern given the importance of soybeans for socio-economic development. Due to its regional heterogeneity, it is important to develop accurate drought forecast and assessment tools for Brazil. We explore machine learning as a method to forecast the vegetation health index (VHI), for large scale monthly drought monitoring across agricultural land in Brazil. Furthermore, we also determine spatio-temporal drivers of VHI across the wide variation in climates, as well as evaluate machine learning performance for ENSO variation, forecasting of the onset of drought impact, and how the trade off between spatial variation and sample size affects model performance. We show that machine learning methods such as gradient boosting methods are able to more easily forecast vegetation health in the north and north east Brazil than south Brazil, and perform better during La Niña events than El Niño events. Drought impacts which reduce VHI below the commonly used 40 % threshold can be forecast across Brazil with similar model performance. SPEI is shown to be a useful indicator of drought impact, with 3 month accumulation periods preferred over 1 and 2 months. Results aim to inform future developments in operational drought monitoring at the National Center for Monitoring and Early Warning of Natural Disasters in Brazil (CEMADEN). Future work should build upon methods discussed here to improve drought forecasts for agricultural drought response and adaptation.
更多查看译文
AI 理解论文
溯源树
样例
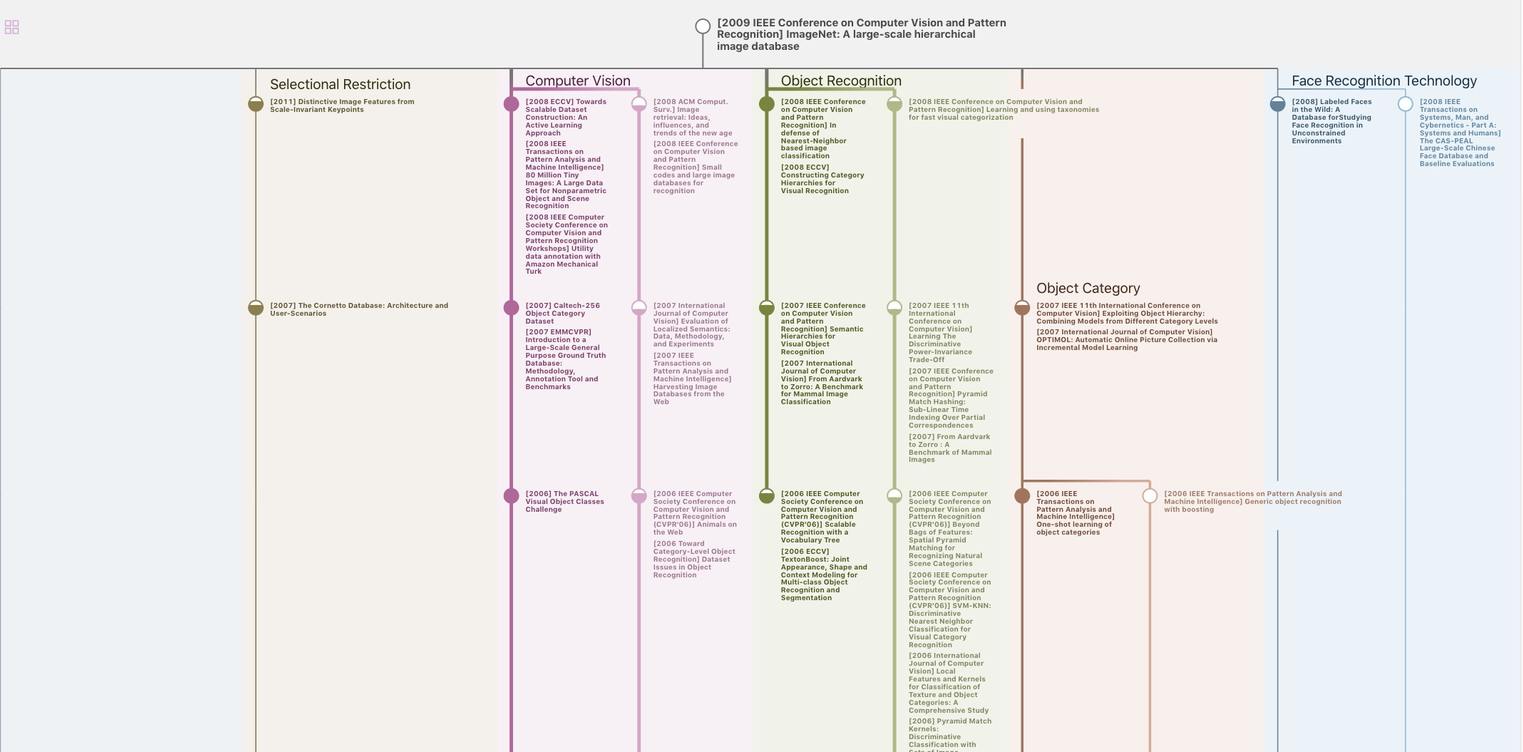
生成溯源树,研究论文发展脉络
Chat Paper
正在生成论文摘要